RNAi screens for the identification and validation of novel targets: Current status and challenges
Posted: 16 December 2010 |
Recent advances in RNA interference (RNAi) technology and availability of RNAi libraries in various formats and genome coverage have impacted the direction and speed of drug target discovery and validation efforts. After the introduction of RNAi inducing reaagent libraries in various formats, systematic functional genome screens have been performed to query the functions of individual genes, pathways or an entire genome in many disease areas, including cancer, viral pathogenesis and others. As a consequence of these screens, novel mediators of cellular response to disease pathogenesis or treatment approaches have been identified leading to the discovery of novel drug targets, development of combinatorial treatment approaches and patient selection biomarkers.
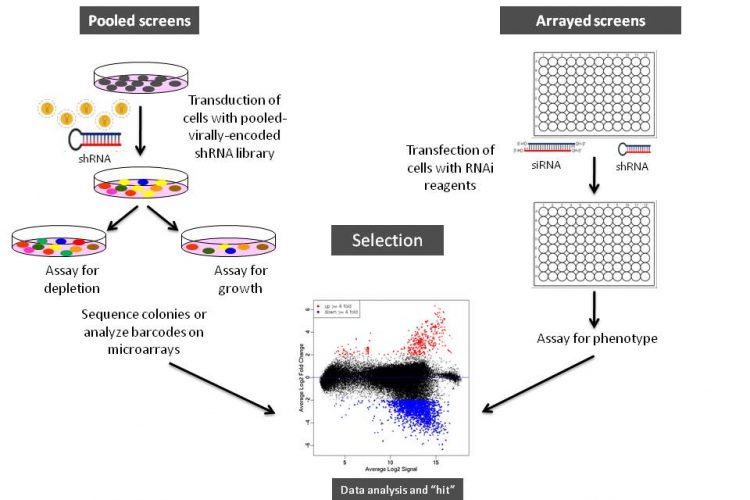
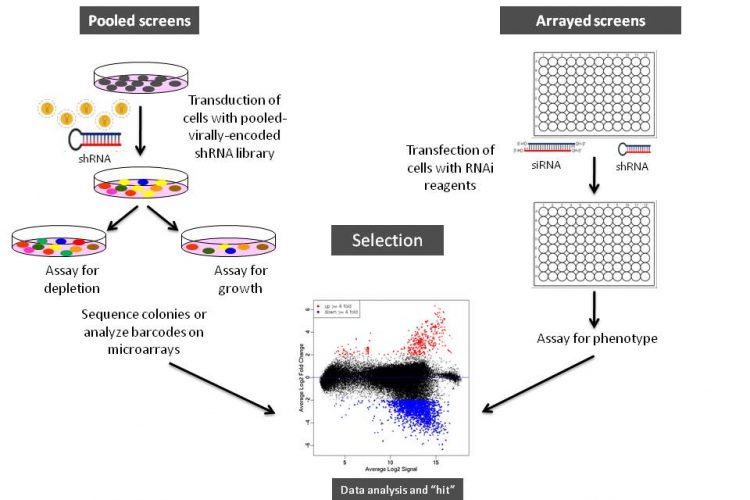
Figure 1 Schematic illustration of arrayed or pooled RNAi screens in cells. Left panel. Pooled-viral vectors encoding libraries of shRNAs targeting multiple genes can be used to transduce a target cell population in a single tissue culture dish. After selection for the desired phenotype, cells are analysed for the identification of genes whose inhibition by RNAi knockdown cause the specific phenotype as described in Table 1. The relative abundance of each shRNA or a random 60-mer barcode expressed from the same vector as the specific shRNA can be identified and quantified by labelling the PCR product with fluorescent dyes (e.g., Cy5 or Cy3). The PCR products are then hybridised to custom designed cDNA microarrays containing barcode or shRNA complementary oligonucleotides. The relative abundance of barcodes obtained from the cells that were exposed to selective pressure are compared to that detected in control cells that have been exposed to the same shRNA library, but not to the selective pressure (for example, drug treatment or genetic mutations). Right panel. Arrayed RNAi screen libraries consist of individual siRNA or shRNA reagents that target different genes and that are placed in each well of a multi-well plate. RNAi reagent libraries can comprise synthetic siRNAs, plasmid-or virally-encoded shRNAs. Various assay readouts are used to determine the effect of RNAi on the phenotype as described in Table 1. Adapted10.
Recent advances in RNA interference (RNAi) technology and availability of RNAi libraries in various formats and genome coverage have impacted the direction and speed of drug target discovery and validation efforts. After the introduction of RNAi inducing reaagent libraries in various formats, systematic functional genome screens have been performed to query the functions of individual genes, pathways or an entire genome in many disease areas, including cancer, viral pathogenesis and others. As a consequence of these screens, novel mediators of cellular response to disease pathogenesis or treatment approaches have been identified leading to the discovery of novel drug targets, development of combinatorial treatment approaches and patient selection biomarkers.
Herein, I review RNAi screening strategies and formats, assay readout methodologies, experimental design and data analysis, optimisation and quality controls, and identification and validation of candidate genes. I further explore some of the technical issues and concerns, particularly concerning cell types and validation of hit genes and future perspectives for this challenging but extremely powerful technology.
While completion of the mapping of the human genome was one of the greatest achievements of our time, its potential societal impact will be fully realised only if genomic regions that are responsible for various traits of medical importance, such as response to a drug or susceptibility to a disease, can be identified. For example, many key targets remain ‘undrugged’ and as a consequence, there is a need to develop new approaches to accelerate drug target discovery and validation, identify disease specific targets and determine the relevance of sequence or isoform variants to the disease of interest. Embarking on this pursuit requires high-throughput, genome-scale comprehensive functional analyses. Although progress in genome sequencing and annotation has enabled biological assays to be performed and analysed in a high-throughput fashion, emergence of RNA interference (RNAi) technology has accelerated this pace and has led to the development of genome-scale RNAi screens in which the effects of gene silencing on biological phenotypes can be systematically interrogated.
RNAi, which is a naturally occurring sequence-specific gene silencing phenomenon induced by double-stranded RNA (dsRNA) that can operate at both transcriptional and posttranscriptional levels1-4 was initially used to silence the function of individual genes of interest. In cells, upon processing of dsRNA into 21-23 base pair small interfering RNAs (siRNAs) by Dicer (type III ribonuclease), one of the siRNA strands is incorporated into RNA-induced silencing complex (RISC), subsequently leading the guide strand within RISC to hybridise to target mRNA and cleave it or block translation if the guide strand does not have perfect complementarity with the mRNA. Although RNAi appears to be evolved as a natural defence against viruses or transposons and induced by endogenous miRNAs encoded in the genome, RNAi can also be triggered by exogenous dsRNAs that have been introduced to the cell in the form of synthetically made or vectorencoded (plasmid or virus) short hairpin RNAs (shRNAs) or siRNAs5-8.
Recent developments in different RNAi reagent technologies and the availability of RNAi libraries in various screening formats from commercial and academic sources have allowed researchers to conduct genome-scale or targeted genomic screens to identify novel therapeutic targets rapidly and cost effectively10. RNAi screening technology can facilitate global scale functional genomics studies by streamlining the process from the initial target identification to the validation of those targets as well as development of a drug10,11 or combination drug treatment strategies (Seyhan A. A., unpublished results).
In genome-scale RNAi screens, there is no requirement for a priori knowledge of what types of targets will be discovered. Thus, genes that are neither classical essential nor pathway specific may be discovered. This includes those which are lineage-specific targets and/or genetic synthetic-lethal targets6. Another advantage of RNAi-based reverse genetic screens is that RNAi screens reveal the sequence identity of all known genes immediately after the screen is complete since it interacts with the target through Watson-Crick base-pairing, permitting more sophisticated data analyses on previously identified (and previously overlooked) genes12. Thereby, RNAi technology enables functional genomics screens to be performed rapidly using loss-of-function of a family of genes or pathways or the entire genome relatively easily. By inhibiting gene expression at the posttranscriptional level, RNAi acts like a bio-pharmacological compound, although more specifically. Since it can target nondruggable and non-protein coding sequences, it provides new opportunities for targeting previously undruggable targets.
