Biomarkers in drug discovery and development
Posted: 1 November 2010 |
Robust and validated biomarkers are needed to improve diagnosis, monitor drug activity and therapeutic response and guide the development of safer and targeted therapies for various chronic diseases. While different types of biomarkers have been impactful in the field of drug discovery and development, the process of identifying and validating disease specific biomarkers has been quite challenging. Recent advances in multiple ‘omics’ (multi-omics) approaches (e.g., genomics, transcriptomics, proteomics, metabolomics, cytometry and imaging) in combination with bioinformatics and biostatistics have made it possible to accelerate the discovery and development of specific biomarkers for complex chronic diseases. Although many challenges still need to be addressed, current efforts for the discovery and development of disease-related biomarkers will assist in optimal decision-making throughout the course of drug development and improve our understanding of the disease processes. Furthermore, effective translation of the preclinical biomarkers into the clinic will pave the way towards effective execution of personalised therapies across complex disease areas for the benefit of patients, healthcare providers and the bio-pharmaceutical industry.
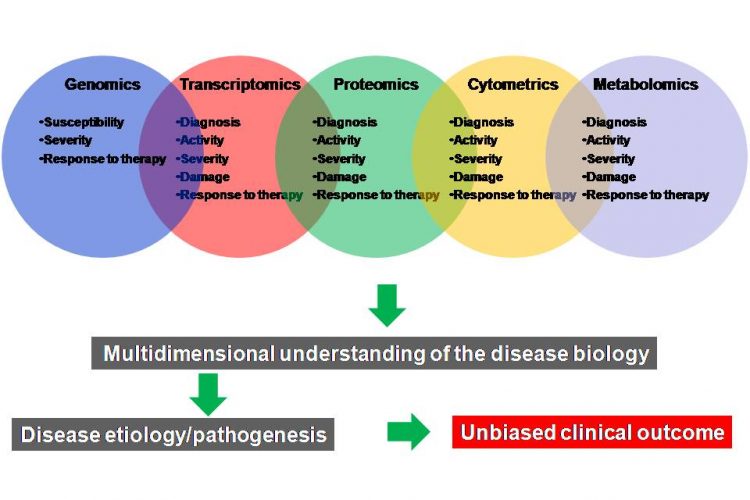
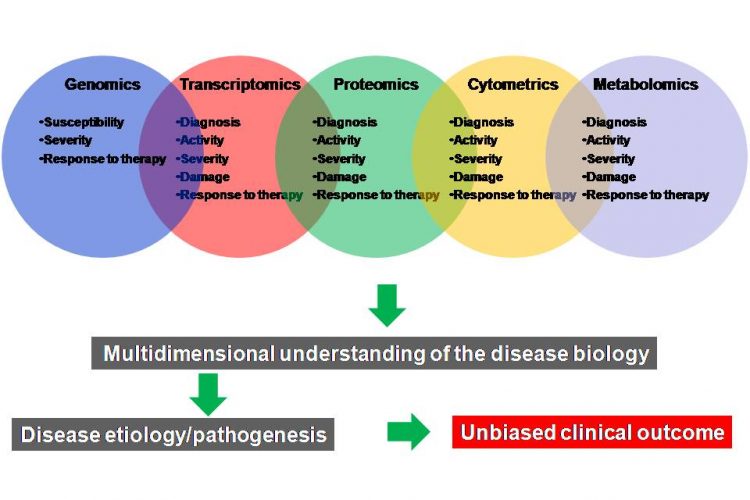
Figure 3 A multi-omics approach for the discovery and validation of biomarkers to probe multidimensional phases of disease biology. A robust biomarker discovery, development and validation effort must bring together multiple ‘omics’ technologies, data types, databases and bioinformatics and biostatics to identify the most predictive biomarkers across DNA, RNA, protein, phenotype and metabolite domains
Robust and validated biomarkers are needed to improve diagnosis, monitor drug activity and therapeutic response and guide the development of safer and targeted therapies for various chronic diseases. While different types of biomarkers have been impactful in the field of drug discovery and development, the process of identifying and validating disease specific biomarkers has been quite challenging. Recent advances in multiple ‘omics’ (multi-omics) approaches (e.g., genomics, transcriptomics, proteomics, metabolomics, cytometry and imaging) in combination with bioinformatics and biostatistics have made it possible to accelerate the discovery and development of specific biomarkers for complex chronic diseases. Although many challenges still need to be addressed, current efforts for the discovery and development of disease-related biomarkers will assist in optimal decision-making throughout the course of drug development and improve our understanding of the disease processes. Furthermore, effective translation of the preclinical biomarkers into the clinic will pave the way towards effective execution of personalised therapies across complex disease areas for the benefit of patients, healthcare providers and the bio-pharmaceutical industry.
Advances in biomedical research over the years have raised expectations that safer and more effective therapies will be available. However, the drug industry’s ability to effectively translate biomedical research into marketed new therapies has met serious limitations2, with a discouragingly high attrition rate in clinical trials of greater than 90 per cent3. In another words, the chances for success of candidate drugs or biologics entering the phase I trial is estimated at approximately eight per cent3,4.
