Using translational pharmacology biomarkers to drive earlier decision making
Posted: 19 April 2011 |
The current high rate of attrition during drug development is unsustainable. An increasing amount of the cost of developing a new drug is made up of the investment in molecules that fail at some point during the process and the later that failure occurs, the more costly it will be. Recent surveys suggest that the attrition rate in the pharmaceutical industry is now more than 90 per cent from the first in human study to launch. Reducing attrition is now one of the key challenges for the pharmaceutical industry, but before exploring potential ways forward, it is necessary to understand what drives the attrition and causes drug candidates to fail during the development process.
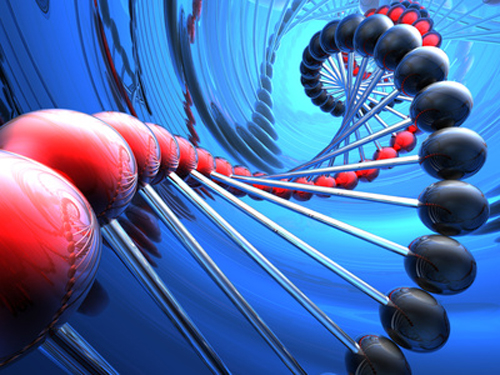
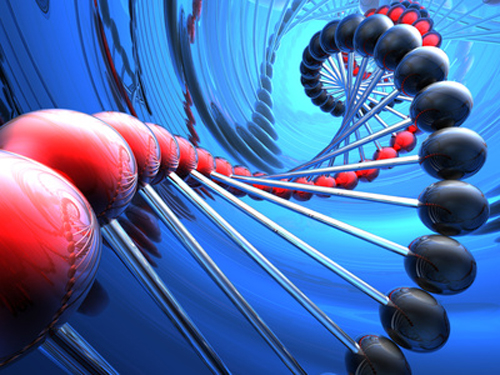
The current high rate of attrition during drug development is unsustainable. An increasing amount of the cost of developing a new drug is made up of the investment in molecules that fail at some point during the process and the later that failure occurs, the more costly it will be. Recent surveys suggest that the attrition rate in the pharmaceutical industry is now more than 90 per cent from the first in human study to launch1-4. Reducing attrition is now one of the key challenges for the pharmaceutical industry, but before exploring potential ways forward, it is necessary to understand what drives the attrition and causes drug candidates to fail during the development process.
Most candidates fail for some basic reasons e.g. a) the original hypothesis and target is wrong; b) the target mechanism does not translate to human due to a different molecular target distribution or the pharmacology between species is not comparable; c) the molecule fails to reach the desired target in sufficient amounts to be efficacious; d) the molecule cannot be formulated in an acceptable way; e) unexpected clinical safety issues; f) changes in the commercial value of target hypothesis. However, the contribution of these different reasons for the attrition of drug development candidates will naturally change over time as risks are identified and addressed. This is exemplified with the introduction of the improved preclinical screening of drug metabolism and the significant reduction in pharmacokinetic reasons for drug candidate failure that this screening has entailed5.
Across the industry, a number of strategies are being implemented to improve the development success of drug candidates. The strategies can be divided into ‘improved target validation’, ‘improved drug candidates’ and ‘increased early attrition rate’2.
The main focus of this review will be how translational pharmacology biomarkers can be used in the strategy to increase the early attrition rate and thus improve the success rate at later stages. The Biomarkers Definitions Working Group6 defined a biological marker as something which is “a characteristic that is measured and evaluated as an indicator of normal biological processes, pathogenic processes or pharmacological responses to a therapeutic intervention.” The generic definition of a biomarker has had the effect that the term biomarker has been widely used and it is therefore imperative that the definition of a biomarker is clearly defined. The generic definition encompasses both disease-related (a biological effect of a drug that is predictive of efficacy in the target disease either in patients or in a human model of the disease) and nondisease related, which is linked to target engagement (a biological effect that indicates that the drug is having the expected pharmacology for its target receptor/enzyme etc). The discussions in this review will focus on the latter type of biomarkers, which has also been called efficacy, target or pharmacology biomarkers.