In principle, RNAi screening is similar to ‘classical’ genetic screening, such as the foundation of assay platforms, executing screens and identifying candidate targets followed by a validation step. RNAi screens are fundamentally a forward-genetics screen. Now that the technology has matured, RNAi screening has become a routine research tool in the functional genomics field. RNAi screening has already led to a large number of results with clinical implications including the identification of novel oncogenes for the development of new therapeutics13 as well as previously unknown genes involved in cancer drug resistance and viral or bacterial pathogenesis10.
Although problems associated with sequence-dependent and independent off-targeting effects and issues related to delivery of these reagents to target cells still exist, recent improvements on reagent design algorithms and various advancements in delivery technologies have mitigated some of these problems14-18. Moreover, earlier screens have led to the establishment of more coherent and robust screening guidelines with appropriate controls and down-stream validation processes.
In this review, I discuss various types and formats of RNAi screening as well as screening strategies, and issues or concerns related to this technology for the identification and validation of novel targets.
RNAi reagents
Various formats and types of RNAi reagent libraries used for screening generally fall into two categories: (i) synthetic siRNAs that are transfected directly into cells, and (ii) vectorencoded shRNAs that are then processed into siRNAs by a cell’s endogenous RNAi machinery (or siRNAs that are expressed from opposing RNA polymerase III promoters in the form of siRNAs8). As depicted in Figures 1 and 2, often the cell type employed and duration of the screen dictates the type of RNAi library reagent format. Therefore, chemically synthesised siRNAs and vector-encoded shRNAs have been used extensively either as arrays in multi-well plates or as pools to transduce cells in bulk (Figure 1).
Since siRNAs induce transient gene silencing and are finite in time, they are mainly used in screens that can be assayed in less than five days and in cells that are amenable to transfection. If difficult to transfect cells must be used for screening or inhibition of a gene-product requires longer time, vector-encoded RNAi reagents should be employed. Because cell types often dictate the delivery options, delivery systems that include use of lipid-based or other transfection methods such as electroporation for synthetic siRNAs, shRNAs, enzymatically prepared siRNAs (esiRNAs) or dsRNAs, or viral vector mediated transduction are the most commonly used strategies in mammalian cells13.
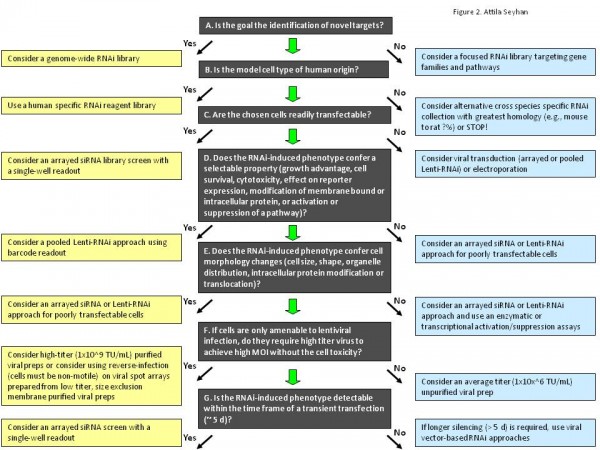
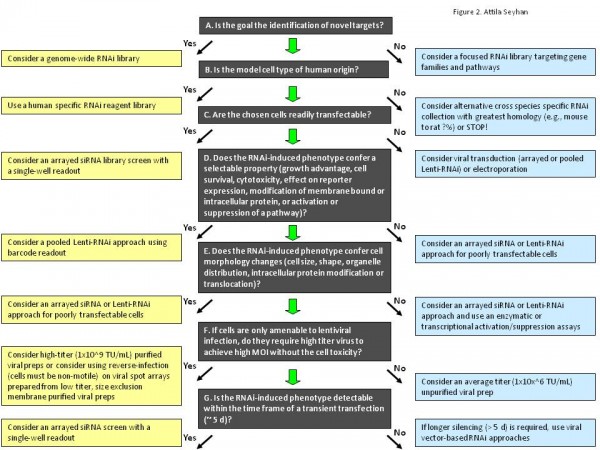
Figure 2 A general guideline for selecting appropriate RNAi screening strategy, experimental format, RNAi reagent libraries in RNAi screens. Technical feasibility is always the first consideration in making the choice of screening method, experimental format and, RNAi reagents. Some key questions and paths to follow when making these choices are depicted. LOF: Loss-of-function. Adapted9.
RNAi library coverage
Currently, various formats and types of RNAi reagent libraries (Figure 1) covering a subset of target class gene families, druggable genome or entire genomes of various organisms19 are commercially available. The RNAi collections can be customised to target any number of genes, pathways, particular gene families (kinases, phosphatases, GPCRs, etc.), biological processes or entire genomes of human, mouse or rat, permitting a systems biology approach for identifying the role of a particular gene within a cell. These advances have enabled many laboratories to perform large-scale RNAi screens using either vector derived shRNAs or synthetically made siRNAs at the genome level or targeted to specific gene families for the analysis of single gene functions as well as characterisation of functional gene clusters and dissection of cellular signalling networks.
Generally, the type of biological question and the scale of the study dictate the type of screening strategy and design to be used. In a more holistic approach, one can perform a genome-scale RNAi screen in cells to elucidate the functions of all possible regulators of a biological process leading to a more comprehensive overview of the types of genes involved in a less well-understood process. In genome-scale RNAi screens, the objectives are: (i) to assess the magnitude of impact on a biological response as a result of suppressed gene expression and (ii) to select RNAi reagents that display the most significant and quantifiable effects on the biological response that is being measured.
The alternative is a more focused ‘targeted’ approach which probes the function of a set of target genes in a specific pathway that is hypothesised to exist, but whose molecular identity is not known. RNAi screens are most effectively used when a focused gene family was the target, including druggable genome, kinases, tyrosine kinases, phosphatases, GPCRs, transcripton factors, nuclear receptors, cell cycle regulators, apoptosis, signalling molecules, proteases, ion channels, transporters and membrane traffic proteins. For example, Bernards et al. have identified the deubiquitinating (DUB) enzyme responsible for removing ubiquitin from the Fanconi Anemia D2 protein in a retroviral RNAi screen using 55 DUB knockdown vector sets in a Western blot format20. In another study, researchers used a focused siRNA collection targeting 510 genes including known and predicted kinases to identify factors that modify cellular sensitivity to TRAIL-induced apoptosis21. The study revealed many well-characterised genes in the apoptosis pathway and several novel modulators including DOBI, a gene required for progression of the apoptotic signal through the intrinsic mitochondrial cell death pathway, and MIRSA, a gene that acts to limit TRAIL-induced apoptosis. Furthermore, Murrow et al.22 performed a siRNA screen targeting the human tyrosine kinome and identified the G2/M checkpoint protein WEE1 as a potential therapeutic target.
RNAi screening formats
As illustrated in Figure 1, RNAi libraries are primarily used in two screening formats: (i) arrayed screening, in which each RNAi is in one well of a multi-well plate and (ii) pooled-screens in which pools of shRNA expressing viral (e.g., retrovirus or lentivirus) vectors are used to transduce target cells in bulk, which are then selected for the phenotype of interest.
The large collections of siRNAs or vector-encoded shRNAs covering the entire human or mouse genome have been used to conduct genome-wide RNAi screens in either arrayed or pooled formats23-31 followed by the measurement of phenotypic alterations after the introduction of RNAi reagents into the cells.