The low probability of success from first-in-human (FIH) to new drug application (NDA) may be explained by one or more reasons including pharmacogenetic heterogeneity of individuals to treatments and drug response3, lack of robust biomarkers, lack of safety and efficacy of drugs, and narrow therapeutic window. The goal of any drug discovery activity is to achieve maximal efficacy with minimal effects with a broader therapeutic window, and when side effects are inevitable, an early detection during clinical trials is essential. Additionally, the goal is to develop precision medicine and stratify patients by disease type or response to treatment for targeted therapies.
For the successful treatment of complex chronic diseases, including autoimmune, inflammatory, metabolic diseases and cancer, as well as to develop precision medicine and targeted therapies, it is essential to monitor the molecular basis of disease initiation and progression before, during and after the therapeutic intervention5. Therefore, a better understanding of the factors affecting response to drugs has resulted in an increased demand for additional efficacy, specificity and safety testing of drugs during new drug development. There is also public pressure for drugs to be more extensively tested for safety before approval6.
Despite this new drug development work path, which has increased time and cost, it is interesting to examine the development of new therapeutics for the treatment of AIDS as a case study for the development of surrogate end point biomarkers for other diseases. The average drug for AIDS was developed in three years, because the FDA has allowed the use of ‘viral load’ as a surrogate biomarker as a measure of probable clinical benefit with later confirmation of a mortality benefit in phase IV studies following registration7. The experience with AIDS drug development demonstrates that innovations can accelerate the development of drugs without compromising safety2. Therefore, validated and clinically relevant surrogate end point biomarkers8 can improve diagnosis, precision medicine, targeted therapy and monitor activity and therapeutic response.
Biomarkers are valuable tools not only for fundamental and applied research and for clinical applications, but also for patient stratification since they can be used to predict molecular and phenotypical alterations and to assess the efficacy and safety of therapeutic intervention across pharmacogenetically heterogeneous individuals.
Because of inter-individual pharmaco – genetic variation in drug response, the search for robust biomarkers for human diseases such as cancer, inflammation and neurodegenerative diseases is urgently needed. Many research groups have demonstrated that genetic polymorphisms influence the sensitivity of drug targets9, drug metabolism10, drug distribution11 and the risk of adverse effects12.
Both in vitro diagnostics and imaging techniques have been used to reveal additional information about disease subsets. For example, in cancer, gene expression profiling is already being used to supplement histologic and clinical assessments of tumours leading to evaluation of the likelihood of recurrence and the need for adjuvant therapy. For example, the target may be polymorphic (e.g., B2 adrenergic receptor), have variable expression (e.g. Her-2/neu, EGFR, and genes belonging to the PI3K pathway13) or be subject to specific mutations that affect expression or function or a particular protein/enzyme (e.g., K-ras, P53 and EGFR) as in cancer14,15. This strategy was successfully used with the drugs trastuzumab (Herceptin®) and imatinib (Gleevec®) to stratify patients6 based on their pharmacogenetic polymorphisms.
Pharmacogenetic heterogeneity in diseases other than cancer also exists, enabling the prediction of the risk or the severity of diseases in individuals (e.g., the increased risk of ankylosing spondylitis in carriers of the HLA-B27 allele16, and the association between the ‘shared epitope’ and rheumatoid arthritis (RA)17. Therefore, individual pharmacogenetic information can improve drug development by reducing variability and increasing the size of the treatment effect by stratifying patients by disease type or response to treatment.
Despite the significant efforts in the development of validated biomarkers to expedite clinical trials, reduce development costs, develop precision medicine and targeted therapies and stratify patients, molecular, imaging and psychometric approaches used in preclinical and clinical studies have resulted in limited success. The contribution of biomarkers to clinical diagnosis has also been poor due, in part, to lack of a coherent workflow connecting clinical end point biomarker discovery efforts with well-established methods for validation18. Additionally, insufficient understanding of immune and tissue specific modulation of target in clinical samples as well as a complete understanding of target modulation in preclinical in vivo models of disease has led to poor outcomes in clinical trials. This is due, in part, to the small size of the patient samples and the resulting limited statistical power of clinical studies. To overcome these limitations, a systematic workflow process involving candidate biomarker discovery, verification, assay validation, standardisation, qualification and finally application as a companion diagnostic should be followed. Moreover, a better coordination between experimental, bioinformatics and clinical strategies and involvement of different stakeholders in the biomarker discovery process is essential. This will require a significant collaborative effort across academic, bio-pharmaceutical and regulatory authorities.
Here, I discuss the definition and different criteria and strategies for biomarker discovery and development using multiple molecular approaches.
Biomarkers
A biomarker is a dynamic and informational biological substance (i.e., cellular, biochemical, molecular, genetic, protein, metabolite, specific post-translational modification or physiological or physical sign) that can be objectively measured and evaluated as an indicator of normal biological process, pathogenic processes and its progression or the pharmacologic responses to a therapeutic intervention19,20. Generally, biomarkers are categorised as molecular, imaging (PET, MRI and CT) and psychometric biomarkers (e.g., loss of memory to predict Alzheimer’s disease (AD)).
