How can AI be best harnessed for pharmacovigilance data?
Posted: 16 June 2023 | Catherine Eckford (European Pharmaceutical Review) | No comments yet
A GSK-funded paper on pharmacovigilance stated that the pharma industry needs to harness the properties of data to allow optimal use of AI, otherwise “we will fail to do all we can for patient safety”.
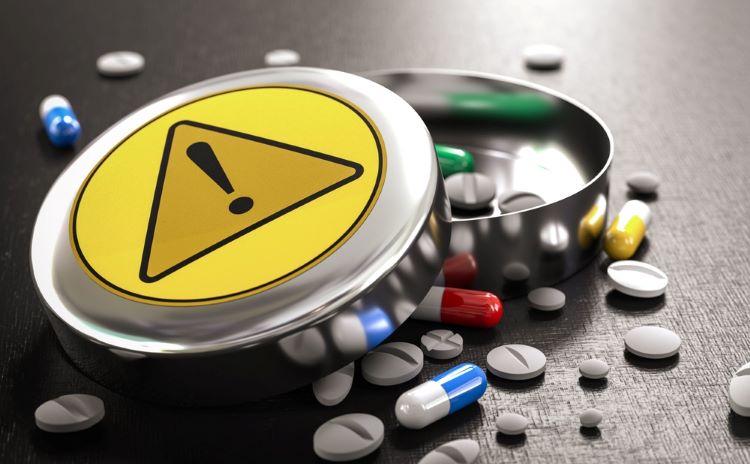
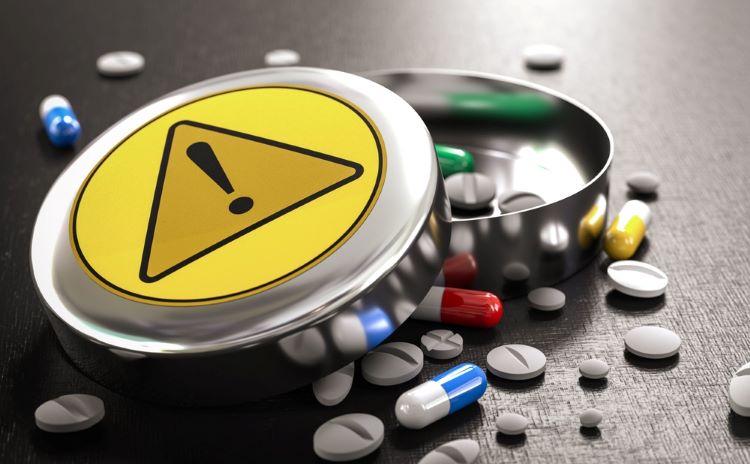
According to a recent review funded by GSK Biologicals, artificial intelligence (AI) and machine learning (ML) seems intuitively, to be well suited to perform pharmacovigilance (PV) tasks given the large volume of data, high degree of uncertainty and need to learn from data.
However, the paper highlighted the challenges these technologies hold for analytics.
The authors argued that fundamentally, implementation of AI/ML for pharmacovigilance has been hindered by the associated legal framework processes and governance issues. Bate et al. posed the question of how to best to harness these technologies to build a future fit for purpose.
Pharmacovigilance and AI
However the paper brought up one potential issue on heterogeneity: pharmacovigilance data are derived from a multitude of medicines, including vaccines, and, as a result, describing very different types of adverse drug reactions (ADRs) and adverse effects of vaccines, that can manifest differently, and have different, often unknown, mechanisms, and the data are captured and shared imperfectly.
AI/ML has the potential to impact the entire pharmacovigilance lifecycle”
Yet AI/ML has the potential to impact the entire pharmacovigilance lifecycle. AI/ML has shown potential in data ingestion (case intake or processing), such as duplicate detection and anomaly identification as an orthogonal approach to quality assurance, the paper noted.
The paper described a review that compared AI with traditional pharmacovigilance methods for a range of applications that primarily involved prediction. AI reportedly outperformed traditional methods in half of the studies evaluated.
Pharmacovigilance and machine learning
Applications of ML include analysing social media posts for safety insights, extracting information from clinical narratives in electronic health records (EHRs) and integrating data sources with multiple analytical approaches to provide richer insights.
The paper divided the use of machine learning for pharmacovigilance into three areas: data ingestion-related tasks, signal detection activities and, thirdly, other applications.
However, the overall performance characteristics of more sophisticated algorithms are not significantly better than simpler methods for signal detection and lack the greater transparency and thus usability of simpler methods, according to Bate et al.
Taking advantage of AI and ML
Research highlighted by the authors indicated that only about 10 percent of studies of AI/ML in pharmacovigilance published between 2000 and 2021 conformed to current best practices in ML. Therefore, this suggests sub-optimal use of ML in pharmacovigilance.
The pharmacovigilance community needs a system where both inputs and outputs are seamlessly connected across different data systems”
The pharmacovigilance community needs a system where both inputs and outputs are seamlessly connected across different data systems, the authors proposed. “Such a system could ultimately inform an interactive continual learning solution that promotes understanding of the benefit–risk of medicines and vaccines and allows prescribers, patients and other stakeholders to obtain information and pose questions as needed”.
Bate et al. concluded: “If we do not harness the properties of data to allow us to leverage AI optimally, we will fail to do all we can for patient safety.”
Related topics
Artificial Intelligence, Big Data, Big Pharma, Biologics, Biopharmaceuticals, Clinical Development, Clinical Trials, Data Analysis, Data integrity, Drug Development, Drug Safety, Informatics, Regulation & Legislation, Research & Development (R&D), Technology, Therapeutics