High dimensional flow cytometry comes of age
Posted: 3 September 2012 |
Technologies for single cell analysis have recently become prominent in the emerging life science sectors. The last few decades have seen an explosion in advancements in cytometric technologies encompassing instrumentation, probes and data analysis. In particular, flow cytometry has become a well-established and routine method in clinical laboratories. Recent developments have placed high dimensional flow cytometry at the forefront of new applications critical for the pharmaceutical sector and clinical laboratories. The integration of systems biology concepts into biomedical studies has resulted in trans – formative advancements in both the amount and type of information that can be gained to help understand the basis of biocomplexity in health and disease. All aspects of a biological process from biochemical, genetic and cellular to clinical and environmental can be integrated using computational models to derive unique signatures from vast amounts of data. The changes in these signatures, often unique to the individual, can serve to define patho – physiological conditions…
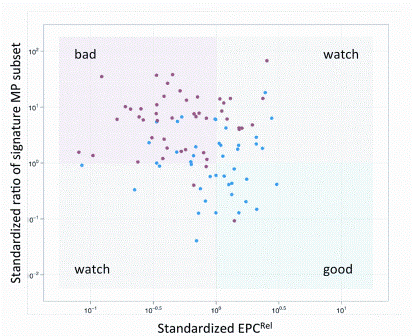
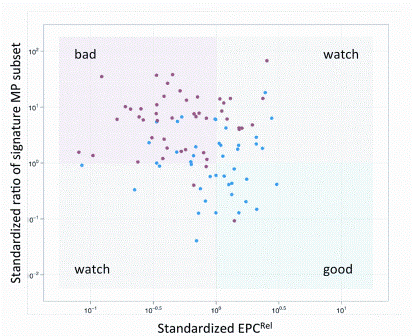
Figure 5: VHP can stratify CVD status. The profile computationally combines EPC and MP measures. In the upper panel, the vertical axis represents a measure of a subpopulation of endothelial microparticles and the horizontal axis a measure of EPCs
Technologies for single cell analysis have recently become prominent in the emerging life science sectors. The last few decades have seen an explosion in advancements in cytometric technologies encompassing instrumentation, probes and data analysis. In particular, flow cytometry has become a well-established and routine method in clinical laboratories. Recent developments have placed high dimensional flow cytometry at the forefront of new applications critical for the pharmaceutical sector and clinical laboratories.
The integration of systems biology concepts into biomedical studies has resulted in trans – formative advancements in both the amount and type of information that can be gained to help understand the basis of biocomplexity in health and disease. All aspects of a biological process from biochemical, genetic and cellular to clinical and environmental can be integrated using computational models to derive unique signatures from vast amounts of data. The changes in these signatures, often unique to the individual, can serve to define patho – physiological conditions1.
Single cell analysis by flow cytometry – the birth of cytomics
Recently, technologies for single cell analysis have become prominent in the emerging life science markets. While genomics, proteomics and metabolomics are familiar to most investigators, and even clinicians, cytomics, facilitated by high dimensional, single cell analysis is becoming a technology of choice for the elucidation of relevant phenotypic patterns that can be directly utilised in the clinical arena (Figure 1).
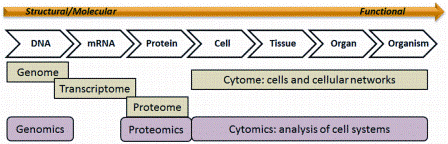
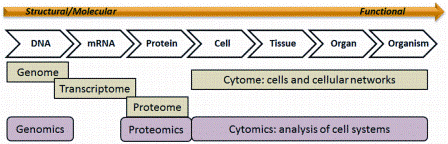
Figure 1: Cytomics – the study of molecular single cell phenotypes resulting from genotype and exposure
A survey produced by HTStec Ltd in June 20122, derived from responses from 202 respondents worldwide, from research to Pharma to clinical laboratories, indicated that most respondents highly rated the specificity that could be provided by singlecell analysis versus the population analysis that is the basis of genomic and proteomic studies. Flow cytometry was rated the preferred technology for determining a specific phenotype within a heterogeneous population and thus enabling rapid identification of relevant biomarkers for both discovery and clinical applications. Flow cytometry enables the detection and enumeration of specific cellular and sub-cellular elements in complex mixture of cells, such as whole peripheral blood. This has led to the birth of cytomic profiling whereby systems biology processes have been coupled with high dimensional flow cytometry to become the basis of a powerful discovery, prognostic, diagnostic and even therapeutic addition to 21st century biomedicine3,4.