Many different biomarkers (Figure 1) can be discovered and developed in various stages of drug development (Figure 2, page 22). FDA pharmacogenomics guidance further defines possible, probable and known valid biomarker categories depending on available scientific information on the marker. For example, clinical end point biomarkers19 are defined as those with a characteristic or variable that reflects how a patient feels, functions or survives, whereas surrogate end point biomarkers21 are intended to substitute for a clinical end point (e.g., regulatory decisions). Therefore, designation of ‘surrogate end point’ requires registration with regulatory authorities. Relatively few biomarkers are surrogate endpoints (e.g., FDA has permitted the use of HIV ‘viral’ load as surrogacy for AIDS7. A surrogate end point is expected to predict clinical outcome (benefit or harm, or lack of benefit) based on epidemiologic, therapeutic, pathophysiologic or other scientific evidence. Although earlier discussions on the use of biomarkers in drug development has emphasised the use of biomarkers as surrogate end points for effectiveness, most applications of new biomarkers may not involve surrogacy (e.g., prediction of drug safety)6.
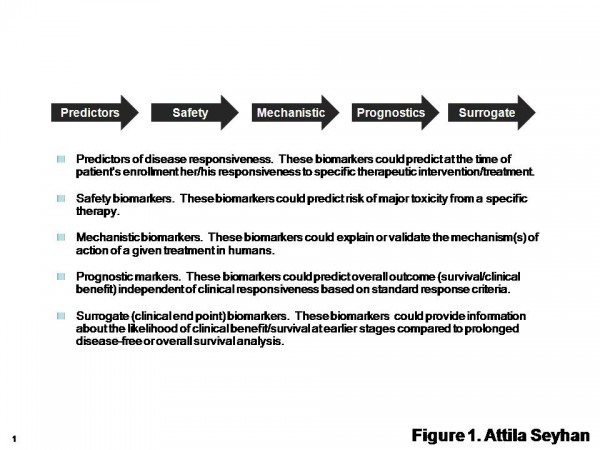
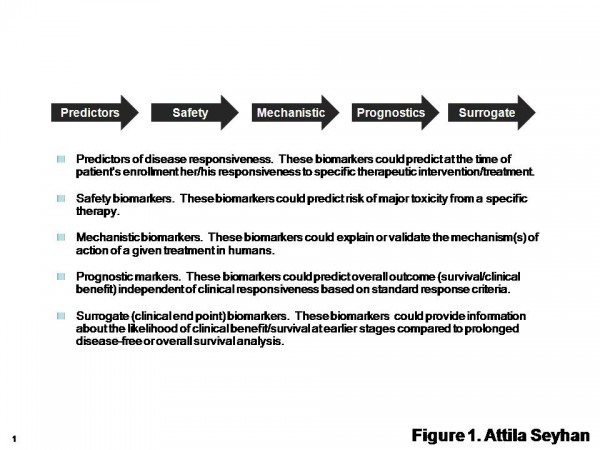
Figure 1 Types of Biomarkers. The International Society for the Biological Therapy of Cancer (iSBTc) in collaboration with the United States Food and Drug Administration (FDA) has developed standardised definitions of biomarkers. Adapted from Butterfield et al1
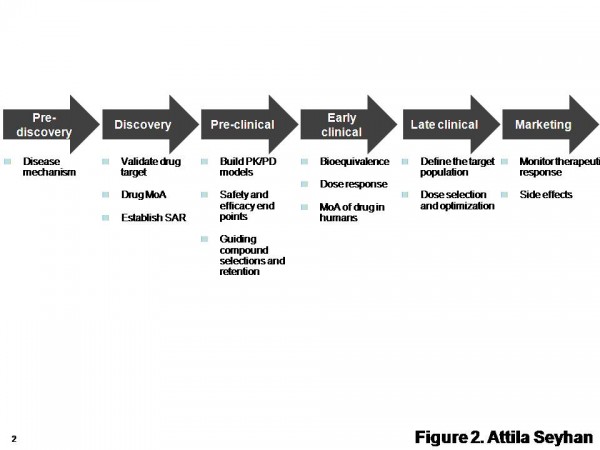
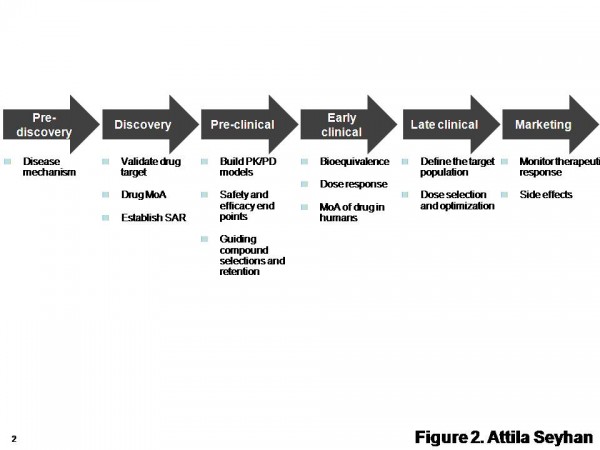
Figure 2 Diverse biomarkers can be discovered and developed in various stages from drug discovery to safety. SAR: structure-activity relationship. MoA: mechanism of action. Adapted from http://www.pharmaasia.com/article-7526-supportingbiomarkerdevelopmentwithit-Asia.html
Types of biomarkers
As summarised in Figure 1 and discussed in Table 1, several biomarkers have been developed to assist in diagnosis and prognosis of specific diseases, disease progress and patient response to treatments, assist in identification and validation of novel targets, provide insights in drug mechanism of action, help to characterise disease sub populations, to establish treatments for the patients and predict treatment response (personalised medicine)1.
