Biomarker discovery and validation in clinical proteomics
Posted: 23 May 2007 | | No comments yet
Until recently the use of proteomics in the biomedical arena has included programmes aimed at the elucidation of cellular responses to extracellular stimuli by known and potential drugs. It has been anticipated that these will lead to the elucidation of the basic mechanisms of cellular responses, potential identification of new drug targets and discovery of the mechanism of action of drugs both NCE’s and those currently in development.
Until recently the use of proteomics in the biomedical arena has included programmes aimed at the elucidation of cellular responses to extracellular stimuli by known and potential drugs. It has been anticipated that these will lead to the elucidation of the basic mechanisms of cellular responses, potential identification of new drug targets and discovery of the mechanism of action of drugs both NCE’s and those currently in development.
Until recently the use of proteomics in the biomedical arena has included programmes aimed at the elucidation of cellular responses to extracellular stimuli by known and potential drugs. It has been anticipated that these will lead to the elucidation of the basic mechanisms of cellular responses, potential identification of new drug targets and discovery of the mechanism of action of drugs both NCE’s and those currently in development.
In addition, the use of proteomics to investigate toxic responses to drugs (toxicoproteomics) in pre-clinical and clinical studies has gained increasing importance, particularly when such data is analysed in the context of more traditional toxic responses. Notably, many of these studies are now undertaken in the context of multi-team groups or a consortia of researchers from different disciplines – including proteomics. An interesting example of such a consortium is the EU and commercially funded ‘PredTox’ predictive toxicology programme being undertaken by a range of companies and Universities; the programme forms part of the ‘Innovative Medicines for Europe’ initiative. There are important lessons to be learnt from the operational management of such consortia (to be discussed further on within the article). The PredTox programme is exemplary of the growing interest in the use of ‘omics technologies, including proteomics, to identify ‘biomarkers’, as well as a vibrant debate on the relative importance of biomarkers for revenue generation in the pharma sector. Here, the term ‘biomarkers’ will be defined in the context of proteomics, an historical overview of the evolution of proteomics technologies and their continuing development will be given, and some recent biomarker discovery projects will be outlined with the use of selected examples. To conclude, some of the lessons learnt and challenges that remain in biomarker discovery, validation and implementation will be summarised.
What is a biomarker?
It is interesting to try to establish ‘de novo’ the definition of a biomarker and the aims of current activity in this arena. Even a cursory ‘dip’ into web search engines produces hundreds of thousands of results for the term biomarker, and many of these give ‘definitions’. For example, the Oxford English Dictionary defines a biomarker as; “A substance used as an indicator of the presence of material of biological origin, of a specific organism, or a physiological condition or process; spec. a diagnostic indicator of (predisposition to) a medical condition.” “Substance”, “indicator” and “medical condition” could be considered as the most important words within this definition and it these connotations that formed the succinct (and elegant) definition used by Sir Richard Doll who suggested that a biomarker is the “Linkage between ‘measurable’ event(s) and disease”. Sir Richard published a landmark paper in 1954 showing the association between smoking and lung cancer. He then continued follow up studies for a further 50 years [see ‘The mortality of doctors in relation to their smoking habits: a preliminary report’, which was reprinted from Br Med J 1954:ii;1451-5 in 20041]. It is notable that biomarker(s) can and should be regarded as more than just a single measurable event and so might represent a combination of parameters; in the present context it is important to emphasise that the events may not all be changes in protein expression. The search for a single protein biomarker of required sensitivity and specificity for clinical use is now almost certainly an unrealistic objective and, as noted later; methods to integrate data from measurement of ‘events’ continue to require considerable refinement and improvement. In particular, there is a growing appreciation that imaging data and molecular data (perhaps including mass spectrometry based molecular imaging) could be integrated to great effect. There are a number of arenas including analysis of individual disease areas in which biomarker discovery makes an impact, these include: protein expression signatures as biomarkers, biomarkers for drug safety assessment, biomarkers for NCE characterization, biomarker assay development and validation and, of course, the use of biomarkers in go versus no-go decisions in drug development. It should be noted that the diversity of current proteomics methods for the discovery of biomarkers is not at present matched by the availability of protein assays that are used in clinical practise. The gold standard assay is the ELISA, however, the length of time in which this will remain the case is a subject of interesting debate. Other platforms include multiplexed immuno-assays on solid surfaces (including microarrays) and bead based assays. More exciting developments in mass spectrometry based imaging and quantitative assays of peptide/protein expression are underway, although in reality they are a long way from gaining widespread acceptance/use in research and (more importantly) clinical applications.
