The role of proteomics in the development of personalised cancer medicine
Posted: 18 April 2013 | | No comments yet
Not all cancer patients, even those with the same tumour type, respond to therapy equally well. An understanding of this heterogeneity at the molecular level is crucial for further advances in the development of cancer therapies. Discerning the mechanisms of cancer heterogeneity will lead to a better selection of the most appropriate therapy for each patient and to an improvement in therapeutic outcomes. The success of such personalised cancer therapies requires biomarkers that can be used to stratify patients based on the likelihood that they may respond a particular drug or therapy1. This article discusses the rationale of using proteomics approaches to characterise such biomarkers.
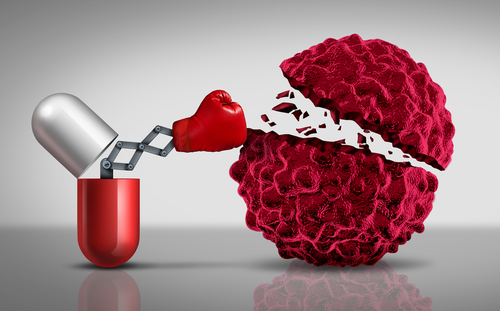
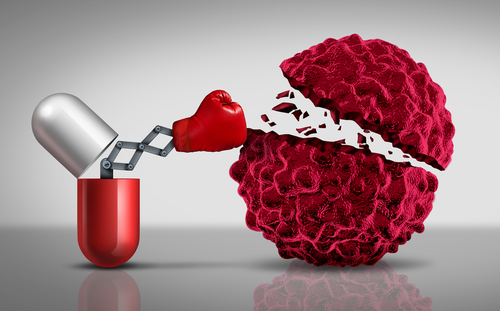
Cancer therapies that target protein kinases are prime examples of the success of personalised medicine2,3. For example, trastuzumab (Herceptin), an antibody inhibitor of the tyrosine kinase HER-2, is efficient in breast tumours that over express HER-2 (about 25 per cent of total). Therefore, patients are selected for therapy based on pathological examination for HER-2 expression. However, it should be noted that about 70 per cent of HER-2 positive patients do not respond to trastuzumab4. Based on these figures, a clinical trial conducted in an unselected population would have shown therapeutic response in less than eight per cent of patients. The selection of patients that were positive for HER-2 was therefore critical for the success of trastuzumab clinical trials and for obtaining regulatory approval4.
Kinase signalling as targets for cancer therapies
HER-2 (also known as ErbB2) is a growth factor receptor and an enzyme with protein kinase activity5. Although not all cancers increase the expression or activity of HER-2, research into cancer biochemistry is leading to the conclusion that all cancers deregulate signalling pathways driven by the activity of protein kinases6. This realisation, together with the success of therapies based on kinase small molecule inhibitors such as imatinib7, made kinases in signalling pathways attractive targets for the development of drugs against different cancer types. Indeed, essentially all pharmaceutical companies have drug development programs that target enzymes in biochemical networks driven by the activity of kinases. Nodes in these networks currently being targeted include, among many others, several receptor tyrosine kinases such as KIT, Flt-3, EGFR and IGF-IR, Ser/Thr kinases such as mTOR, Pim, PAK, MEK, AKT, PKC, and lipid kinases including different isoforms of PI3K5,8. In the future, therapeutic approaches may be available for each of the different 518 protein kinase genes present in humans (or at least for each of the kinases expressed in cancer). As discussed above, patient selection is crucial for the overall success of therapies that target kinase signalling. Therefore, the key question in the field is how to best select patients for therapy in order to increase the success of these novel targeted therapies and gain regulatory approval for patients’ benefit1. Related to this, a more fundamental question is how many different oncogenic routes are there in cells and what are their relative contributions to cancer onset and progression?