Biomarkers can be classified based on their area of applications such as predictor, diagnostic, prognostic, mechanistic, pharmacodynamic, safety and surrogate end point biomarkers (Table 1).
Types of Biomarkers | Description | Examples |
Predictor | These are disease-associated biomarkers that can indicate whether there is a threat of disease and measure patient’s responsiveness to treatment. | VEGF and fibronectin can predict clinical response to interleukin-2 (IL-2) therapy of patients with metastatic melanoma and renal cell carcinoma and high levels of these proteins correlate with lack of clinical response to IL-2 therapy and decreased overall survival of patients 39. Serum VEGF and fibronectin are easily measured predictor biomarkers; therefore, could serve to exclude patients unlikely to respond to IL-2 therapy. Carbonic anhydrase IX-G250MN (CAIX) expression can predict responses to IL-2 in renal cell carcinoma and high CAIX staining of primary renal cell carcinoma tumors correlate with a significantly better survival rate after IL-2 therapy 40. |
Diagnostic | These are the biomarkers used for diagnosis of an existing disease and staging of disease biomarkers 15. Diagnostic biomarkers can also be used to stratify patients by disease type and response to treatment. Biomarkers that can reveal the status of the target (e.g., at the level of receptor expression, genetic polymorphism, gene expression, somatic mutation, etc) in individual patients may provide significant predictive value for treatment response. | Cardiac troponin for the diagnosis of myocardial infarction and staging of disease biomarkers such as, brain natriuretic peptide for congestive heart failure and measurements of carcinoembryonic antigen-125 for various cancers 19. Autoantibodies such as anti-cylic citrullinated peptide (CCP) are detected in patients with RA 41. Antinuclear antibodies and anti-Ro (SSA) antibodies are detected in patients with Sjogren’s syndrome and other systemic autoimmune diseases 42. In cancer, gene expression profiling was successfully used in tumors to evaluate the likelihood of recurrence (e.g., overexpression of Her-2/neu) and the need for adjuvant therapy leading to the successful use of trastuzumab and imatinib in responder only patient populations 6. |
Prognostic | These biomarkers predict the future outcome of a disease in an individual and can predict overall survival rate and clinical benefit from a therapeutic intervention. These biomarkers are often used to establish inclusion criteria for a clinical trial or for defining a patient population. | The immunological data (the type, density, and location of immune cells within the tumor samples) is a better predictor of patient survival than the histopathological methods currently used to stage colorectal cancer 43. The presence of intratumoral T cells correlates with improved progression-free survival and overall survival among patients with advanced ovarian carcinoma 44. Overexpression of Her-2/neu in breast cancer or EGFR expression in colorectal cancer indicates poor prognoses. |
Mechanistic | These biomarkers may inform and validate the mechanism(s) of action of a particular treatment | Sequential gene profiling of basal cell carcinomas treated with imiquimod (a Toll-like receptor-7 agonist capable of inducing complete clearance of basal cell carcinoma (BCC) and other cutaneous malignancies) in a placebo-controlled study has revealed the early transcriptional events induced by imiquimod and provided insights about immunological events preceding acute tissue and/or tumor rejection 45. |
Pharmacodynamic | These biomarkers are signatures of a certain pharmacological response to an active compound or biomarkers for monitoring the clinical response to an active compound or intervention. Linking biomarkers and mechanistic PK/PD models are critical to understand the relationship between dose and biomarker response. Preclinical PK-PD models have to be validated by predicting dose-efficacy relationship in clinical trials, knowing the extent and duration of target exposure and modulation is necessary to achieve effects during clinical trials. This is often achieved by preclinical PK/PD modeling by measuring plasma exposure, target occupancy and PD endpoints for target activity (e.g., the level of P-kinase or P-substrate for kinase targets) over time and at various doses 46. To show target engagement; biomarkers need to be very close to target (e.g., examining proximal P-kinase or P-substrate levels for kinase targets should reveal target engagement by the drug). | Hemoglobin A1C for antidiabetic treatment, which of special interest in dose optimization studies and is used in decision making in early drug development 47. DCEMRI (dynamic contrast-enhanced magnetic resonance imaging) for the detection of changes in the permeability of tumor vasculature, FDG PET (fluorodeoxyglucose positron emission tomography) for the detection of changes in Glucose metabolism and FLT PET (fluoro-L-thymidine positron emission tomography) for the detection of changes in DNA synthesis 48. |
Surrogate end point | These may yield information on the clinical benefit/survival at earlier stages as opposed to prolonged disease-free or overall survival analysis 1. If validated, a surrogate biomarker may serve as a primary end point in a registration study 49. | For example, if a treatment affects the biomarker, the biomarker will serve as a surrogate end point for evaluating clinical benefit (e.g., Hemoglobin A1C) 8. Response rate or progression free survival in oncology or bone mineral density in osteoporosis prevention and treatment. Another example is the HIV viral load as a measure of probable clinical benefit with later confirmation of a mortality benefit 7. |