Contemporary flow cytometry dates from the early 1970’s when the Herzenberg Laboratory at Stanford University used a laser as an excitation source for fluorescein and demonstrated enhanced sensitivity of detection. By combining sensitive detection with fluidics that allowed for single cell interrogation and subsequent conversion of the signals generated to digital format, the platforms we use today were born, and cell surface, intracellular protein or nucleic acids could be detected by fluorescent probes specific for the target. With this technology, it became possible to characterise multiple targets within complex heterogeneous mixtures and even sort these targets for further studies.
Basic principles
Single cell analysis by flow cytometry can be described as a three step-process: 1) Preparation of the cells, 2) Data acquisition and 3) Data analysis (Figure 2).
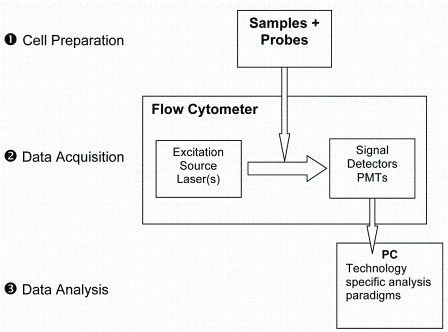
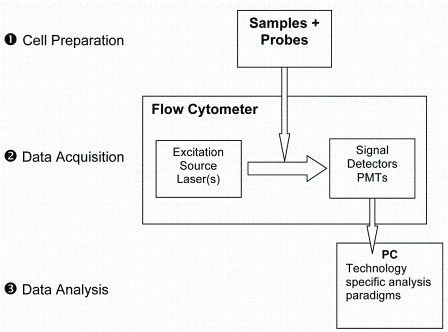
Figure 2: Flow cytometry is a three-step process involving cell preperation, data acquisition and data analysis
Briefly, single cell suspensions are labeled with fluorescent probes specific for cellular components and introduced into the flow cytometry analyser. Using hydrodynamic focusing, the cells flow individually past one or more lasers which excite the fluorescent probes, the emitted light is transmitted through a series of photomultiplier tubes (PMTs) and filters, and collected. The signals are digitised and the data is stored in an individual but correlated manner such that all data present on all cells is acquired. Traditionally, these data files are read into one of several flow cytometry data analysis software programs and a series of two dimensional dot plots are generated to represent the expression of each probe on each cell.
Major improvements in flow cytometry technology
The last few decades have seen an explosion in advancements in cytometric technology encompassing instrumentation, probes and new data handling methods5. Current instruments are configured with multiple, solid state diode lasers that allow sensitive detection of many fluorochromes enabling high dimensional polychromatic flow cytometry3. Parallel to this, there was a realisation that this technology could provide useful clinical information. Simplified benchtop cytometers, with solid state diode lasers, were created and flow cytometers moved from the laboratory to the clinic to become a mainstay in the diagnosis of hematologic malignancies and immunodeficencies. Current clinical platforms are capable of measuring up to 12 simultaneous parameters, at a cost of approximately USD 150,000, making high dimensional cytometry an attainable clinical service.
Coincident with the evolution of instru – mentation has been the development of new fluorescent probes. Once limited to FITC, now new dyes ranging from the ultraviolet to the near infrared allow the multiplexing of probes to provide a more sensitive description of the target. The use of nanoparticles, which can be coupled to proteins, has vastly extended the reach of high dimensional flow cytometry. Cytometry has moved beyond simple phenotyping to functional measures by using probes that can measure cell division, apoptosis, proliferation, protein phosphorylation etc. in unique populations within a heterogeneous mixture, shedding light on very complex interactions.