Oncogene addiction only partially explains susceptibility to targeted therapies
Cancer growth and survival is sometimes dependent on an aberrantly activated protein or pathway. In such cases, the cancer is said to be ‘addicted’ to that pathway or oncogene9. The reliance of chronic myelogenous leukaemia to the activity of the BCR-Abl is a prime example of oncogene addiction9. Similarly, HER-2 positive cancers that respond to trastuzumab may be addicted to HER-2 activity. Nevertheless, although conceptually appealing, the theory of oncogene addiction does not always explain why some cancers respond to therapies. For example, the fact that 70 per cent of HER-2 positive patients do not respond to trastuzumab appears to contradict the theory of oncogene addiction. Similarly, there does not seem to be a correlation between the sensitivity of cancer cells to PI3K inhibitors with the activity of the pathway that is being targeted10,11. In this regard, a recently reported clinical trial found no significant correlation between the responses to PI3K therapies and PIK3CA mutation status in patients12. Therefore, in these cases, cancer cells were not addicted to PI3K activity despite the pathway being overactive. A phospho proteomic study found that cancer cells resistant to PI3K inhibitors activated several kinase signalling pathways ‘in parallel’10, thus allowing them to proliferate by signalling routes alternative to the one being inhibited. Therefore, cancer cells that are intrinsically resistant to signalling inhibitors may activate several kinase pathways. This is supported by the observation that inhibiting several kinases is more efficient in arresting the proliferation of cancer models than hitting a single target13.
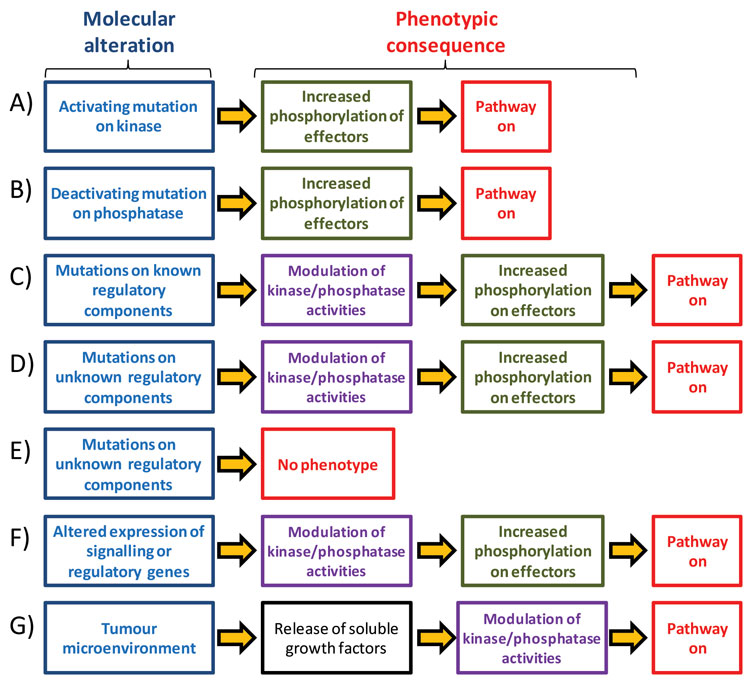
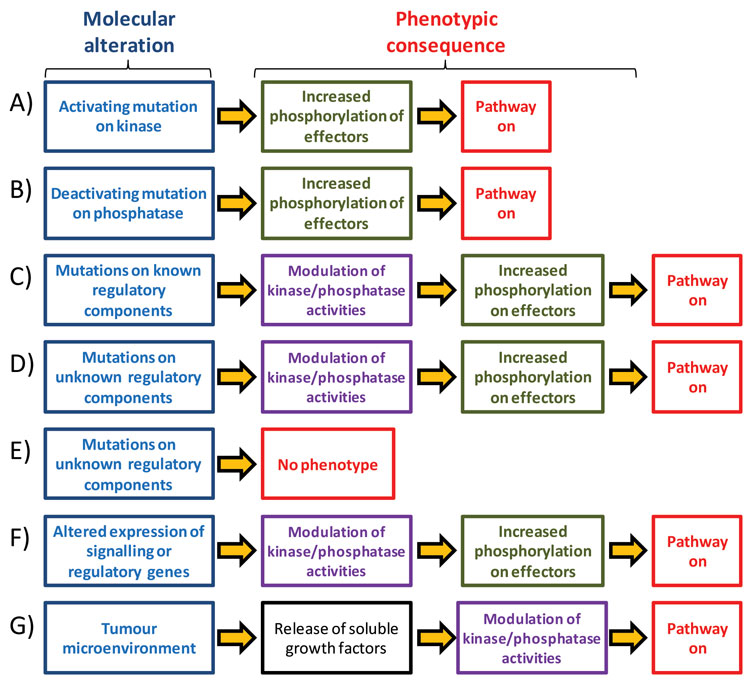
Figure 1: Different molecular events can result in the activation of singling pathways that drive tumour growth Oncogenic signalling pathways may be activated by mutations on kinases (A) or phosphatases (B). Mutations on known or unknown regulatory components can also results in increased pathway activation (C,D). Many of the mutations that are found in cancer do not have a phenotypic consequence (E). Genetic mutations is not the only mechanism to activate a signalling pathway as changes in gene expression (F), epigenetic changes and signals from the tumour extracellular microenvironment (G) can also affect oncogenic pathway activities
The above discussion relates to intrinsic (innate) resistance or sensitivity to therapies; that is, to the sensitivity of cancer cells to kinase inhibitors at presentation. However, it should also be noted that essentially all tumours that initially respond to kinase therapies eventually develop resistance. One of the mechanisms for this acquired resistance is the activation of pathways parallel to those being targeted14,15. It is therefore clear that there are many different routes by which cells can acquire the malignant phenotype.