Safety
| These biomarkers can predict at the time of patient’s enrollment her/his likelihood to suffer major toxicity from a specific therapy 1. Safety biomarkers measure drug induced organ (e.g.,, kidney, liver, vascular system, carcinogenesis, and myopathy) injuries. | Albumin, total protein, B2-microglobulin, cystatin C, Kim-1, and TFF3 have been selected from earlier studies as biomarkers of drug-induced kidney injuries 50. |
However, irrespective of their definition and classification, the development and use of biomarkers should be dictated by the principle of being associated to how they will be used; i.e., ‘fit-for-purpose’22. Since biomarkers can provide valuable information during the drug development and decision-making process, the biomarker strategy must be integrated into various phases of drug development from early discovery to late clinical drug development. Especially in the preclinical studies, linking biomarkers and mechanistic pharmacokinetic and pharmacodynamic (PK/PD) models can reveal the underlying mechanism of drug action, the relationship between dose and biomarker response, impact dose escalation studies on the time course of response, measure duration of response and lag between plasma drug concentration and response, measure effect of repeated doses (toleration, sensitisation, reflex mechanisms), range of doses, dose separation, and dosing interval and form the basis for clinical trial simulations. Biomarkers can be used in dose selection for phase II/III trials based on biomarker PK/PD and projected therapeutic index since optimal dose selection may be the most important deliverable from early clinical development. In addition, biomarkers can help to differentiate a candidate drug from other compounds based on demonstration of a key response (safety, efficacy).
Since the main objective is not to measure biomarker response but to make informed decisions, these all contribute to and increase confidence in biomarkers, leading to go/no-go decisions and help with designing future studies.
To have any value, biomarkers must be validated by several molecular methods so that any time they are identified, they would be pathognomonic (characteristic) of a particular biological state or disease15. After validation, a biomarker can be used to diagnose disease risk, presence, prognosis or to establish treatments for the patients.
Technologies used for biomarker discovery
The information on biomarkers comes in the form of human genes, genetic variation, measurements of RNA, proteins and metabolites. Therefore, many approaches, including genomics, proteomics and metabolomics as well as imaging techniques hold promise for generating new biomarkers that can reflect the state of health or disease at the molecular level14. Different biomarkers (Figure 2) can be quantitatively measured in biological samples (e.g., plasma, serum, cerebrospinal fluid, bronchoalveolar lavage, tissue biopsies, whole blood, urine, saliva, etc) and include genomic biomarkers such as gene mutations or polymorphisms, transcriptomic biomarkers such as gene or microRNA (miRNA) expression profiling, allele/haplotype mapping, epigenomics, SNP analysis, pharmacogenomics, and non-genomics, proteomics, metabolomics, glycomics, and other small molecules in samples. Researchers can now perform fullgenome, deep and transcriptome-sequencing of mRNA or miRNAs and determine gene copy-numbers23 and mass spectrometry-based analysis, which allows detection and measurement of selected compounds, proteins and other biomolecules24. Advances in high throughput and multiplexed processes, microarray and microfluidics25 technologies, and global-scale genomic and proteomic approaches have made it possible to characterise global changes associated with disease conditions and therapeutic intervention and, in the process, identification of novel biomarkers and associated pathways. For example, novel analytical technologies have enabled global-scale and highly parallel surveys of genetic polymorphisms, gene expression, and protein expression in clinical samples26. This has led to profiling populations of cells in the immune system using various methodologies such as multiparameter flow cytometry which allow functional evaluation of living cell populations and multiplex analysis of intraand extra-cellular mediators, targeted or genome-wide profiles of gene expression27, profiles of miRNA expression28,29, histone modification28,30 and protein analysis.
The utility of large-scale analysis of many proteins and mRNAs using multiplexed protein arrays and cDNA microarrays have been described for studying autoimmune disease31. In another study, researchers32 compared the protein expression profile of the synovial tissue samples of patients with RA with that of patients with osteoarthritis identifying disease specific signatures.
New biomarker types (Figure 1) and methodologies for their discovery are constantly emerging and existing technologies are evolving (Figure 3). Therefore, more critical to this process is to identify robust and clinically relevant multiple (6 – 10) end point biomarkers using a combination of multi-omics approaches (Figure 3) and validation of these biomarkers in clinically relevant human populations to see whether the distribution of biomarkers is Gaussian, and whether significant differences in values exist among different age, sex or race.