Both synthetic siRNAs and vector-encoded shRNAs are well suited for arrayed screens, where RNAi reagents targeting one gene are introduced into cells in a single well, followed by the measurement of phenotypic changes induced by RNAi several days after the introduction of RNAi reagents into the cells. Although much simpler in its application and data deconvolution, the logistics involved in arrayed screening is much more complex, requiring costly RNAi reagents, liquid handling stations and a robust LIMS system for sample tracking.
Conversely, pooled screens, although appearing complex, can deliver genome-wide RNAi reagent libraries in a single tube format and have the potential to greatly increase the number of large-scale analyses that can be performed without the requirement of laboratory automation. Additionally, viral RNAi constructs enable the generation of cell lines that express RNAi reagents from constitutive or inducible promoters, allowing constitutive or regulatable, and long term silencing of gene expression. This enables the analysis of many relevant biological questions for a longer time period in cell lines as well as primary and stem cells that are not amenable to conventional transfection methods. Following the trans – duction of cells with the vector library, the cell population is subjected to a selective pressure for a period of time to permit the RNAi-mediated loss-of-function of gene expression to reveal specific cell phenotypes or change of growth patterns or viability, followed by the identification of RNAi reagents that are either enriched or depleted from the population.
Libraries consisting of the whole genome, a particular pathway or a subset of gene families using retroviral24,29,30 or lentiviral27,32 vectors to deliver shRNA or miRNA-based shRNAs (shRNAmir) (Seyhan et al., in preparation) have been employed to conduct genome-wide RNAi screens. These types of screens are useful for examining cell survival in cancer, and can be extended to compare different populations to identify genes involved in susceptibility to drug treatment25, comparison of genotypic variation23 or comparison of distinct cancer subgroups28. For example, subset or complete genome-wide RNAi screens were conducted to identify genetic interdependencies in human cancer20,28,33-35. RNAi screening was used to identify synthetic lethal interactions in cancer cells harbouring mutant KRAS which revealed that cells that are dependent on mutant KRAS confers sensitivity to suppression of the serine/threonine kinase STK33, although STK33 is not required by KRAS-independent cells35. In another wholegenome RNAi screen, Ngo et al.28 discovered genes linked to cell survival in two distinct diffuse large B cell lymphoma subtypes.
The pooled RNAi screens have been most successfully used in positive selection screens where RNAi-mediated gene silencing results in a phenotype that is easily identifiable, such as colony formation or growth in soft agar23,26,31,36, cell rescue from cytotoxic or cytostatic influence, cellular proliferation, membrane bound or intracellular protein modification, or activation or suppression of a pathway (i.e., promoter activation using reporter genes)24,26,31. For example, RNAi screens identified the mechanisms that control the cell surface expression of the pro-apoptotic Fas receptor in Fas receptor-negative, K-Ras positive NIH 3T3 cells31. The screen revealed a mechanism by which the oncogene Ras-dependent epigenetic silencing of the Fas gene permitted tumour cells to escape apoptosis. The genes whose inhibition permitted cells to escape apoptosis were identified by sequencing or analysis of shRNA or barcode sequences by custom cDNA microarray analysis (Figure 1, page 16)31.
On the other hand, the negative selection screens are more complex than positive selection screens, because one has to select for RNAi reagents that are lost from the populations of transduced cells. To identify those RNAi reagents from the cell populations that are depleted in the screen, a molecular bar-coding strategy has been developed. Towards this goal, a unique 60-mer barcode sequence linked in cis to each shRNA or one of the strands of the shRNA sequence itself can be used to identify cell-lethal or drug-sensitive RNAi reagents that were depleted29,30 or enriched24,25 during the course of screens. The identities of genes whose inhibition confer cells to be sensitised or enriched are then identified by competitive hybridisation of oligonucleotide barcodes before and after selection or treatment in a microarray29,30,37,38. Thus, the bar-coding strategy for the identification of candidate ‘hit’ genes is suitable for both positive and negative selection screens. For instance, positive selection barcode screens have identified novel drug targets, probed the drug host interaction and identified genes and pathways that resulted in resistance or sensitivity to a specific agent25,27,31. RNAi screens have also been successfully used to identify host factors involved in viral or bacterial pathogenesis including HIV39,40, HCV41-43, influenza44, WNV45 and brucella46.
The experimental endpoint readouts
The outcome of an RNAi screen depends on methods for detecting the desired phenotype, easily, robustly and in a high-throughput fashion. For this, most of the many functional assays can be customised to a multi-well plate-based format to analyse the effect of RNAi silencing of gene expression. The readout system must be robust to avoid background noise, but sensitive enough to detect subtle changes induced by RNAi reagents.
Readout systems, as summarised in Table 1, must involve a selectable alteration in cell phenotype such as cell viability or cell proliferation, apoptosis or altered expression of a protein that can be detected by flow cytometry (i.e., a surface marker expressed through activation of a promoter or a signalling pathway, a florescent reporter activated by promoter activation, or splicing of a reporter gene), enzymatic assays (e.g., ELISAs), or high content image analysis. Additionally, changes in protein-protein associations can be measured by fluorescence resonance energy transfer and changes in phosphorylation status can be monitored with phosphorylation-specific antibodies9,47,48. In addition, any combination of these assays can be performed to address different biological questions (e.g., RNAi and drug synthetic lethal screens).
In a pooled RNAi screen, the ‘readout’ must confer a selectable change in cell phenotype, cell viability or proliferation, or altered expression of a protein that can be detected by flow cytometry as described above. Cells that show the desired phenotype are analysed to determine if any genes silenced by RNAi are responsible for producing the phenotype.
Experimental design considerations and data analysis
As illustrated in Figure 2, setting up an RNAi screen presents many challenges including identifying the most suitable experimental system to answer the biological question of interest, choosing the screening strategy (genome-wide or a more focused screen of a subset of genes, gene families, pathways, etc.), use of individually arrayed RNAi reagents or pooled vector-derived RNAi screens, developing robust and reproducible readout assays for the identification and characterisation of candidate genes. Other technical considerations such as delivery of RNAi reagents to target cells, the use of proper controls (e.g., positive, negative and nonsilencing), elimination of cell culture assay related artefacts (e.g., well, plate, and edge effects), the choice of statistical methods for data analyses and criteria for candidate ‘hit’ gene identification and validation of these genes in appropriate systems, have been noted. All these require careful planning and execution of experiments, upfront assay optimisations and small scale pilot screens followed by the execution of the actual screen, validation of candidate ‘hit’ genes and the re-examination of biological question of interest in appropriate cell types, such as cells obtained from patient samples or animal models.
Moreover, cells are controlled by the dynamic actions of many genes interacting with one another. Because of the existence of these dynamic complex gene networks as well as signalling cascades, the loss-of-function of any gene by RNAi may affect other genes, pathways or the entire network or signalling cascade49. It is rare that a siRNA has exactly no impact on a measured biochemical response; most siRNAs have some effect in a genomescale RNAi screen.
Because of these reasons, in RNAi screens, specific and non specific off-targeting effects can lead to the false discovery of candidate genes. Off-targeting effects can occur: (i) if a sequence of RNAi reagent is identical or nearly identical to a sequence of an irrelevant mRNA, leading to a phenotype or production of a false positive result (Jackson et al., 2003); (ii) if sequences that are identical to ‘seed’ sequences of the natural miRNAs result in unwanted miRNA-like unrelated gene silencing by pairing with a complementary sequence in the 3’untranslated region (UTR) of an unrelated mRNA leading to mRNA degradation or translational block (Jackson et al., 2006b;14,15,17; (iii) if double-stranded RNA mediated activation of the antiviral type I interferon response in a sequence-independent fashion (by not targeting irrelevant mRNAs) (e.g., PKR signalling)50 although this problem is usually exacerbated when saturating concentrations of siRNAs are introduced to cells18, and (iv) if Toll-like receptor (TLR)51 or other cellular signalling pathways are activated. In some cases, this has been traced to the presence of GU-rich motifs in the siRNA or shRNA16 (e.g., 5’-UGUGU-3’ or 5’-GUCCUUCAA-3’) and has only been observed with primary peripheral blood leukocytes and plasmacytoid dendritic cells.