Although the power of flow cytometry to define complex processes in individual cells is becoming recognised, its potential impact at the systems level has not been appreciated largely because computational approaches have not evolved parallel to the base technology. As the dimensionality of flow cytometric data has increased, due to the evolution of instru – mentation and expansion of available probes, the potential use of this technology for more of a discovery approach is intriguing. The vast amount of data generated has the potential to elucidate complex phenotypes and interactions that could never be identified with conventional technologies, which have hampered high dimensional analyses. Typical analytical approaches depend on sequential gating to reduce complex data sets so that algorithms suitable for two-dimensional analysis can be applied. This approach is very reductionist and often results in only the most apparent discernible patterns. The selection of these gates is often subjective and may result in a biased analysis by uncoupling relationships that may be informative. Several groups, including our own, have approached this dilemma seeking to define new data analysis and data mining approaches. To address this limitation, we developed a new algorithm for high dimensional flow cytometric data analysis, Cytometric Fingerprinting (CF)6. Computational methods such as CF air to increase objectivity, speed and completeness in analysis of flow cytometric data. Ungated flow cytometric data is collected and directly analysed using a computationally-based unbiased approach. A model of a dataset is created by binning some or all of the instances in the data set. Then the model is applied to each instance in the data set. A fingerprint of each instance is generated. In Figure 3, the process is illustrated.
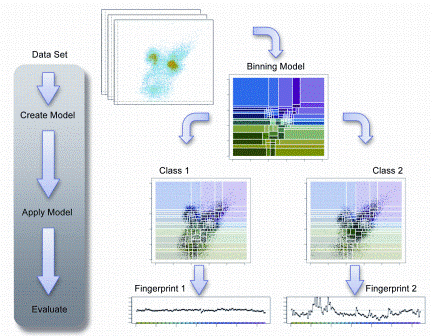
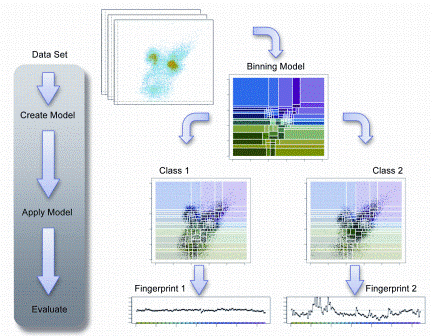
Figure 3: Cytometric Fingerprinting can detect population differences. In this example, two classes are considered: Class 1 is healthy, Class 2 is diseased. The left example, a member of Class 1 has a distribution similar to the model distribution, so the fingerprint is flat. The right example has a distribution quite different from the model, thus its fingerprint exhibits large excursions indicating differences from the healthy. The associated phenotypes causing this deviation can be identified
Computational approaches, such as CF, will enable the automation of flow cytometric data analyses, eliminating tedious and subjective manual gating, leading to the current mantra of ‘don’t gate it, model it’ (C. Bruce Bagwell, MD, PhD, personal communication, 35th Annual Course in Flow Cytometry, Bowdoin College, 2012). As with all computational data analysis methods, strict adherence to standards and established protocols are required, but subjectivity is removed and the process is not hampered by increasing dimensionality. Fingerprints can be archived into a large-scale database that will facilitate data mining and increase sensitivity for elucidating biologic effects that may reveal new disease associations or mechanisms. Major improvements of flow cytometry analysis are summarised in Table 1.
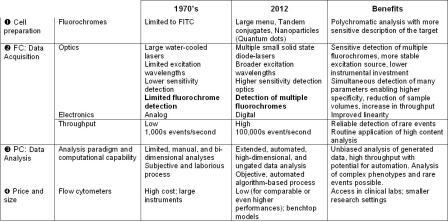
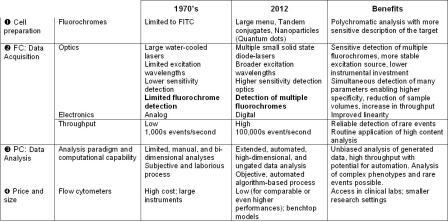
Table 1: Technical evolution of flow cytometry
Flow cytometry: A wide and expanding range of applications
All these developments have placed high dimensional flow cytometry at the forefront of new applications critical for research, discovery, diagnostic and prognostic applications.
Flow cytometry in the pharmaceutical sector
Flow cytometry is increasingly used for discovery and identification of molecular hotspots. Disease inducing molecular pathways are explored by a retrograde molecular analysis strategy (molecular reverse engineering) of molecular phenotype differentials at the cell system level (Figure 4). The dimensionality and high content nature of the technology can rapidly translate to relevant target identification.