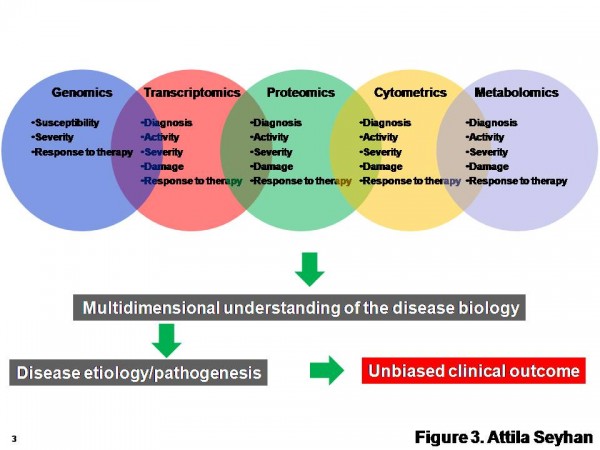
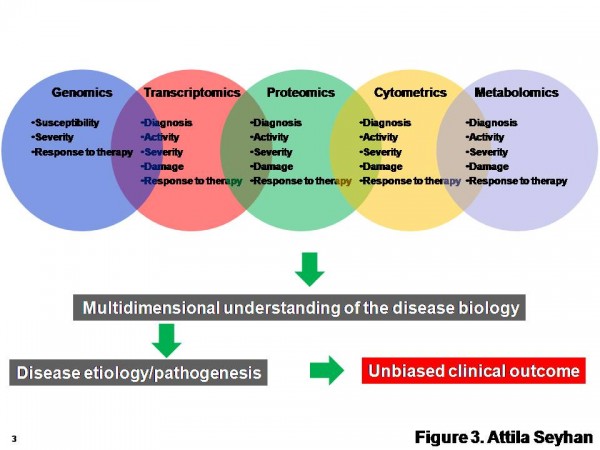
Figure 3 A multi-omics approach for the discovery and validation of biomarkers to probe multidimensional phases of disease biology. A robust biomarker discovery, development and validation effort must bring together multiple ‘omics’ technologies, data types, databases and bioinformatics and biostatics to identify the most predictive biomarkers across DNA, RNA, protein, phenotype and metabolite domains
Use of biomarkers in preclinical and clinical studies
Use of biomarkers from pre-discovery to late clinical drug development (Figure 2) and decision making is critical to evaluate activity in animal models, link animal and human pharmacology via proof-of-mechanism or other observations, evaluate safety in animal models and assess human safety early in development. Additionally, every stage of drug development has its own specific set of biomarkers that may or may not be applicable to other stages.
To have any value, biomarkers must be robust, and be reproducible and be accessible (i.e., present in body fluids and measurable). A biomarker must also be sensitive and specific to distinguish true positives from false negatives33. They should indicate not only the disease presence but also the disease response to time and treatment. And most importantly, detection of a biomarker should be clinically relevant and provide clinical benefits to the patient (i.e., improved survival and / or quality of life).
Examples of biomarkers in preclinical studies are serum chemistries, cell surface protein expression, drug PK/PD measurements, drug metabolising isoenzyme phenotype, serum transaminases, genomic expression profile, drug distribution or receptor occupancy via imaging.
Use of biomarkers in late drug development are the evaluation of dose-response and optimal regimen for desired pharmacologic effect, safety markers to determine dose-response for toxicity, and determination of the role of differences in metabolism34. Once validated, for example, a biomarker can be used in dose selection for phase II/III clinical trials based on the biomarker’s PK/PD relationship and projected therapeutic index as well as to differentiate candidate compounds from other compounds. The biomarkers that measure PK/PD relationship also provide valuable feedback to discover whether the mechanism does or does not translate to clinical trials. Target specific biomarkers can also be used to stratify patients by disease type or response to treatment. This strategy was effectively used with the drugs trastuzumab (Herceptin®) and imatinib (Gleevec®) to stratify patients6 based on their pharmacogenetic polymorphisms.
Biomarkers in clinical studies have been used for diagnosis, a tool for staging disease, as indicators of disease status, and to predict and/or monitor clinical response to a therapeutic intervention (e.g., electrocardiogram, PET brain image, serum chemistries, auto-antigens in blood, bone densitometric measurement, pulmonary function test, neonatal Apgar score). Biomarkers used in late clinical development are psychometric testing, pain scales, imaging studies, culture status (antimicrobials), pulmonary function tests, serum chemistries and electrocardiogram. Moreover, biomarkers have the potential to reveal prognostic information about the future health status of a patient whereas diagnostics classify patients at one point in time.
Current status, challenges and future perspectives
Although DNA microarray studies have led to important advances in the study of global gene expression profiling of autoimmunity, their use is limited owing to the problem of physio – logically important splice variants that exist in individual cell populations31. An additional problem lies in the fact that a large proportion of mRNAs are not translated into proteins, despite being activated at the level of transcription.
Proteomics is limited largely by current technology and the scarcity of high-quality reagents such as monoclonal antibodies and informatics tools as well as difficulty to identify low-abundance markers18. Unbiased proteomic approaches have had limited success for studying systemic autoimmune diseases, while array and FACS platforms are limited because it is a bottom-up targeted approach with a priori knowledge of target proteins. It is impossible to know which important aspects will be missed owing to the limitations of current arrays or antibodies, etc31.
