Implementing the FAIR data principles is now a critical endeavour
Posted: 29 June 2021 | Ian Harrow (The Pistoia Alliance), Thomas Liener (The Pistoia Alliance) | No comments yet
With companies acknowledging the value of their data, it becomes increasingly important that data integrity be assured – and data stewardship implemented. In this article, Ian Harrow and Thomas Liener, Consultant Project Managers at The Pistoia Alliance explain the importance of the FAIR (Findable, Accessible, Interoperable, Reusable) data principles, factors currently limiting their implementation and the potential benefits should these challenges be overcome.
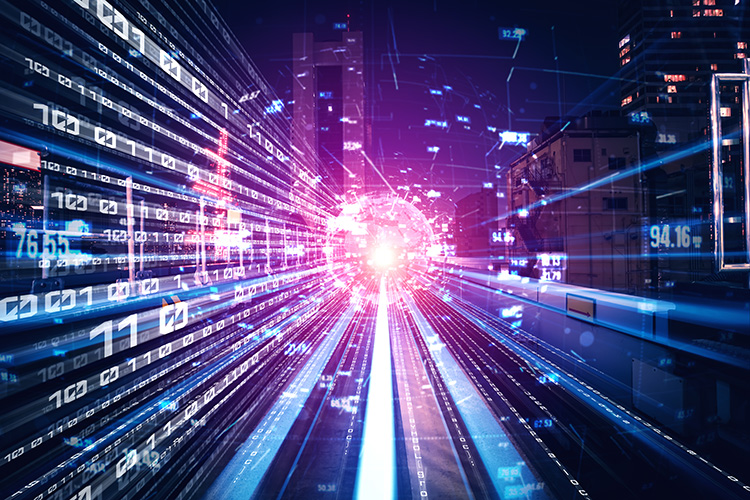
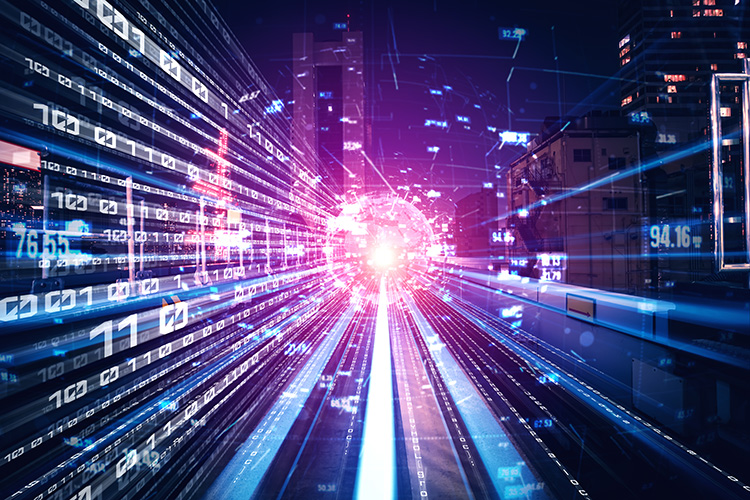
TODAY, MANY LIFE SCIENCE companies have shifted to essentially become big data enterprises. They have come to understand the value of their data as a powerful corporate asset. However, the industry also has one of the biggest varieties of data sources from which to extract insight, continuously being generated in large volumes. This results in a ‘data deluge’. Much of the data used by life science companies are siloed in widely differing formats and locations – making it difficult to retrieve, query and share – rendering it essentially unusable.
Organisations understand this needs to change, that data integrity must be ensured and that principles of data stewardship need to be implemented with clear practical guidance. Especially as the industry shifts towards greater adoption of artificial intelligence (AI) and automation, without robust data management strategies for the entire lifecycle of data these efforts will fail to deliver. The EU revealed that this lack of FAIR (Findable, Accessible, Interoperable, Reusable) research data and associated metadata is costing the European economy at least €10.2bn every year.1
Furthermore, by drawing a rough parallel with the European open data economy, it concluded that the downstream inefficiencies arising from not implementing FAIR could account for a further €16bn in losses annually.
What are the FAIR guiding principles?
The FAIR guiding principles contribute to better scientific R&D of new medical therapies for the efficacious and safe treatment of disease and health conditions. The principles place “…specific emphasis on enhancing the ability of machines to automatically find and use the data, in addition to supporting its reuse by individuals”.2 They were published in 2016 with the goal of emphasising machine-actionability (the idea that computational systems can find, access, interoperate and reuse data with minimal human intervention) as humans increasingly need computational support to manage the vast volume and complexity of data generated daily.
FAIR can enable future innovation
Currently, many data are not standardised or easily retrievable. This makes it difficult or, in some cases, impossible to use for algorithms without scientists spending extended amounts of time wrangling data. However, the Lab of The Future (LoTF) will be digitally driven3 and will rely on high levels of automation. Both AI and machine learning (ML) systems will play a key role, both of which will also need quality data to ‘learn’ from. When AI is used to aid decision‑making regarding health, then harmonised, comparable and trusted data is paramount. In the LoTF, clear data management is required to continue to modernise lab environments and help the industry continue to make breakthroughs. FAIR implementation strategies must also, for example, provide for technology and format obsolescence to ensure data remains reusable.