The goal of this type of biomarker is to confirm the drug pharmacology in human studies and explore dose predictions so that the key programme hypothesis can be evaluated as early as possibly, ideally already in healthy volunteers. For any drug development programme, it is imperative that the target mechanism is tested in the clinic so that it is possible to confidently walk away from a mechanism that does not work. Historically, as discussed above, this has not been the case, resulting in continued high level investment and subsequent failure at later stages. How do you then identify the development molecules that are more likely to succeed, or in other words, ‘how do you spot the winners’? Firstly, the molecule needs to achieve exposure at the site of action and secondly the molecule needs to engage and bind to the pharmacological target. Finally, the binding to the target should lead to expression of pharmacology and added confidence and value would be provided if this pharmacology showed some kind of modulation of the disease mechanism. A pharmacology biomarker will provide support for the last two points and show that your molecule binds to the intended target and causes an effect. However, it is important to realise that a positive effect on your pharmacology biomarker in your healthy volunteer study does not necessarily provide any evidence that your molecule will be efficacious, since your initial hypothesis might be wrong. What it does provide is increased confidence of target engagement and guidance in your dose predictions for later stage studies. If no target engagement can be achieved then there is no reason to continue investing in the molecule. It is also critical to realise that the discovery and validation of a pharmacology biomarker is not a trivial undertaking and a defined strategy needs to be implemented as early as possible in the discovery process when a pharmacological tool is available. Figure 1 shows a timeline of suggested FTE investments for a translatable pharmacology biomarker identification and validation.
The pre-clinical strategy for biomarker identification needs to factor in the feasibility and ease of use in a clinical setting i.e. translatability from pre-clinical to clinical is a key factor. There is little point in spending time and effort developing a pharmacology biomarker that can only be used in a pre-clinical setting. A simple strategy for identifying a translatable pharmacology biomarker is outlined in Figure 2, separating the approach into ‘hypothesis driven’ and ‘non-hypothesis driven’ paths. The most efficient is the evidence based strategy and if the identification of a biomarker is considered by the pre-clinical scientist during the exploratory phase, a number of obvious hypothesis driven opportunities are likely to fall out and can then be explored in parallel to the normal drug discovery process. However, for novel targets, this approach can be difficult and a nonhypothesis driven strategy might be the only path forward, but this approach requires for example multiple tools available to better understand the link between target and pharmacology. A number of available translatable pharmacology biomarkers are exemplified in Figure 2. More specific examples of how some translational pharmacology biomarkers that can be used in the drug discovery process will be discussed below.
Physiological biomarkers
There is a range of translatable physiological biomarkers (e.g. blood pressure, heart rate, core body temperature, sleep and EEG) that can be used to assess target engagement. As an example, electrophysiological measurements will be discussed briefly here7. Sleep and electroencephalography (EEG) changes have been shown to be relatively easy, non-invasive, inexpensive and replicable within subjects, which makes them ideal for measuring drug induced changes in a crossover design both in clinical and pre-clinical studies8,9. Positive results in normal subjects provide evidence of a central nervous system effect and, as mentioned above, can be used as translatable pharmacology biomarkers. In short, it has been shown that human and rodent sleep is structurally very similar and most of our knowledge of sleep regulation originates from preclinical experiments. It is also well understood how different neurotransmitters systems are involved in the regulation of sleep changes10-12. Due to the nature of translatable research, there will always be differences in recording and analysis techniques across different species and this needs to be taken into consideration during the biomarker identification stage and minimise the impact of these differences. It has been shown convincingly that drugs from different drug classes interact with the sleep regulating centres in both humans and rodents in a similar way, both under normal conditions and in models of insomnia7. Therefore drug induced changes in sleep pattern are ideal as non-specific translatable pharmacology biomarkers in the drug discovery process.
Soluble biomarkers
In clinical studies, the biofluid of choice due to technical ease of collection and high subject compliance is whole blood. Blood can be harvested in to a variety of vac-containers containing a host of alternative reagents that enable immunoassays, transcriptional profiling and flow cytometry to be performed optimally resulting in data from which key project decisions can be derived. The general workflow is highlighted in Figure 3 opposite. Briefly, whole blood is harvested over a time course then aliquoted and mixed with the ex-vivo challenge, following the required incubation time, the samples are treated in accordance with the protocol for the platform they are to be used on and then analysed. This assay platform has been key to demonstrate that a pharmacologically active dose has been administered, especially important in projects where the compound has extremely high plasma protein binding and enables the true PK/PD relationship to be determined as well as an accurate dose prediction for future clinical studies. To exemplify the value obtained using ex-vivo assays, two examples are described below.