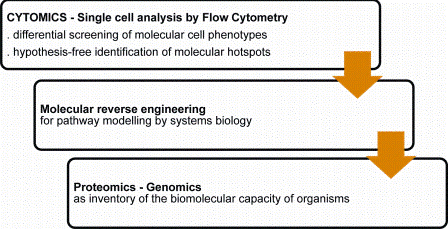
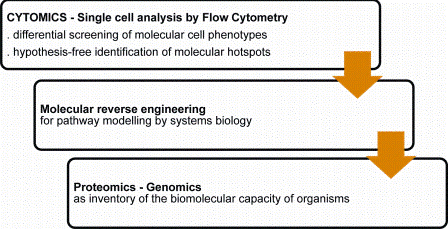
Figure 4: Flow cytometry and discovery
Flow cytometry in the clinical arena – personalised medicine
Sensitive measures of rare cells and cell-based functional assays are expanding the applications of high dimensional translational and clinical flow cytometry. The development of applications such as the detection of the phosphorylation state of kinases critical for cell function now allow the ‘barcoding’ of cellular responses and identification of potential therapeutic targets7. Investigation of cytokine networks of individual cells within a population can demonstrate unique patterns that can reflect functional defects in a disease process8. Cell proliferation assays can allow the dissection of growth and interaction characteristics of multiple populations9. Specific cell populations can actually be sorted and used therapeutically in a variety of settings. Personalised medicine and companion diagnostics approach take into account each patient’s unique physiology and enables to identify the disease, select the appropriate treatment and monitor its efficacy. Once only used for hematology/oncology and infectious disease, cytometric profiling, enabled by new computational approaches, is extending into new disciplines such as cardiology. Cardiologists struggle to identify patients for whom specific therapies will be effective as many of the most widely prescribed classes of drugs, such as ACE inhibitors, beta-blockers, and statins are often marginally effective10. Clinical studies have shown that individuals with low or intermediate risk according to Framingham criteria can nevertheless go on to develop symptomatic CVD11. Twenty per cent of all vascular events occur in the absence of conventional risk factors (hypertension, tobacco-smoking, diabetes, and hyper – lipidemia)12, and 50 per cent of them occur in the absence of significant lipid abnormalities13. Our group, in conjunction with CytoVas, LLC, has developed the first cytomic assay, the Vascular Health profile (VHP), aimed at measuring the state of vascular health in patients. This simple blood test measures components of vasculogenesis (endothelial progenitor cells) and of vascular destruction (micro – particles) and using cytometric fingerprinting, derives a signature profile of the status of the patient’s vascular health that can be used to monitor therapy and potentially to stratify at risk groups to facilitate medical management (Figure 5). This assay has recently been validated in a study comparing normal controls to diabetic patients14,15.
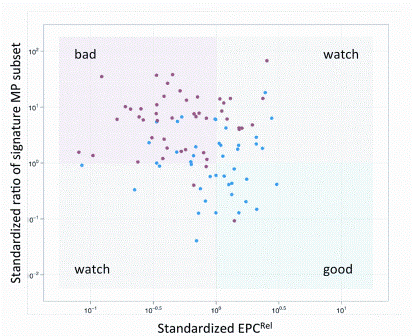
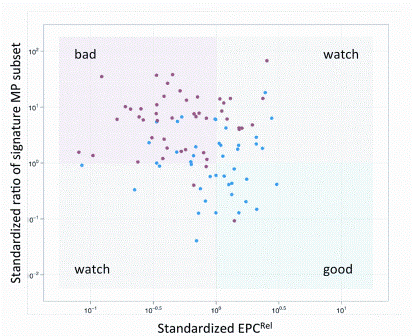
Figure 5: VHP can stratify CVD status. The profile computationally combines EPC and MP measures. In the upper panel, the vertical axis represents a measure of a subpopulation of endothelial microparticles and the horizontal axis a measure of EPCs
Conclusion
High dimensional flow cytometry has indeed come of age and is rapidly taking its place as a technology of choice for discovery, diagnostic and therapeutic applications beyond hemat – ology and immunology. The combination of established platform with high dimensional and high throughput capabilities positions cellbased analyses as the high content technology of choice, edging out proteomics and genomics, for ‘payback’ for the identification of biomarkers that can be immediately applied. As we begin the 21st Century, we find that not only have flow cytometers evolved, but we are beginning to see ‘technology merges’. The recent development of mass cytometry combines the power of mass spectroscopy with single cell flow cytometry to provide superior resolution of over 30 target proteins simultaneously3. Cytomics has been combined with genomics to use the power of sensitive cell surface phenotyping with single cell gene expression profiling16. With the evolution of computational methods that approach data in an agnostic manner, advancements in platforms and probes and the merging of other high dimensional technologies, cytomic profiling is poised to become a major player in personalised medicine.