Careful choice of the siRNA duplex sequence and designing siRNA sequences devoid of any of the above mentioned sequences minimise or eliminate these types of off-targeting effects. However since most RNAi reagent libraries are available commercially, this requires an initiative by the manufacturer of these libraries. Because of this, before embarking on any large scale RNAi screen experiments, an experimental plan has to be drafted and tested in a pilot study where every variable in the screen must be optimised.
To further minimise or eliminate the detrimental RNAi reagent and methodologyinduced off-targeting effects, multiple non-targeting or scrambled RNAi reagent sequences should be used extensively in the RNAi screens. In addition, interferon responses and cell activation and/or differentiation that might be induced by the delivery methods or reagents used for the RNAi reagent delivery to target cells must also be examined to identify the source of any off-targeting effect during the optimisation and pilot screen phase of screens. Furthermore, extensive use of statistical tests that are sensitive to the unique dynamics of RNAi screens and standards for performing and reporting screening data will improve robustness of the screens and will further reduce false discovery rates.
A well designed and executed large-scale screening assay must have a robust signal-to-noise ratio and low inter and intra-plate and assay variation. To achieve this, it all begins with a careful planning of the screen. This begins with a decision making process (Figure 2) on the type of biological question being interrogated and type of answer being sought as well as the type of tools, methods and assays to be used in the screen. After a decision is made on the specifics of the screen design, a pilot screen must be executed to optimise assay conditions in the screen following the general guidelines shown in Figure 3. In the optimisation phase of the screen, efficacy, specificity and potency of control RNAi reagents targeting known genes as well as the efficacy of transfection reagents and end point read out assays are examined. In addition, during the optimisation study, duration of gene silencing, cell densities, plate formats, (96-well or 384-well), number of assay replicates and any other assay variables must be ‘titrated’ to optimise assay conditions52. Moreover, multiple mock treated and untreated samples should be included in the analysis to control for the general variables in the screening process. Positive RNAi reagents against predefined housekeeping genes, (e.g., GAPDH) and negative control RNAi reagents (multiple non-targeting RNAi reagents) should be tested to determine baseline, signal and standard deviation (s.d.) values for the system. Once the conditions are optimised, a small-scale pilot screen should be performed using a subset of the RNAi reagent library to evaluate the overall performance, reproducibility and robustness of the screen. Once the confidence with the screen is achieved, the actual screen is performed in multiple technical replicates (ideally three) as illustrated in Figure 3 on page 20. The raw data from the screen is collected and analysed to calculate quality control parameters, such as outliers between replicate measurements, plate effects and other variables, and to identify the measurements for downstream analysis12.
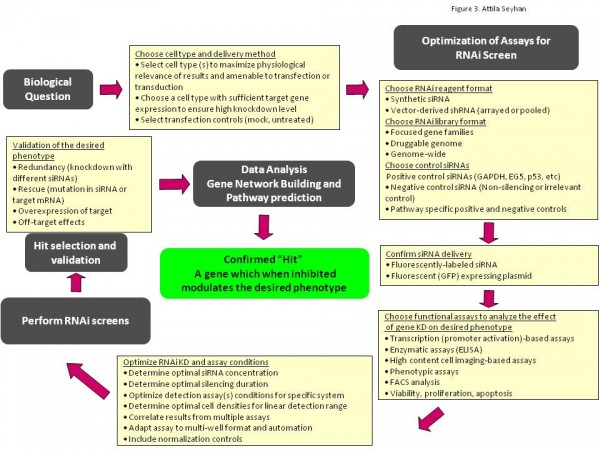
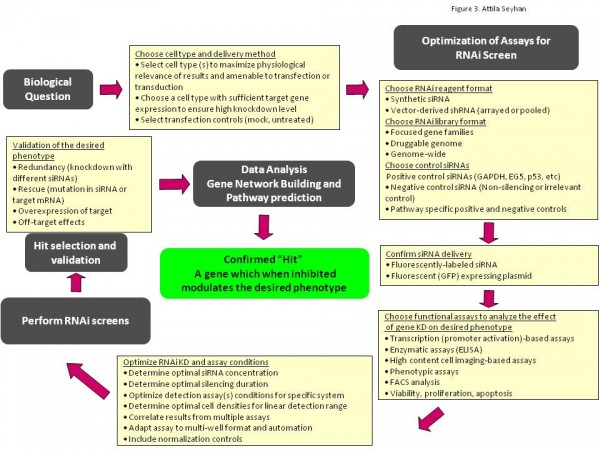
Figure 3 A general guideline for successful RNAi screens. RNAi screening workflow requires a careful planning, selection of appropriate cell types for the biology being interrogated, RNAi reagents and reagent formats, reagents for the delivery to target cells, RNAi and reagent controls, endpoint readout assays, and data analysis and selection of candidate ‘hit’ siRNAs and their cognate target genes and finally confirmation of ‘hits’. A pilot screen is required to optimise the conditions for the screen as well as strong bioinformatics and biostatistics support for proper analysis of large data sets, hit selection, candidate gene annotation, gene network building and pathway prediction.
In addition, the raw data is normalised to a control or set of controls and ranked according to predefined criteria such as the significance of the phenotypes compared with positive or negative controls12. The analysis of data from a screen can be expedited by using various computational data analysis packages to normalise the data and to correct for plate position effects and batch-to-batch variations. For example, several software solutions (e.g., cellHTS, Spotfire/Tibco) can be applied for normalisation of the screen and for identifying the initial candidate gene ‘hit’ list19.
Recently, the utilisation of multiple RNAi reagents targeting the same gene has become a standard procedure both to maximise the identification of a true phenotype and to accelerate the validation phase, since at least two siRNA molecules in the pool should capture the phenotype. Because of this, the rank of hits can be further scored if multiple RNAi reagents targeting the same gene were used in the screen. The general quality of the screen and noise in large data sets can be measured, with Z’ factors, as a measure of the statistical power of the assay to discriminate between positive and negative hits53 before hits are selected on the basis of predefined thresholds. The Z’ factor, a measure of statistical effect size often used in high-throughput screens as an indicator of assay quality can be used to determine which of a large number of possible assay formats is robust enough for a screen12. The Z’ factor is calculated by using several replicates of the assay with positive and negative controls. A value of > 0.5 (ideally > 0.7) suggests a suitable difference between signal and background values together with low variability. An assay with a Z’ – factor between 0.5 and 1 is considered excellent12. However, for RNAi, 0.5 is considered acceptable since there are many more variables in this type of screen.
As a general rule, candidate ‘hit’ genes are identified when assay replicates that are beyond two to three standard deviation (s.d.) values from the mean or beyond a predetermined phenotypic threshold (for screens that assess morphology or other parameters that cannot be readily quantified). This is termed the Z-score and defined as a measure of the distance in standard deviations of a sample from the mean12. Although mean and s.d. values may be calculated with control samples, a sample-based approach where the entire RNAi library as de facto non-functional control or negative controls can be used as well. Sample-based normalisation relies on more experimental data; thereby, it can lead to more accurate data analysis. If good positive and negative controls are not available, the sample-based approach should be used as a negative control54. Hits with an s.d. of > 5 from the mean should be excluded from the analysis so as to not to skew the calculated mean values. Both the array- and pool-based screens should be done at least in triplicate, to minimise false discovery27,28.