The evolution of proteomics for biomarker discovery
The term “proteome” was first coined in 1995 by Marc Wilkins and his colleagues2 and was defined as the “protein complement of a genome”. However, the term “proteomics” has now come to be regarded as the global analysis of the proteins expressed in normal biological processes (e.g. development, cell cycle, ageing, apoptosis), in response to the environment (drugs, toxic agents) and in disease states. In its use to measure the expression (including localisation, turnover and modification) of proteins it is regarded as providing the opportunity for protein-based “discovery”. The results of proteomics studies can provide important new insights into the molecular basis of biological processes in both health and disease, identifying new biomarkers that can be exploited as diagnostic/prognostic reagents and/or as therapeutic targets. In general proteomics biomarker discovery experiments follow a relatively straightforward workflow of:
- Sample acquisition and preparation. The importance of this initial step in the discovery process cannot be over-emphasized. There are many pre-existing biobanks (collated collections of samples) but in reality the samples contained within them were often obtained from different poorly co-ordinated studies, using different protocols (not following standard operating procedures), and are not age-matched or otherwise suitably matched for the objectives of the investigation. Samples can include biological fluids, tissues – including biopsies (disease vs. normal), cells and sub-cellular components (membranes / mitochondria/ nuclei etc.);
- Protein/peptide separation. This is achieved by a now bewildering array and combination of approaches including one and two-dimensional gel electrophoresis and liquid chromatography based separations;
- Protein/peptide detection and protein/peptide identification/quantification. The techniques used include covalent modification of proteins with fluorophores prior to separation (DIGE), conventional dye detection of proteins with mass spectrometry (MALDI and ES) for identification and quantification. These MS techniques convert biomolecules which are polar and charged into gas-phase ion, before subsequently being analyzed. There are many diverse MS analysers available in the proteomic research field including: time of flight (TOF), quadrupole, ion trap, and the fourier transform-ion cyclotron resonance (FT-ICR).
Despite numerous advances in b) and c) the separation of intact proteins by high-resolution two-dimensional gel electrophoresis (2-DE) remains one of the most popular of the “discovery” proteomics workflows3. Following separation and sensitive detection of the separated proteins; often using fluorescence based methods, the 2-D protein spot patterns are subjected to differential semi-quantitative analysis using one of a range of commercially available computer packages. In brief, this process typically involves: image digitization, image filtering, background subtraction, spot detection (e.g. by Gaussian fitting, Laplacian and second derivatives, edge detection), landmarking, matching, and analysis (quantitative, qualitative, statistical). This process continues to be a major bottleneck in the analysis of proteomic data from 2-D gels as intrinsic variability in the shape and distibution of the protein ‘features’ necessitates extensive manual interaction with the software, rendering it at best a semi-automated process4. The combination of fluorescent dye labeling (DIGE) with the ‘Progenesis’ software package (Non-Linear Dynamics) is obtaining increasing acceptance and adoption as a currently effective platform.
In alternative proteomics workflows, samples are initially digested to peptides (usually with trypsin) and the peptides then analysed by tandem mass spectrometry (MS/MS), normally coupled either on or off-line to one or liquid chromatography steps. This approach, often termed “shotgun” proteomics or, a version of it; MudPIT5, is very powerful in identifying large numbers of proteins in a complex mixture. However, this approach is inherently non-quantitative and not currently amenable to large numbers of samples that might be required for a statistically valid method for biomarker discovery. To overcome the problem of quantitaties, peptide based approaches can be combined with stable isotope labeling strategies, such as SILAC, ICAT, or iTRAQ6 In this combined workflow, one of each pair of samples is labeled, either at the level of whole cells (e.g. SILAC), intact proteins (e.g. ICAT) or tryptic peptides (e.g. iTRAQ), with a stable isotope labeled form of the particular reagent. The two (or four in the case of iTRAQ) samples are then combined (multiplexed) and processed further together. The resulting peptides coded in this way then appear during MS as clusters of ions separated by the mass difference in the coding agents or by the release of the mass tag (iTRAQ). The ratio of the ion intensities of these clusters or the mass tag is then a measure of the relative abundance in the samples of the protein from which the particular peptides was generated. The analysis of the complex spectra to generate this relative quantitative information is challenging and there is considerable scope for the development of new and improved approaches. More recently there has been growing interest in the use of ‘signature’ peptides for quantitative and sensitive measurement of protein expression7, 8, 9.
