Coronavirus: modelling the world of COVID-19
Posted: 6 July 2020 | Matt Jones (Tessella) | No comments yet
New data models need to be built rapidly to respond to the COVID-19 pandemic. Matt Jones explains how to do it.
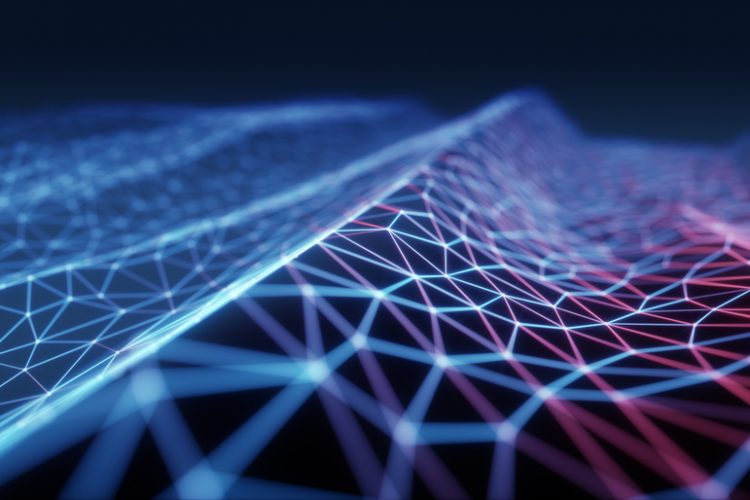
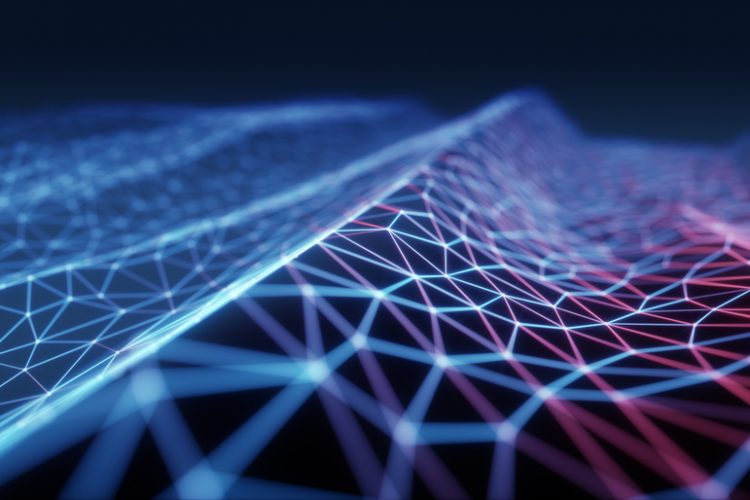
In their drive to develop new therapeutics and vaccines for COVID-19, researchers are building, training and deploying data models at an unprecedented speed.
However, the data they are using is still very new and full of uncertainties. As a result, experts designing drugs and vaccines are spending a lot of time engineering data and rebuilding and validating models. This takes up valuable research time so needs to be conducted quickly. Despite this, if models are rushed through based on old assumptions, rather than being explicitly tailored for the problem at hand, they may not produce the right results and cause problems down the line.
The following four areas should help to guide COVID-19 task forces when responding to the unprecedented challenges before them.
1. Accessing the right data
The core of the current challenge is data uncertainty. Most disease research is built on years of study. Now, we are dealing with data on biological mechanisms and patient responses where our understanding is still evolving. Investigating potential secondary indications of existing drugs, for example, involves data based on subjective assessments by doctors who are still getting to grips with and learning about the disease.
The result is that open source data or data from clinical trials and hospitals may include bias or misreporting and various sources may use different labels and data capture mechanisms. Models build on such data will not produce reliable results.
Metadata should be added on what the data represents – eg, type of molecule or toxicology, but also provenance, timestamps, usage licences, etc. There must also be a consistent taxonomy established for naming things so models (and humans) can find and make sense of the data.