Validation of candidate ‘hit’ genes
As discussed earlier, RNAi reagents are known to have nonspecific off-target effects, including activation of innate immune responses (e.g., PKR signalling)50, TLR activation51, and miRNA like activities14,15,17. In addition to the inherent characteristics of siRNA design that leads to false discovery in RNAi screens, sub-optimal experimental design or execution can contribute to false-positives and -negatives in large-scale RNAi screens. Other factors that may contribute to the false discovery in RNAi screens are: use of poorly designed RNAi reagents, lack of appropriate controls and insufficient number of replicate plates, inappropriate tissue culture practices including issues related to well and plate effects (e.g., edge effect), or lack of statistical analyses for the specific screen performed13. These often skew the data. Because of this, several strategies and methodologies have been used for experimental confirmation of candidate ‘hits’ identified from large-scale RNAi screens and to distinguish true hits from the false hits.
Following the analysis of the primary screen data, the goal is to identify high scoring genes, annotate the hits and determine which biological processes are represented by the high scoring genes. To search for Gene Ontology (GO) categories that are enriched for high-scoring genes, several data analysis packages are available. For example, the Bioconductor Category package authored by Robert Gentleman55 compares the distribution of scores of genes in a category with the overall distribution. Interesting categories are often those that separate from the mean plot; they have both a large difference in means as well as a small P value.
To confirm that an observed phenotype is the result of RNAi effect, ‘redundancy or rescue’ experiments are used as the widely recognised strategy56. This is achieved by using an independent set of RNAi reagents (i.e., from different vendors) targeting different sites on the same gene or reversing the phenotype induced by the RNAi by expressing a cDNA that has a mismatch mutation with the RNAi reagent in the targeted site. If a library of pooled siRNAs (e.g., four siRNAs per well targeting the same gene) are used in the screen, the candidate pools must be deconvoluted into single sequences after the screen to identify the most potent siRNA that is responsible for the phenotype. From the screen, at least two siRNA molecules in the pool should confer the phenotype and there should be a clear correlation between mRNA, protein depletion and phenotypic severity.
RNAi knockdown by a single siRNA is demonstrated by using qRT-PCR and immunoblotting and correlating the results with the observed phenotype where the candidate gene phenotypes should be reproducible with at least two or more independent RNAi reagents19. Alternatively, to rescue the phenotype later, siRNAs can be designed to target the 3’UTRs such that the rescue construct can then be designed without the 3’UTR, becoming resistant to the siRNAs19. Redundancy, if done thoroughly, is usually sufficient to confirm specificity. This is because multiple RNAi reagents acquired from different vendors feature different sequence characteristics and often target different sites of the same gene. If the phenotype is due to inhibition of a particular gene, these RNAi reagents, if functionally efficient, should lead to the same phenotype. If multiple RNAi reagents targeting a single gene are identified as hits, this redundancy is considered to be accomplished. Additionally, to confirm that hits are gene and phenotype specific, the same assay in different cell type or secondary assays with different end point readouts should also be used (e.g., microscopy-based translocation assays or immunoblot analysis).
Moreover, RNAi induced phenotypes should be associated to the related disease or disorder by mapping disease-associated regions, mutations and amplifications via gene-and pathway level validation (disease association is indicative of a relevant phenotype)13. Gene and pathway level validation can also be performed by examining protein-protein interactions. For example, coimmunoprecipitation, mass spectrometry and yeast two-hybrid screens can often reveal physical interactions among newly identified proteins13.
To establish network relationships among a set of genes identified from the screen, database mining and bioinformatics are commonly used (e.g., Ingenuity™)57. Hits may be grouped by end points themselves or grouped by hierarchical clustering or principal-components analyses. For screens that test multiples for each gene, these methods allow detection of off-targeting effects through clustering. A siRNA that results in an off-targeting effect will induce a different set of phenotypes and therefore fall into a separate cluster from the other siRNAs targeting the same gene58. The candidate genes in a pathway should be investigated further to elucidate their exact mechanism of action in the respective signalling pathway(s). For example, to verify if two genes selected in the screen are in the same pathway, simultaneous gene silencing should be performed with combination of RNAi reagents targeting two different genes. Finally, genetic analysis in vivo to examine effects of mutations of gene hits in whole animals is fundamental for validating the phenotype in an in vivo system.
Validation of candidate genes is often more complex and time consuming than the primary screen itself. In order to facilitate the validation phase of the screen, bioinformatics tools such as pathway analysis tools (e.g., Ingenuity™) should be used which subject the candidate genes to pathway prediction analysis to determine whether a number of hits from the screen cluster into the same biological pathway or have a well-established physical or functional interaction.
Challenges and future perspectives
The genome scale loss-of-function screens mediated by RNAi have shown their capability to identify novel targets and pathways providing important insights in individual genes and associated molecular networks that can be explored for developing novel therapeutic strategies. However, translating in vitro functional data into in vivo systems often results in poor correlation of in vitro phenotype to in vivo systems.
This is partly due to the limited under – standing of the underlying biological systems that is being interrogated, the technical issues related to screen design, methods, reagents, and assays used in the screen, as well as statistical methods used for data analysis. The RNAi ‘hits’ or ‘phenocopy’ results from a primary screen must be validated to establish the biological effect independent of the cellular context and under the influence of the complex in vivo environment. When using human cells, it must be ensured that the cell type or line selected for evaluation is directly relevant to the disease being studied. Classical recombinant or transformed cell lines (e.g., HEK293, HeLa, etc.) conventionally used in RNAi screens are not the best choice to study the biology of different diseases or disorders such as T or B cell receptor signalling, cancer or viral host interactions. Additionally, the genes identified from the screen should be validated in appropriate cell types, such as cells obtained from patient samples or animal models.
Screening efforts should incorporate integrative and multiplexed strategies such as screening of multiple cell types or lines, pathogens, active compound treatments as in synthetic lethal screens and assays, and capture multiple assay readouts per screen. Furthermore, technical issues related to improved design of RNAi reagent libraries, improved methods for RNAi screening and assays for endpoint readouts, continued efforts at standardisation of data analysis, along with the subsequent improved bioinformatics should improve both the screening effort itself as well as the analysis of RNAi screening results.
Since validation of the candidates sometimes takes much more time than the actual screen itself, new strategies for large-scale screens or new methods for rapid confirmation of screen results need to be developed. Making the primary data more robust is one way to introduce a new paradigm into the large-scale screens which can reduce the lengthy validation phase of an RNAi screen. For example, efforts at designing next generation virally-encoded RNAi reagent libraries where each gene is targeted by more than 10 shRNAs are promising strategies. This strategy introduces a built-in RNAi reagent redundancy into the library potentially precluding the need for validation of hit genes by redundant shRNAs targeting different sites of the same gene after the primary screen.
In arrayed screens, performing RNAi screens in high density formats in 1536 well plates or on microarray chips can greatly increase the throughput capacity of cell based assays59. The use of high density RNAi screens enables the screens to be performed rapidly, are cost effective60, and permits the use of expanded genome coverage in a few plates or chips leading to possible ultra-throughput combination screens with compounds61.
Since the biological question also relates to a tissue and whole organism rather than a specific cell type, screens that are performed in an environment that closely resembles the three-dimensional environment of the actual tissue would be an ideal strategy. Strategies such as co-culturing cells or employing threedimensional cell culture systems can be practiced to recreate complex, functional tissue ex vivo environment for validating these candidate genes62. Now that three-dimensional cell culture is emerging as a powerful and versatile tool, RNAi screens performed in such environments should translate into in vivo systems more effectively than the twodimensional monocultures grown in two-dimensions as monolayers62. For example, direct screening of primary tumour cells isolated from a tumour sample can be used in RNAi screens. An approach, termed ‘direct functional ex-vivo screening’ has been used successfully to examine the vulnerabilities of primary leukaemia cells from 30 patients using a focused siRNA library that individually assesses targeting of each member of the tyrosine kinase gene family63. Therefore, the direct functional ex vivo screening of cells obtained from patients could, in principle, identify novel targets, pathways, and vulnerabilities that can be translated directly into humans.