New approaches in terms of bioinformatics and assay platforms as well as integration of multi-omics platforms need to be developed to study disparate datasets simultaneously, including gene, miRNA and protein expression, and FACS platforms. The use of multi-omics platforms and multiplexed approaches in the analysis of systemic autoimmune diseases will not only identify numerous useful biomarkers but also reveal areas for further development and improvement. There is also increasing consensus that more than one biomarker is needed for making accurate efficacy or safetyrelated decisions. Because of this, early identification of these biomarkers is critical in go/no-go decisions and at critical stages of drug development. In this context, using multiplexed technology and multi-omics approach could offer a significant contribution to early clinical development. Therefore, understanding the advantages of these strategies and technologies as well as their limitations, and how they could be utilised for the discovery of specific biomarkers is critical for successful delivery of ‘fit for purpose’ biomarkers. Another problem in the field is the assay reproducibility and robustness. Increasing the sample size and number of replicates as well as incorporation of a team of bioinformatics scientists and biostatisticians into the study from clinical study design to data analysis should improve this process.
The limited or restricted access to disease associated tissues is another limitation in the field. This has led to the wide scale utilisation of peripheral blood for identification of DNA, RNA, protein or other small molecule biomarkers largely in immune diseases. For instance, global gene expression profiling is now used as surrogates for biological phenotypes of cell populations within the immune system35.
Although the global profiling of either gene or protein expression is instructive, evaluation of mRNA or protein expression alone may not be sufficient to identify clinically relevant surrogate end point biomarkers that confidently reflect disease phenotype before, during or after therapeutic intervention15. Most of the novel biomarkers and their correlations to disease largely derived from two or more samples at one point in time. The methodologies employed are essentially snap-shot events of the disease state in a specific time, whereas signalling networks are dynamic in both structure and their use23. Because single measurements rarely capture all dimensions of clinical outcomes, a multidimensional and continuous model is needed to replace the single dimension.
Information arising from population ‘mean’ data may not be applicable for a particular individual, because outcomes happen to individual rather than an entire cohort; therefore, individual responders rather than population mean analyses should be developed and correlated with specific biomarker status towards the development of a targeted therapy. Decreasing individual differences in drug exposure is therefore critical to reduce response variability6.
In addition to developing sensitive and robust biomarkers for various indications, coupling biomarkers to a simple, inexpensive point-of-care diagnostic test can help to stratify patient populations for a specific disease or treatment regimen and reduce the size of clinical trials. It has been stated that development of trastuzumab without incorporation of a diagnostic assay would have required a trial of 11,000 patients to achieve a statistically significant result14.
After verification of the clinical usefulness of a biomarker, in vitro diagnostics companies may commercialise it; however, medical, regulatory and legal issues will also affect this decision36.
The life-cycle for biomarker development from discovery to validation and submission to regulatory authorities for qualification is a concern and this can be expedited by engaging other stakeholders earlier in the process8. Towards this goal, significant effort has gone into the establishments of initiatives, such as ‘The Biomarker Consortium’ (http://www.biomarkersconsortium.org), whose members include government, private, industrial, academic and patient groups for the development of clinically translatable biomarkers6.
Finally, because biomarkers may have undesirable consequences for individuals (e.g., professional implications, stigmatisation, obtaining insurance and / or financial credits), their use may raise many social, ethical and legal questions37. A collaborative effort between scientists, clinicians, ethicists, legal scholars, policymakers and marketers should ameliorate these issues38.
Conclusion
Technical challenges and poor experimental design have resulted in limited success in the discovery and validation of robust biomarkers as surrogate end-points that can be substituted for clinical meaningful end-points. A better coordinated experimental and clinical design, more standardised multi-omics and multi-parametric technology and analysis platforms, as well as a collaborative effort between scientists, clinicians, biopharmaceutical industry and regulatory authorities should expedite the discovery of validated and qualified biomarkers to a much greater extent.
Acknowledgement
I would like to thank Monique Lehmann, Claudio Carini, Paresh Thakker, Divya Chaudhary, Cara Williams, David Beidler and Jaime Masferrer for critical reading of the article.