Once complete, data must be fed into central and accessible data stores, while tools and integrators should be setup to feed data to data science teams.
At Tessella, we have seen many projects derailed because modellers drew invalid conclusions from data sets which contained errors, bias or lacked contextual information. In a project with a pharma company – which used pre-clinical data to predict late-stage failures – we discovered the problem was that their modellers said data was hard to find, difficult to understand, laborious to use and risky to draw conclusions from. By addressing this, we significantly reduced failures. If this happens during normal times, it will doubtlessly be a major problem in the current rush for answers.
2. Choosing the right models
The next potential sticking point is building the right model. There is no rule for which approach is best for a particular problem. The nature and context of the issue, data quality and quantity, computing power needs, speed and intended use, all feed into model choice and design. A model which analyses lung scans to monitor disease progression will look very different to a model which analyses molecule libraries to identify likely candidates for new drug targets.
Start by understanding the type of problem. Is it classification or regression, supervised or unsupervised, predictive, statistical, physics-based, etc? Do not just settle for the approach you are most familiar with.
Screen data to understand what is possible. Perform rapid and agile early explorations using simple techniques to spot the correlations that will guide your plan. From this analysis, identify candidate modelling techniques (eg, empirical, physical, stochastic, hybrid) before narrowing down to the most suitable model for that specific problem.
‘Most powerful’ is not the same as ‘must suitable’. Techniques such as machine learning need lots of well understood data and so are ill-suited to most COVID-19 challenges at this stage. Approaches such as Bayesian uncertainty quantification may be better where limited trusted data is available.
3. Ensure your answers are trusted
So does a lack of explainability. If users cannot understand why the model reached a result, they will end up having to repeat work manually. A good model contains tools to analyse what data was used, its provenance and how the model weighed different inputs, then report on that conclusion in clear language.
Privacy and ethical concerns also undermine trust. Patient data must be freely given and kept securely. If, as some suspect, there is variability in disease response between ethnicities, these must be reliably accounted for. Models which only work for white people will quickly be shelved.
4. Deploying models at scale
Models must work in the enterprise, not just for the data scientists.
Usually that involves engineering the final model into a piece of software and integrating it into a mobile or web app, or a bespoke piece of technology. This requires an understanding of the rules and complexities of enterprise IT or edge computing where the model must operate.
Data scientists must make necessary changes to ensure consistency”
This may involve wrapping models in software (‘containers’) which translate incoming and outgoing data into a common format, to allow it to slot into an IT ecosystem. It will require allocating power to compute demands relevant to the application. This means planning for ongoing maintenance, support and retraining. This is where a lot of models face big hold ups, since pharma researchers and even data scientists are not usually software engineers.
If all goes well, the user is presented with a clear interface. They enter the relevant inputs, eg, desired pharmacological properties. The model runs and presents the resulting insight in an easy to understand way that the user is comfortable acting upon.
Bringing it all together for rapid results
Time can be saved by identifying your end objective and being laser-focused on capturing and curating the most relevant data. However, rigour is needed throughout and there are few shortcuts to take. Speed is not about cutting corners; it is about doing things right first time, so you do not have to abandon projects and start again.
That means efficient allocation of resources – selecting the right skills for the right job. Getting data experts to handle the data, modellers to do the models and software engineers to manage the software. Critically, it means giving COVID-19 experts the tools and time to focus where their true expertise lies – understanding the disease and developing drugs and vaccines.
This article is based on Tessella’s whitepaper, COVID-19: Effective Use of Data and Modelling to Deliver Rapid Responses, developed with input from a range of modelling experts.
About the author
Dr Matt Jones holds a PhD in synthetic organic chemistry and has over 20 years of experience in pharmaceutical R&D. He has been at Tessella since 2014, before which he held a number of technical and management roles at GlaxoSmithKline (GSK). In 2015 he was elected to the board of directors of Pistoia Alliance.
Related topics
Big Data, Data Analysis, Informatics, Research & Development (R&D), Viruses