It has also become clear that threedimensional assays can reveal the identity of genes that are required for anchorageindependent growth in cancer cells that two-dimensional tissue culture assays may not capture. For example, demonstration of anchorage-independent growth of tumour cells grown in soft agar in a three-dimensional environment is one way of determining the tumorigenicity of cells in tissue culture which can yield results that closely match those of xenograft assays64. In addition, conducting RNAi screens in three-dimensional soft agar format can reveal pathways and novel targets whose suppressed expression results in a slowed or suppressed tumour growth with a more physiologically relevant phenotype than cells grown in a two-dimensional plastic dish or plate61. To support this strategy, highthroughput soft agar assays have previously been demonstrated65,66.
Correlation of the expression levels of the selected genes to either treatment response or disease outcome can facilitate the validation of the RNAi ‘hits’. Another area of improvement may include the correlation of different types of large scale genomic datasets with the RNAi screening data to select those targets that are truly important for the biological phenotype under investigation58.
Results obtained from RNAi screens performed in cell lines or panels of co-cultured cells do not necessarily recapitulate the true in vivo consequences and clinical response. Therefore, conducting RNAi screens in vivo would be the most ideal strategy to overcome these issues. Recently, several in vivo RNAi screens in whole-organisms have been performed, mostly in Caenorhabditis elegans67,68 and Drosophila melanogaster69,70 and related Melanogaster libraries71,72. More recently, several reports have demonstrated the utility of this approach using a small panel of lentiviral RNAi libraries in mice targeting a small subset of genes73-75. Although results are promising, this approach has been mostly employed for confirmation of candidate genes, such as tumour suppressor genes74-76. In another study, researchers introduced lentiviral-shRNAs into mice to knockdown the gene encoding the immunomodulatory receptor CTLA-4. The goal of the study was to compare hypomorphic phenotypes resulting from partial knockdown with the more severe phenotypes produced by complete gene deletion77. Furthermore, pooled RNAi screens, in which populations of virustransduced cells have been proposed as a way to reintroduce libraries of cells into mice and select for loss- or gain-of-function or phenotype (e.g., cell intrinsic factors) or control the activity (e.g., differentiation, function and homing) of cells in an organism52. However, results from such a study have yet to be demonstrated. Although these screens select for phenotypes that are more relevant in vivo, they do not capture the existing heterogeneity in a population.
Conclusions
RNAi-mediated functional genomics screens have gained wide acceptance from academia to industry towards the discovery of novel genes that encode molecules with previously unknown functions in a biological system. As demonstrated by various findings reported here and elsewhere10,19,52, the application of RNAi screening technology has been successful in examining important biological questions and to identify novel, important insights in individual genes and the associated molecular networks that can be explored for therapeutic intervention. Consequently, many of the RNAi screens have resulted in the identification of novel modulators, alternative signalling and disease pathways, and drug targets and biomarkers in areas including cancer, general cell biology, RNA biology, cancer and viral pathogenesis10.
However, because of the challenges and limitations related to RNAi technology, screening methodology and the underlying biological model systems, the identified genes still require extensive validation to establish a relationship to the in vivo biological effect independent of the cellular context.
Nonetheless, with the recent improvements in RNAi reagent libraries and extensive use of strong bioinformatics support, interpretation of RNAi screening results will be more robust and easier, leading to the identification of novel drug targets, biomarkers and disease pathways that can deliver tangible results that impact not only the basic science but also translational medicine. In addition, the correlation of different types of large scale genomic data with the results of RNAi functional genomics screens can be used to select those targets that are truly important for the biological phenotype of interest.
Acknowledgements
I would like to thank Bruce Jacobson, Jennifer Weissert, Steven A. Haney, Okechukwu Ukairo, Jocelyn Jakubik and Karl Nocka for critical reading of the article.
References
1. Dykxhoorn, D. M., Novina, C. D. & Sharp, P. A. Killing the messenger: short RNAs that silence gene expression. Nat Rev Mol Cell Biol 4, 457-467 (2003)
2. Meister, G. & Tuschl, T. Mechanisms of gene silencing by double-stranded RNA. Nature 431, 343-349 (2004)
3. Zamore, P. D. & Haley, B. Ribo-gnome: the big world of small RNAs. Science 309, 1519-1524 (2005)
4. Chapman, E. J. & Carrington, J. C. Specialization and evolution of endogenous small RNA pathways. Nat Rev Genet 8, 884-896, doi:nrg2179 [pii] 10.1038/nrg2179 (2007)
5. Whitehead, K. A., Langer, R. & Anderson, D. G. Knocking down barriers: advances in siRNA delivery. Nat Rev Drug Discov 8, 129-138, doi:nrd2742 [pii] 10.1038/nrd2742 (2009)
6. Quon, K. & Kassner, P. D. RNA interference screening for the discovery of oncology targets. Expert Opin Ther Targets 13, 1027-1035, doi:10.1517/14728220903179338 (2009)
7. Seyhan, A. A., Alizadeh, B. N., Lundstrom, K. & Johnston, B. H. RNA interference-mediated inhibition of Semliki Forest virus replication in mammalian cells. Oligonucleotides 17, 473-484, doi:10.1089/oli.2007.0079 (2007)
8. Seyhan, A. A. et al. Complete, gene-specific siRNA libraries: production and expression in mammalian cells. RNA 11, 837-846, doi:11/5/837 [pii] 10.1261/rna.7285805 (2005)
9. Echeverri, C. J. & Perrimon, N. Highthroughput RNAi screening in cultured cells: a user’s guide. Nat Rev Genet 7, 373-384, doi:nrg1836 [pii]
10.1038/nrg1836 (2006) 10. Seyhan, A. A. & Ryan, T. E. RNAi Screening for the Discovery of Novel Modulators of Human Disease. Curr Pharm Biotechnol, doi:BSP/CPB/E-Pub/0077-11-5 [pii] (2010)
11. Iorns, E., Lord, C. J., Turner, N. & Ashworth, A. Utilizing RNA interference to enhance cancer drug discovery. Nat Rev Drug Discov 6, 556-568, doi:nrd2355 [pii] 10.1038/nrd2355 (2007)
12. Boutros, M. & Ahringer, J. The art and design of genetic screens: RNA interference. Nat Rev Genet 9, 554-566, doi:nrg2364 [pii] 10.1038/nrg2364 (2008)
13. Mohr, S., Bakal, C. & Perrimon, N. Genomic screening with RNAi: results and challenges. Annu Rev Biochem 79, 37-64, doi:10.1146/annurev-biochem-060408- 092949 (2010)
14. Birmingham, A. et al. 3′ UTR seed matches, but not overall identity, are associated with RNAi off-targets. Nat Methods 3, 199-204, doi:nmeth854 [pii] 10.1038/nmeth854 (2006)
15. Jackson, A. L. et al. Widespread siRNA “off-target” transcript silencing mediated by seed region sequence complementarity. RNA 12, 1179-1187, doi:rna.25706 [pii] 10.1261/rna.25706 (2006)
16. Judge, A. D. et al. Sequence-dependent stimulation of the mammalian innate immune response by synthetic siRNA. Nat Biotechnol 23, 457-462, doi:nbt1081 [pii] 10.1038/nbt1081 (2005)
17. Lin, X. et al. siRNA-mediated off-target gene silencing triggered by a 7 nt complementation. Nucleic Acids Res 33, 4527- 4535, doi:33/14/4527 [pii] 10.1093/nar/gki762 (2005)