References
- Butterfield, L. H. et al., (2008) J Transl Med 6, 81
- Woosley, R. L. and Cossman, J., (2007) Clin Pharmacol Ther 81 (1), 129
- Lang, L., (2004) Gastroenterology 127 (4), 1026
- fda.gov, http://www.fda.gov/oc/speeches/ 2004/bascrty0707.html
- Shendure, J., Mitra, R. D., Varma, C., and Church, G. M., (2004) Nat Rev Genet 5 (5), 335
- Woodcock, J. and Woosley, R., (2008) Annu Rev Med 59, 1
- Hughes, M. D. et al., (1998) AIDS 12 (14), 1823
- Wagner, J. A., Williams, S. A., and Webster, C. J.,(2007) Clin Pharmacol Ther 81 (1), 104
- D’Andrea, G. et al., (2005) Blood 105 (2), 645
- Kirchheiner, J. and Brockmoller, J., (2005) Clin Pharmacol Ther 77 (1), 1
- Badagnani, I. et al., (2006) J Pharmacol Exp Ther 318 (2), 521
- Hung, S. I. et al., (2006) Pharmacogenet Genomics 16 (4), 297
- Buckland, D., (2010) The Bulletin Board 4 (3), 441
- Woodcock, J., (2007) Clin Pharmacol Ther 81 (2), 164
- Bayele, H. K. et al., (2010) Drug Discov Today 15 (3-4), 127
- Thomas, G. P. and Brown, M. A., (2010) Immunol Rev 233 (1), 162
- de Vries, R. R., Huizinga, T. W., and Toes, R. E., (2005) J Autoimmun 25 Suppl, 21
- Rifai, N., Gillette, M. A., and Carr, S. A., (2006) Nat Biotechnol 24 (8), 971
- Group, T. B. D. W., (Clin. Pharmacol. Therap., 2001), Vol. 69, pp. 89
- Alevizos, I. and Illei, G. G., (2010) Nat Rev Rheumatol 6 (7), 391
- Administration, U. S. F. a. D., edited by U. S. D. o. H. a. H. S. U.S. Food and Drug Administration (Rockville, MD, USA, March 2004)
- Lee, J. W. et al., (2006) Pharm Res 23 (2), 312
- Erler, J. T. and Linding, R., (2010) J Pathol 220 (2), 290
- Ong, S. E. and Mann, M., (2005) Nat Chem Biol 1 (5), 252
- Kotz, K. T. et al., (2010) Nat Med 16 (9), 1042
- Haining, W. N. and Wherry, E. J., (2010) Immunity 32 (2), 152
- Heng, T. S. and Painter, M. W., (2008) Nat Immunol 9 (10), 1091
- Hyatt, G. et al., (2006) Nat Immunol 7 (7), 686
- Schones, D. E. et al., (2008) Cell 132 (5), 887
- Wu, H. et al., (2007) PLoS One 2 (10), e1020
- Araki, Y. et al., (2009) Immunity 30 (6), 912
- Fathman, C. G., Soares, L., Chan, S. M., and Utz, P. J., (2005) Nature 435 (7042), 605
- Drynda, S. et al., (2004) Pathol Res Pract 200 (2), 165
- Gramolini, A. O., Peterman, S. M., and Kislinger, T., (2008) Clin Pharmacol Ther 83 (5), 758
- Rolan, P., (1997) Br J Clin Pharmacol 44 (3), 219
- Nevins, J. R. and Potti, A., (2007) Nat Rev Genet 8 (8), 601
- Vitzthum, F., Behrens, F., Anderson, N. L., and Shaw, J. H., (2005) J Proteome Res 4 (4), 1086
- Caux, C., Roy, D. J., Guilbert, L., and Viau, C., (2007) Soc Sci Med 65 (2), 344
- Singh, I. and Rose, N., (2009) Nature 460 (7252), 202
- Dumez, B., Van Damme, K., and Casteleyn, L., (2007) Int J Hyg Environ Health 210 (3-4), 263
- Sabatino, M. et al., (2009) J Clin Oncol 27 (16), 2645
- Atkins, M. et al., (2005) Clin Cancer Res 11 (10), 3714
- Nishimura, K. et al., (2007) Ann Intern Med 146 (11), 797
- Schulte-Pelkum, J., Fritzler, M., and Mahler, M., (2009) Autoimmun Rev 8 (7), 632
- Galon, J. et al., (2006) Science 313 (5795), 1960
- Zhang, L. et al., (2003) N Engl J Med 348 (3), 203
- Panelli, M. C. et al., (2007) Genome Biol 8 (1), R8
- Winkler, H., (2010) European Pharmaceutical Review (Volume 13, Issue 3)
- Eck, S. L. and Paul, S. M., (2009) Clin Pharmacol Ther 87 (1), 21
- Wagner, J. A. et al., (2009) Clin Pharmacol Ther 86 (6), 619
- Holmgren, E., (2008) Stat Med 27 (24), 4928
- Zwierzina, H., (2008) Ann Oncol 19 Suppl 5, v33
- Sistare, F. D. et al., (2010) Nat Biotechnol 28 (5), 446
About the Author
Attila A. Seyhan
After receiving his PhD in Microbial Biochemistry and Biotechnology from Michigan Technological University, Houghton, MI on a NATO (North Atlantic Treaty Organisation) Science Fellowship, Dr. Attila Seyhan moved to Paris, France, for his postdoctoral studies in Professor Bernard Grandchamp’s lab at Xavier Bichat Hospital, University VII. There he studied regulation of ferritin gene during erythroid differentiation and cell proliferation with Dr. Carole Beaumont on a postdoctoral fellowship from the National Institute of Health and Medical Research of France (INSERM). He did his second postdoctoral fellowship with Professor John Burke at the University of Vermont, studying hairpin ribozyme biochemistry and their use as antigene and antiviral agents. He later joined SomaGenics, Inc. to develop self-ligating hairpin ribozymes (RNA LassoTM) for in vitro and in vivo target modulation and as diagnostics and then on RNAi and methods for generating RNAi libraries and their application as antigene and antiviral (HIV, hepatitis C virus, and Semliki Forest virus) therapeutics. He then moved to Dharmacon, Inc. and OpenBiosystems (both part of Thermo Scientific) to develop lentiviral miRNA-based siRNA libraries, lentiviral-shRNAmir libraries, and lentiviral human and mouse miRNA mimic libraries. Thereafter he joined Wyeth Pharmaceutical to lead the RNAi and compound library screening team for target identification and validation in various diseases. He is now a senior leader in Translational Immunology of Inflammation and Immunology at Pfizer Biotherapeutics, leading the genomics biomarker discovery and development team.
email: [email protected]