Thus, as in transcriptomics there are a bewildering array of potential platforms, and choosing the most appropriate for the task in hand can be difficult. It is often a decision that has to combine a range of factors which vary during the implementation of the discovery process. As a general observation, it seems unfortunate that there appears to be such intense competition to promote an exclusive paramount technology (which necessarily emphasises the shortcomings of the alternatives); with the consequence that opportunities for mutually compatible and even synergistic combinations platforms of over-lapping capabilities may be being unnecessarily overlooked.
Application of proteomics for biomarker discovery
In our own research portfolio we have and continue to undertake biomarker discovery in diverse areas including pancreatic cancer, prostate cancer and predictive toxicology; including the development of a quantitative ‘assay’ for the simultaneous measurement of the expression of cytochrome P450 isoforms. In pancreatic cancer we have effectively applied careful sample acquisition and preparation (using laser capture microdissection) with 2-DE and MS to identify potential biomarkers of the disease. One such biomarker, S100A6 protein, has been ‘validated’ by immuno histochemistry of tissue microarrays and ‘linkage’ between the expression of high levels of S100A6 in the nuclei of ductal cells of the pancreas as a prognostic indicator of disease outcome10, 11. In prostate cancer, as part of an Irish Cancer Fund supported consortium of hospitals and research institutes, proteins and metabolites in semen and urine12, 13 are being sought to identify more effective biomarkers than the prostate specific antigen (PSA) which are currently used. PSA is one example of the many current diagnostic markers that have acknowledged no effective replacement (supplement). Alongside these studies we (in common with many others) are undertaking proteomic biomarker analysis of patient response to treatment, in our case detailing the response to combined chemo and radiotherapy treatment of prostate cancer. This has highlighted the need to implement a robust process for sample accrual (during patient follow-up) and the necessary long-term nature of such studies. For us, these studies have highlighted some of the conclusions that may be drawn from current approaches and some of the questions that remain for biomarker discovery (Figure 1).
Teamwork, high quality samples, flexible application of discovery technologies and the ability to integrate data have proven to be extremely important. Remaining questions include the identification of current bottlenecks in the technologies and their exploitation, as well as ways in which the data generated can be implemented into a clinically relevant process of disease/patient assessment and monitoring (Figure 1). The latter represents a major undertaking that may need to be co-ordinated with other innovative approaches for patient assessment and monitoring to assist clinical decision making. It has been noted that the increasingly large and detailed array of information must be presented in a form that can be processed by the human user14; this in itself will be a major undertaking.
Conclusion
What is likely to make the application of proteomics to biomarker discovery successful? The building of effective teams is one of the most challenging aspects; industry has a theoretical head start in that it is assumed that everyone working for the company has the companies’ success as a shared goal, whilst in academia there may be a greater element of self-directed motivation. In any environment flexibility, motivation and generosity in the sharing of success is essential. It is also reasonably obvious that an ideal discovery scenario would be one that has unlimited resources: from sample availability, to instrumentation and software as well as a flexible ability to ‘follow through’ on discoveries in an appropriate manner. There are so many potential avenues of opportunity afforded by proteomics based discoveries that future ‘follow through’ could require the implementation of new experimental approaches not already in the research teams ‘armoury’. An all embracing, watchful and objectively critical appraisal of new and unfamiliar technologies is likely to be essential for competitive success in the demanding but potentially profitable arena of biomarkers.