18. Sledz, C. A. & Williams, B. R. RNA interference and double-stranded-RNA-activated pathways. Biochem Soc Trans 32, 952-956, doi:BST0320952 [pii] 10.1042/BST0320952 (2004)
19. Falschlehner, C., Steinbrink, S., Erdmann, G. & Boutros, M. High-throughput RNAi screening to dissect cellular pathways: a how-to guide. Biotechnol J 5, 368-376, doi:10.1002/biot.200900277 (2010) 20. Bernards, R., Brummelkamp, T. R. & Beijersbergen, R. L. shRNA libraries and their use in cancer genetics. Nat Methods 3, 701-706, doi:nmeth921 [pii] 10.1038/ nmeth921 (2006) 21. Aza-Blanc, P. et al. Identification of modulators of TRAIL-induced apoptosis via RNAi-based phenotypic screening. Mol Cell 12, 627-637, doi:S1097276503003484 [pii] (2003)
22. Murrow, L. M., Garimella, S. V., Jones, T. L., Caplen, N. J. & Lipkowitz, S. Identification of WEE1 as a potential molecular target in cancer cells by RNAi screening of the human tyrosine kinome. Breast Cancer Res Treat, doi:10.1007/s10549-009-0571-2 (2009)
23. Berns, K. et al. A large-scale RNAi screen in human cells identifies new components of the p53 pathway. Nature 428, 431-437, doi:10.1038/nature02371 nature02371 [pii] (2004)
24. Berns, K. et al. A functional genetic approach identifies the PI3K pathway as a major determinant of trastuzumab resistance in breast cancer. Cancer Cell 12, 395-402, doi:S1535-6108(07)00262-0 [pii] 10.1016/j.ccr.2007.08.030 (2007)
25. Brummelkamp, T. R. et al. An shRNA barcode screen provides insight into cancer cell vulnerability to MDM2 inhibitors. Nat Chem Biol 2, 202-206, doi:nchembio774 [pii] 10.1038/nchembio774 (2006)
26. Kolfschoten, I. G. et al. A genetic screen identifies PITX1 as a suppressor of RAS activity and tumorigenicity. Cell 121, 849-858, doi:S0092-8674(05)00397-1 [pii] 10.1016/j.cell.2005.04.017 (2005)
27. Luo, B. et al. Highly parallel identification of essential genes in cancer cells. Proc Natl Acad Sci U S A 105, 20380-20385, doi:0810485105 [pii] 10.1073/pnas.0810485105 (2008)
28. Ngo, V. N. et al. A loss-of-function RNA interference screen for molecular targets in cancer. Nature 441, 106-110, doi:nature04687 [pii] 10.1038/nature04687 (2006).
29. Schlabach, M. R. et al. Cancer proliferation gene discovery through functional genomics. Science 319, 620-624, doi:319/5863/620 [pii] 10.1126/science.1149200 (2008)
30. Silva, J. M. et al. Profiling essential genes in human mammary cells by multiplex RNAi screening. Science 319, 617-620, doi:319/5863/617 [pii] 10.1126/science. 1149185 (2008)
31. Westbrook, T. F. et al. A genetic screen for candidate tumor suppressors identifies REST. Cell 121, 837-848, doi:S0092-8674(05)00350-8 [pii] 10.1016/j.cell.2005.03.033 (2005)
32. Root, D. E., Hacohen, N., Hahn, W. C., Lander, E. S. & Sabatini, D. M. Genome-scale lossof- function screening with a lentiviral RNAi library. Nat Methods 3, 715-719, doi:nmeth924 [pii] 10.1038/nmeth924 (2006)
33. Downward, J. Use of RNA interference libraries to investigate oncogenic signalling in mammalian cells. Oncogene 23, 8376-8383, doi:1208073 [pii] 10.1038/sj.onc.1208073 (2004)
34. Westbrook, T. F., Stegmeier, F. & Elledge, S. J. Dissecting cancer pathways and vulnerabilities with RNAi. Cold Spring Harb Symp Quant Biol 70, 435-444, doi:10.1101/sqb.2005.70.031 (2005)
35. Scholl, C. et al. Synthetic lethal interaction between oncogenic KRAS dependency and STK33 suppression in human cancer cells. Cell 137, 821-834, doi:S0092-8674(09)00316-X [pii] 10.1016/j.cell.2009.03.017 (2009)
36. Hattori, H. et al. RNAi screen identifies UBE2D3 as a mediator of all-trans retinoic acid-induced cell growth arrest in human acute promyelocytic NB4 cells. Blood 110, 640-650, doi:blood-2006-11-059048 [pii] 10.1182/blood-2006-11-059048 (2007)
37. Whitehurst, A. W. et al. Synthetic lethal screen identification of chemosensitizer loci in cancer cells. Nature 446, 815-819, doi:nature05697 [pii] 10.1038/nature05697 (2007)
38. Luo, J. & Elledge, S. J. Cancer: Deconstructing oncogenesis. Nature 453, 995-996, doi:453995a [pii] 10.1038/453995a (2008)
39. Brass, A. L. et al. Identification of host proteins required for HIV infection through a functional genomic screen. Science 319, 921-926, doi:1152725 [pii] 10.1126/science.1152725 (2008)
40. Zhou, H. et al. Genome-scale RNAi screen for host factors required for HIV replication. Cell Host Microbe 4, 495-504, doi:S1931-3128(08)00330-2 [pii] 10.1016/j.chom.2008.10.004 (2008)
41. Li, Q. et al. A genome-wide genetic screen for host factors required for hepatitis C virus propagation. Proc Natl Acad Sci U S A 106, 16410-16415, doi:0907439106 [pii] 10.1073/pnas.0907439106 (2009)
42. Tai, A. W. et al. A functional genomic screen identifies cellular cofactors of hepatitis C virus replication. Cell Host Microbe 5, 298-307, doi:S1931-3128(09)00061-4 [pii] 10.1016/j.chom.2009.02.001 (2009)
43. Randall, G. et al. Cellular cofactors affecting hepatitis C virus infection and replication. Proc Natl Acad Sci U S A 104, 12884-12889, doi:0704894104 [pii] 10.1073/pnas.0704894104 (2007)
44. Hao, L. et al. Drosophila RNAi screen identifies host genes important for influenza virus replication. Nature 454, 890-893, doi:nature07151 [pii] 10.1038/nature07151 (2008)
45. Krishnan, M. N. et al. RNA interference screen for human genes associated with West Nile virus infection. Nature 455, 242-245, doi:nature07207 [pii] 10.1038/nature07207 (2008)
46. Qin, Q. M. et al. RNAi screen of endoplasmic reticulum-associated host factors reveals a role for IRE1alpha in supporting Brucella replication. PLoS Pathog 4, e1000110, doi:10.1371/journal.ppat.1000110 (2008)
47. Moffat, J. & Sabatini, D. M. Building mammalian signalling pathways with RNAi screens. Nat Rev Mol Cell Biol 7, 177-187, doi:nrm1860 [pii] 10.1038/nrm1860 (2006)
48. Perrimon, N. & Mathey-Prevot, B. Applications of high-throughput RNA interference screens to problems in cell and developmental biology. Genetics 175, 7-16, doi:175/1/7 [pii] 10.1534/genetics.106.069963 (2007)
49. Zhao, H. F. et al. High-throughput screening of effective siRNAs from RNAi libraries delivered via bacterial invasion. Nat Methods 2, 967-973, doi:nmeth812 [pii] 10.1038/nmeth812 (2005)
50. Sledz, C. A., Holko, M., de Veer, M. J., Silverman, R. H. & Williams, B. R. Activation of the interferon system by short-interfering RNAs. Nat Cell Biol 5, 834-839, doi:10.1038/ncb1038 ncb1038 [pii] (2003)
51. Agrawal, S. & Kandimalla, E. R. Role of Toll-like receptors in antisense and siRNA [corrected]. Nat Biotechnol 22, 1533-1537, doi:nbt1042 [pii] 10.1038/nbt1042 (2004)
52. Sharma, S. & Rao, A. RNAi screening: tips and techniques. Nat Immunol 10, 799-804, doi:ni0809-799 [pii] 10.1038/ni0809-799 (2009)
53. Zhang, J. H., Chung, T. D. & Oldenburg, K. R. A Simple Statistical Parameter for Use in Evaluation and Validation of High Throughput Screening Assays. J Biomol Screen 4, 67-73 (1999)
54. Birmingham, A. et al. Statistical methods for analysis of high-throughput RNA interference screens. Nat Methods 6, 569-575, doi:nmeth.1351 [pii] 10.1038/nmeth.1351 (2009)
55. Boutros, M., Bras, L. P. & Huber, W. Analysis of cell-based RNAi screens. Genome Biol 7, R66, doi:gb-2006-7-7-R66 [pii] 10.1186/ gb-2006-7-7-R66 (2006) 56. Echeverri, C. J. et al. Minimizing the risk of reporting false positives in large-scale RNAi screens. Nat Methods 3, 777-779, doi:nmeth1006-777 [pii] 10.1038/nmeth 1006-777 (2006)
57. Lord, C. J., Martin, S. A. & Ashworth, A. RNA interference screening demystified. J Clin Pathol 62, 195-200, doi:jcp.2008.058735 [pii] 10.1136/jcp.2008.058735 (2009).