What is holding back implementation?
For most life science companies, there are still many barriers to overcome during the implementation of FAIR. The biggest is often the financial and time investment required to begin the change process. Senior leadership teams need to be convinced that FAIR implementation will lead to long-term return on investment (ROI). As discussed above, FAIR can act as an enabler. AI thrives on accurate, reliable and machine-readable data to function; by making data machine-readable and AI-ready, it can usher in a new level of efficiency and effectiveness in biopharma R&D. FAIR can also provide ROI, as it greatly reduces the need for data wrangling and enhances data integration, meaning researchers and scientists can spend more time on innovative activities.
Workplace culture also needs to change. There must be a shift from the traditional approach of “my lab, my data” to a data-centric culture. It needs to be company-wide data at a minimum – and a clear strategy on how to store and reference data and metadata is needed. Often today, big breakthroughs are made through cross-company and cross-discipline collaboration and through sharing pre-competitive data. Take the collaborations and data sharing seen in the last 18 months to tackle COVID-19, which has resulted in the creation of life-saving treatments, diagnostics and vaccines in record time. If strong data management infrastructure and FAIR principles were adopted industry wide, we could see a repeat of such breakthrough efforts in other therapeutic areas.
However, the life science industry often has to protect sensitive data, especially given the highly regulated nature of the industry, which has made many hesitant to change their perspective to a data-centric culture. We need to educate employees and leaders on the value of FAIR implementation. This should be done through industry-wide training, development and education programmes. The implementation of FAIR principles requires an incentivised cultural change underpinned by clear and frequent communications. Awareness of the needs and challenges of all stakeholders in the early planning phase of FAIR implementation will lead to better solutions and better acceptance. The designation of data stewards in each company, charged with ensuring that data management and governance is embedded and maintained within the organisation, can catalyse this change.
Realising productivity gains
Adopting FAIR implementation can unlock the long-term potential of data for future research, improve collaboration, enable scientific queries to be answered more rapidly and support alignment with compliance requirements. All these factors can help to create productivity gains, which are now essential in biopharma as the time and cost of creating new therapies continues to skyrocket. In the same vein, the implementation of a FAIR mindset can reduce the need for work to be repeated, as it enables more data to be used for secondary purposes. In addition, the implementation of FAIR principles can also aid the drive towards precision medicine, by enabling the development of more segmented or personalised real-world data to match best treatment to relevant patient cohorts.
A crucial aspect of implementing the FAIR guiding principles is the measurement of the level of FAIRness. This is achieved using various metrics or maturity indicators that quantify FAIRness to seek improvements in an iterative manner to optimal levels, determined by business needs.
The Pistoia Alliance facilitates the implementation of the FAIR guiding principles with its FAIR Implementation Project.4 It shares best practices through the ongoing development of the FAIR Toolkit5 and through the development of a guide for the implementation of FAIR for Clinical (FAIR4Clin) trial and healthcare data. We welcome involvement from any stakeholder who would benefit from FAIR implementation for clinical data and those who are keen to share their knowledge and learnings.
About the authors
References
- Cost-benefit analysis for FAIR research data: cost of not having FAIR research data. [Internet]. Op.europa.eu. 2019 [cited 27 May 2021]. Available from: https://op.europa.eu/en/publication-detail/-/publication/d375368c-1a0a-11e9…
- Wilkinson M, Dumontier M, Aalbersberg I, et al. The FAIR Guiding Principles for scientific data management and stewardship. Scientific Data. 2016;3(1). Available from: nature.com/articles/sdata201618
- Oakley Y, Proudlock D, et al. Lab of the Future Archives – Pistoia Alliance [Internet]. Pistoia Alliance. 2021 [cited 10 June 2021]. Available from: https://www.pistoiaalliance.org/tag/lab-of-the-future/
- Harrow I. The FAIR Toolkit and FAIR Life Science Industry Implementation Project [Internet]. Pistoia Alliance. 2021 [cited 10 June 2021]. Available from: https://www.pistoiaalliance.org/projects/…
- FAIR Toolkit – The FAIR Toolkit by Pistoia Alliance – A FAIR Toolkit for Life Science Industry [Internet]. Fairtoolkit.pistoiaalliance.org. 2021 [cited 10 June 2021]. Available from: https://fairtoolkit.pistoiaalliance.org/
- Pistoia Alliance | Collaborate to Innovate Life Science R&D [Internet]. Pistoia Alliance. 2021 [cited 10 June 2021]. Available from: https://www.pistoiaalliance.org/
Issue
Related topics
Artificial Intelligence, Big Data, Data integrity, Informatics, Internet of Medical Things (IoMT), Lab Automation, Technology