References
1. Loscalzo J, Kohane I, Barabasi AL. Human disease classification in the postgenomic era: a complex systems approach to human pathobiology. Mol Syst Biol. 2007;3:124
2. Single Cell Technologies Trends 2012. HTCtec Ltd Report, June 2012
3. Bendal SC, Nolan GP, Roederer M, Chattopadhyay PK. A deep profiler’s guide to cytometry. Trends in Immunology. July 2012: 33(7): 323-332.
4. Nolan JP, Yang L. the flow of cytometry into systems biology. Brief. Funct. Genomics Proteomics 2007; 71(6): 81-90
5. Smith PJ, Edward R, Ervington RJ. (2011). Recent advances in flow cytometry: Platforms, tools, and challenges for data analysis. In Litwin V & Marder P, Flow Cytometry in Drug Discovery and Development (pp. 23-54). Hoboken, USA: John Wiley and Sons.
6. Rogers WT, Moser AR, Holyst HA, et al. Cytometric fingerprinting: quantitative characterization of multivariate distributions. Cytometry A. May 2008;73(5):430-441
7. Krutzik PO, Nolan GP. Fluorescent cell barcoding in flow cytometry allows high-throughput drug screening and signaling profiling. Nature Methods 2006; 3 (5): 361-368
8. Moore JS, Zaki MH. Clinical Cytokine Network Cytometry. Clinics Lab Med 2001; 21: 795
9. Bantly A.D., Gray B.D., Breslin E., Weinstein E.G., Muirhead K.A., Ohlsson-Wilhelm B.M., Moore J. CellVue Claret, a new far-red dye, facilitates, polychromatic assessment of immune cell proliferation. Immunol Investigations 2007; 36:581-605
10. Aspinall M, Hamermesh R. Realizing the promise of personalized medicine. Harvard Business Review. October 2007; 109-117
11. Castelli WP. Epidemiology of coronary heart disease: the Framingham study. Am J Med. Feb 27 1984;76(2A):4-12
12. Khot U, Khot M, Bajzer C, et al. Prevalence of conventional risk factors in patients with coronary heart disease. JAMA. Aug. 2003; 898-904
13. Preface. Cardiovascular disease risk assessment: lessons from observational studies over 60 years. XV International Symposium on Atherosclerosis. June 2009
14. Curtis AM, Zhang L, Medenilla E, et al. Relationship of microparticles to progenitor cells as a measure of vascular health in a diabetic population. Cytometry Sep 2010;78(5):329-337
15. Kurtzman N, Zhang L, French B., et al. Cytomic vascular health profile evaluation of atherosclerosis in diabetic patients. 2012. Submitted
16. Narsinh, KH, Sun N, Sanchez-Freiere V, Lee AS, Almeida P, Hu S, Jan T, Wilson KD, Leong D, Rosenberg J, Yao M, Robbins, RC, Wu, J. Single cell transcriptional profiling reveals heterogeneity of of human induced pluripotent stem cells. J Clin Invest 2011; 121(3): 1217-1221
About the authors
Jonni Moore, PhD, is a Professor of Pathology and Laboratory Medicine, Director, Clinical Flow Cytometry, HUP, Director, Abramson Cancer Center Flow Cytometry and Cell Sorting Shared Resource at the Perelman School of Medicine of the University of Pennsylvania. Receiving her PhD in immunology from Thomas Jefferson University, Philadelphia, Dr. Jonni Moore has been on the faculty at the University of Pennsylvania for 28 years, where she has established an international reputation in clinical and research flow cytometry. She is also co-founder of CytoVas, LLC, an early stage in vitro diagnostics company.
Dr. Pascal Yvon has 25 years of global experience in the Diagnostics and Life Sciences industries working with startups to large companies. He holds a Doctorate in Pharmacy from the Pharmacy University, Paris and an MBA from Rutgers University, NJ. He is a member of BioNJ where he co-chairs the Diagnostics and Personalised Medicine Committee.