58. Haney, S. A. Increasing the robustness and validity of RNAi screens. Pharmacogenomics 8, 1037-1049, doi:10.2217/14622416.8.8.1037 (2007)
59. Maffia, A. M., 3rd, Kariv, I. I. & Oldenburg, K. R. Miniaturization of a Mammalian Cell-Based Assay: Luciferase Reporter Gene Readout in a 3 Microliter 1536-Well Plate. J Biomol Screen 4, 137-142 (1999)
60. Pfeifer, M. J. & Scheel, G. Long-term storage of compound solutions for high-throughput screening by using a novel 1536-well microplate. J Biomol Screen 14, 492-498, doi:1087057109335261 [pii] 10.1177/1087057109335261 (2009)
61. Borawski, J. & Gaither, A. The evolution of RNAi technologies in the drug discovery business. European Pharmaceutical Review 5, 35-39 (2010)
62. Stein, R. A. in Genetic Engineering and Biotechnology News Vol. 30 1, 18, 20-21 (2010)
63. Tyner, J. W. et al. RNAi screen for rapid therapeutic target identification in leukemia patients. Proc Natl Acad Sci U S A 106, 8695-8700, doi:0903233106 [pii] 10.1073/pnas.0903233106 (2009)
64. Freedman, V. H. & Shin, S. I. Cellular tumorigenicity in nude mice: correlation with cell growth in semi-solid medium. Cell 3, 355-359, doi:0092-8674(74)90050-6 [pii] (1974)
65. Anderson, S. N., Towne, D. L., Burns, D. J. & Warrior, U. A high-throughput soft agar assay for identification of anticancer compound. J Biomol Screen 12, 938-945, doi:12/7/938 [pii] 10.1177/1087057107306130 (2007)
66. Thierbach, R. & Steinberg, P. Automated soft agar assay for the high-throughput screening of anticancer compounds. Anal Biochem 387, 318-320, doi:S0003-2697(09)00054-2 [pii] 10.1016/j.ab.2009.01.029 (2009)
67. Fraser, A. G. et al. Functional genomic analysis of C. elegans chromosome I by systematic RNA interference. Nature 408, 325-330, doi:10.1038/35042517 (2000).
68. Gonczy, P. et al. Functional genomic analysis of cell division in C. elegans using RNAi of genes on chromosome III. Nature 408, 331-336, doi:10.1038/35042526 (2000)
69. Bartscherer, K., Pelte, N., Ingelfinger, D. & Boutros, M. Secretion of Wnt ligands requires Evi, a conserved transmembrane protein. Cell 125, 523-533, doi:S0092-8674(06)00451-X [pii] 10.1016/j.cell.2006.04.009 (2006)
70. Boutros, M. et al. Genome-wide RNAi analysis of growth and viability in Drosophila cells. Science 303, 832-835, doi:10.1126/science.1091266 303/5659/832 [pii] (2004).
71. Dietzl, G. et al. A genome-wide transgenic RNAi library for conditional gene inactivation in Drosophila. Nature 448, 151-156, doi:nature05954 [pii] 10.1038/nature05954 (2007)
72. Kambris, Z. et al. Drosophila immunity: a large-scale in vivo RNAi screen identifies five serine proteases required for Toll activation. Curr Biol 16, 808-813, doi:S0960-9822(06)01276-0 [pii] 10.1016/j.cub.2006.03.020 (2006)
73. Dickins, R. A. et al. Tissue-specific and reversible RNA interference in transgenic mice. Nat Genet 39, 914-921, doi:ng2045 [pii] 10.1038/ng2045 (2007)
74. Zender, L. et al. An oncogenomics-based in vivo RNAi screen identifies tumor suppressors in liver cancer. Cell 135, 852-864, doi:S0092- 8674(08)01302-0 [pii] 10.1016/j.cell.2008.09.061 (2008)
75. Bric, A. et al. Functional identification of tumor-suppressor genes through an in vivo RNA interference screen in a mouse lymphoma model. Cancer Cell 16, 324-335, doi:S1535-6108(09)00262-1 [pii] 10.1016/j.ccr.2009.08.015 (2009)
76. Xue, W. et al. DLC1 is a chromosome 8p tumor suppressor whose loss promotes hepatocellular carcinoma. Genes Dev 22, 1439-1444, doi:22/11/1439 [pii] 10.1101/gad.1672608 (2008)
77. Chen, Z., Stockton, J., Mathis, D. & Benoist, C. Modeling CTLA4-linked autoimmunity with RNA interference in mice. Proc Natl Acad Sci U S A 103, 16400-16405, doi:0607854103 [pii] 10.1073/pnas.0607854103 (2006)
About the Author
Attila A. Seyhan
After receiving his PhD in Microbial Biochemistry and Biotechnology from Michigan Technological University (MTU), Houghton, MI on a NATO (North Atlantic Treaty Organization) Science Fellowship, Dr. Attila Seyhan moved to Paris, France, for his postdoctoral studies in Professor Bernard Grandchamp’s lab at Xavier Bichat Hospital, University of Paris VII. There he studied regulation of ferritin gene during erythroid differentiation and cell proliferation with Dr. Carole Beaumont on a postdoctoral fellowship from the National Institute of Health and Medical Research of France (INSERM). He did his second postdoctoral fellowship with Professor John Burke at the University of Vermont studying hairpin ribozyme biochemistry and their use as antigene and antiviral agents. He later joined SomaGenics, Inc. to develop self-ligating hairpin ribozymes (RNA LassoTM) for in vitro and in vivo target modulation and as diagnostics and then on RNAi and methods for generating RNAi libraries and their application as antigene and antiviral (HIV, hepatitis C virus, and Semliki Forest virus) therapeutics. He then moved to Dharmacon, Inc. and OpenBiosystems (both part of Thermo Scientific) to develop lentiviral miRNA-based siRNA libraries, lentiviral-shRNAmir libraries, and lentiviral human and mouse miRNA mimic libraries. Thereafter he joined Wyeth Pharmaceutical to lead the RNAi and compound library screening team for target identification and validation in various diseases. He is now a senior leader in Translational Immunology of Inflammation and Immunology at Pfizer Biotherapeutics, leading the genomics biomarker discovery and development team.