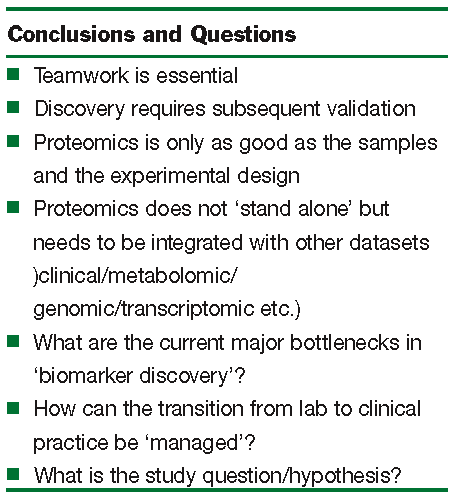
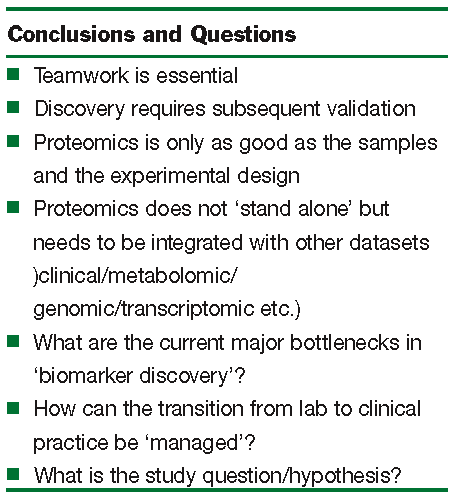
Figure 1: Observations from proteomic biomarker discovery programmes. Key conclusions and remaining questions. Notably, the precise definition of the research and clinical question or hypothesis under investigation needs to be cleary articulated and shared by the team of researchers, nurses, clinicians and informaticians involved.
References
- Doll, RA and Hill, AB. (2004) British Medical Journal, 328, 1529-1533.
- Wasinger, VC, Cordwell, SJ, Cerpa-Poljak, A, Yan, X, Gooley, AA, Wilkins, MR, Duncan, MW, Harris, R, Williams, KL, and Humphery-Smith, I. (1995). Progress with gene-product mapping of the Mollicutes: Mycoplasma genitalium. Electrophoresis, 16, 1090-1094.
- Gorg A, Weiss W, Dunn MJ. (2004) Current two-dimensional electrophoresis technology for proteomics. Proteomics 4, 3665-3685.
- Dowsey AW, Dunn MJ, Yang GZ. (2003) The role of bioinformatics in two-dimensional gel electrophoresis. Proteomics 3, 1567-1596.
- Wolters DA, Washburn MP, Yates JR 3rd. (2001) An automated multidimensional protein identification technology for shotgun proteomics. Anal Chem. 73, 5683-5690.
- Julka S, Regnier F. (2004) Quantification in proteomics through stable isotope coding: a review. J Proteome Res. 3, 350-363.
- Jenkins RE, Kitteringham NR, Hunter CL, Webb S, Hunt TJ, Elsby R, Watson RB, Williams D, Pennington SR, Park BK. (2006) Relative and absolute quantitative expression profiling of cytochromes P450 using isotope-coded affinity tags. Proteomics 6,1934-1947.
- Aebersold, R. (2007) Quantitative proteomics and systems biology FASEB J., 21, A212
- Barnidge, DR, Goodmanson, MK, Klee, GG and Muddiman, DC (2004) Absolute Quantification of the Model Biomarker Prostate-Specific Antigen in Serum by LC MS/MS Using Protein Cleavage and Isotope Dilution Mass Spectrometry J. Proteome Res., 3, 644-652.
- Shekouh AR, Thompson CC, Prime W, Campbell F, Hamlett J, Herrington CS, Lemoine NR, Crnogorac-Jurcevic T, Buechler MW, Friess H, Neoptolemos JP, Pennington SR, Costello E. (2003) Application of laser capture microdissection combined with two-dimensional electrophoresis for the discovery of differentially regulated proteins in pancreatic ductal adenocarcinoma. Proteomics 3, 1988-2001.
- Vimalachandran D, Greenhalf W, Thompson C, et al. (2005) High nuclear S100A6 (Calcyclin) is significantly associated with poor survival in pancreatic cancer patients. Cancer Res 65:3218-3125.
- Downes, MR, Byrne, JC, Dunn, MJ, Fitzpatrick, JM, Watson, RWG and Pennington SR (2006) Application of proteomic strategies to the identification of urinary biomarkers for prostate cancer: A review. Biomarkers, 11, 406 – 416.
- Tyers, M. and Mann, M. (2003) Nature 422, 193-197.