The use of flow cytometry for the quantification of cellular biomarkers in both pre-clinical and clinical studies has become relatively routine practice in industry and hospital laboratories. The application and versatility of the available methodologies such as morphology, cell activation markers, intra-cellular phospho-protein, cytokine determination and the multi-sample reading makes this the platform of choice. To exemplify the use of flow cytometry as a pharmacology biomarker, a whole blood ex-vivo assay was developed to support a first in human clinical study targeting a histamine receptor. In this study, the subjects were provided with a single dose of the histamine H4 antagonist and blood was harvested at pre-designated time points in a time course up to 24 hours (Figure 4). Following the blood draw, the samples were then stimulated with the receptor agonist histamine and the response measured using the flow cytometry method for cellular morphology (side scatter and forward scatter) gating for eosinophils. The resulting data demonstrated that the response for the lower doses was comparable with placebo. The response to the three milligram dose demonstrated a pharmacologically active dose that decreased rapidly to base line over a period of 24 hours. However, the final two doses of 12 milligrams and 48 milligrams showed a comparable pharmacological response that provided the desirable exposure over the course of the study. Of significant interest, given the selective cellular expression of the target and its potential role in disease, the 12 milligrams dose provided maximal pharmacology and going beyond this dose did not provide any advantages and may only increase any chance of adverse events occurring due to off-target activity.
Historically, immunoassays have been the key platform for both biomarker discovery and clinical utility, with numerous of examples of approved assays in routine clinical use. In this innovative example, we have ultimately used a human ex-vivo blood assay in a first in human clinical study in order to confirm administration of a pharmacologically active oral dose of a Prostanoid EP2 receptor antagonist. Briefly, the assay relies on the observation that lipopolysaccaride (LPS) acting via the TLR4 receptor stimulates the synthesis and release of TNFα. However, in the presence of Prostaglandin E2 (PGE2), the production and release of TNFα can be inhibited. Therefore, in the presence of an EP2 receptor antagonist, which inhibits the action of the EP2 receptor agonist, the production of TNFα can be restored in a dose dependant manner. This assay has been used for compound selection and validated for clinical use through demonstrating the sensitivity, reproducibility and robustness of the intra and inter subject response over a two week period. Furthermore, the assay was transferred to the clinical lab through a detailed SOP and hands-on training resulting in high quality data that clearly demonstrated a pharmacologically active dose was administered, critical for PK/PD modelling and further dose selection.
In summary, this review has described how the use of translational pharmacology biomarkers can help drive early decisions in the drug discovery process. More specifically translational pharmacology biomarkers significantly impact the drug development process and allows a project to a) prove the pharmacology and target engagement; b) select the right compound and dose (PK/PD model); c) make informed decision early and stop progression or; d) invest with confidence that the mechanism will be tested in the disease.
References
- Kola, I., Landis, J. (2004). Can the pharmaceutical industry reduce attrition rates? Nature Reviews Drug Discovery, 3:711−715
- Peck, R.W. (2007). Driving earlier clinical attrition: if you want to find the needle, burn down the haystack. Considerations for biomarker development. Drug Discovery Today 12(7/8):289-294
- Bakhtiar (2008). Biomarkers in drug discovery and development. J Pharmacol Toxicol Methods 57:85–91
- Wagner, J.A. (2008). Strategic Approach to Fit-for- Purpose Biomarkers in Drug Development Annu. Rev. Pharmacol. Toxicol. 48:631–51
- Frank, R., Hargreaves, R. (2003) Clinical biomarkers in drug discovery and development. Nature Rev 2:566-580
- Atkinson, A. J., Jr. et al. (2001) Biomarkers and surrogate endpoints: Preferred definitions and conceptual framework Clin Pharmacol Thera 69, 89–95
- Ivarsson, M (2009) Sleep changes as translational pharmacodynamic biomarkers. European Pharmaceutical Review Sept 25 5:40-44
- Kondacs and Szabo (1999). Long-term intra-individual variability of the background EEG in normals; Clinical Neurophysiol 110:1708-1716
- Van Albada, S.J. Rennie, C.J., Robinson P.A. (2007). Variability of model-free and model-based quantitative measures of EEG. J Integr Neurosci. 6(2):279-307
- Saper CB, Scammell TE, and Lu J (2005). Hypothalamic regulation of sleep and circadian rhythms. Nature 437:1257-1263
- Fuller, P.M., Gooley, J.J., Saper, C.B. (2006). Feedback Neurobiology of the Sleep-Wake Cycle: Sleep Architecture, Circadian Regulation, and Regulatory. J Biol Rhythms 21:482-493
- Schwartz, J.R.L., Roth, T. (2008). Neurophysiology of Sleep and Wakefulness: Basic Science and Clinical Implications. Current Neuropharmacology, 6: 367-378