A modified theory to rationalise why some cancers respond to kinase inhibitors, whereas others are resistant, is that cells have many different ways of wiring the signalling network (this plasticity may have evolved as a way of coffering robustness to biological systems16). Inhibitors against a single kinase may be effective in cancers that activate a single signalling route whereas most cancers activate several nodes of the signalling network. Cancers addicted to a single signalling route quickly develop resistance by activating nodes in the network in parallel to, or downstream of, those being targeted. To advance, the field needs robust biomarkers that can be used to infer, as comprehensively as possible, how biochemical networks are wired in each tumour when the patient is first examined, and how these are rewired should resistance ensue.
Genetic mutations are not ideal indicators of pathway activities
Signalling pathways often acquire oncogenic properties by activating mutations on kinases or deactivating mutations on phosphatases that control pathway fluxes (Figure 1A and 1B). Examples of this include activating mutations on B-RAF, often found in melanomas17, and on PI3K (gene name PIK3CA), a lipid kinase often found mutated in colon and breast cancers, among others18. Inactivating mutations on phosphatases (including MKPs and PHLPP) that oppose oncogenic kinase activation are also found in cancer19,20 and are expected to have the same phenotype as mutations on kinases (Figure 1B). Mutations on regulatory molecules that act upstream of these pathways are also a mechanism to activate oncogenic signalling (Figure 1C), a classical example of this being mutations on Ras upstream of RAF-MEK-ERK and PI3K/AKT pathways21.
Spurred by the success of cancer genomics, further gene sequencing efforts are uncovering thousands of mutations in different cancer types22. However, the role, if any, of the majority of these mutations in cancer onset and progression remains unknown. Since we do not yet have a complete picture of how biochemical networks are wired, some of these mutations may activate or deactivate regulatory components upstream of kinase signalling pathways and could thus have value as markers of pathway activities (Figure 1D). However, it is also clear that many mutations do not change protein activity, as many of these mutations occur as a result of genomic instability in cancer cells (Figure 1E). At present, it is not possible to accurately distinguish between mutations that drive cancer progression (driver mutations) to those without a consequence for cancer development (passenger mutations), thus making it difficult to infer how genetic mutations rewire the signalling network23.
Even if we knew the consequences that each genetic mutation has on the activity of biochemical pathways, it is unlikely that sequencing full genomes will provide a comprehensive picture of pathway activation in cancer. This is because genetic mutations are not the only means by which enzymes may be aberrantly activated. Increased copy number of oncogenes or decreased expression of tumour suppressors can also affect pathway activities (Figure 1F), as exemplified by over expression of HER-2 in breast cancer. In addition, kinase signalling can also be activated in cancer cells by growth factors present in the tumour microenvironment (Figure 1G), which are secreted from stromal cells present in tumours and which act on surface receptors on cancer cells24. Stromal cells are untransformed and non-malignant and are found in tumours as a mixture of fibroblasts, epithelial cells and cells of the immune system such as macrophages and neutrophils. Activation of signalling pathways by heterotypic interactions, growth factors and cytokines derived from the stroma has an effect on how cancer cells respond to targeted compounds25.
Given the several molecular phenomena discussed above (and schematically illustrated in Figure 1) by which signalling pathways may be activated in cancer, predictive biomarkers based on surrogate readouts of pathway activity, such as genetic mutations or protein expression, will produce numerous false positives and negatives. This perhaps explains why only 30 per cent of HER-2 positive breast tumours respond to trastuzumab. Technology for direct assessment of pathway activities, complementary to genomic techniques, is therefore needed to advance cancer personalised medicine.
Phosphoproteomics as a tool for comprehensive and direct quantification of kinase signalling activity
Cells contain thousands (perhaps more than 100,000) phosphorylation sites on proteins. Each of these phosphorylation sites is, by definition, the result of a kinase activity (opposed by phosphatase activity), and by extension, a measure signalling pathway activity (Figure 2). For example, the activities of the kinases JAK, PDK1 and MEK are currently measured by examining key phosphorylation sites on their substrates STAT, PKB/Akt and ERK, respectively. These measurements are commonly performed using immunochemical techniques, but recently, mass spectrometry (MS) methods have been developed that enable measuring the phosphorylation of thousands of sites simultaneously. Since, as discussed above, each of these sites is the result of a kinase activity; these techniques can now be used to measure kinase signalling with unprecedented depth.
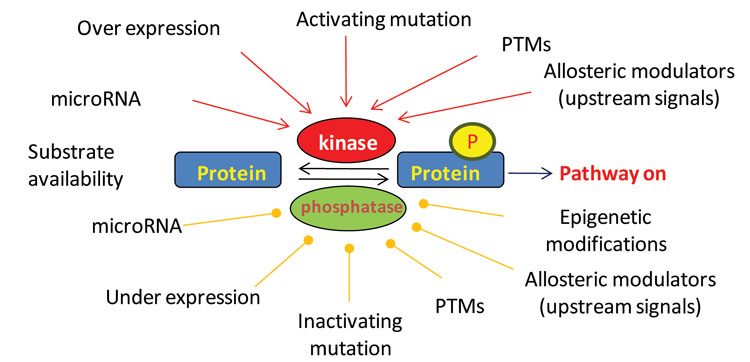
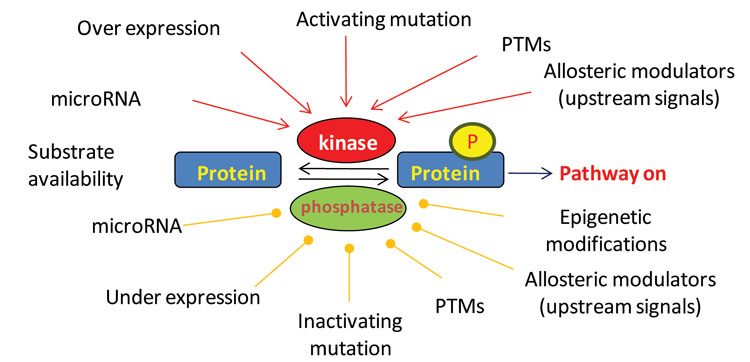
Figure 2: Different molecular events can result in the activation of singling pathways that drive
tumour growth
Oncogenic signalling pathways may be activated by mutations on kinases (A) or phosphatases (B). Mutations on
known or unknown regulatory components can also results in increased pathway activation (C,D). Many of the
mutations that are found in cancer do not have a phenotypic consequence (E). Genetic mutations is not the only
mechanism to activate a signalling pathway as changes in gene expression (F), epigenetic changes and signals
from the tumour extracellular microenvironment (G) can also affect oncogenic pathway activities
Advances that have made the development of phosphoproteomics possible include the introduction of mass spectrometers with high mass accuracy, sensitivity and duty cycle26, the development of chromatographic methods for robust and reproducible enrichment of phosphopeptides27,28, and computer programs to extract qualitative and quantitative information from MS data29,30. Quantitative MS has traditionally relied on the use of chemical or metabolic labelling techniques, which involve tagging proteins in the samples that are being compared with compounds of different mass31. Since the number of tags is limited, these methods are not ideal for the analysis of primary samples and for clinical studies. Thus, in order to be able to quantify phosphorylation comprehensively in primary samples, techniques for label-free quantification have been developed28. These label-free methods were found to be more accurate and precise than quantification by immunoblotting10, in addition to being able to compare thousands of phosphorylation sites across as many samples as required in the experimental design.
Development of bioinformatic tools to infer kinase pathway activation from phosphoproteomics data
Although conceptually possible, in practice, inferring kinase activity from phosphoproteomics is not straightforward. This is because there is limited information on the connectivity between kinases and their substrates. In addition, different kinases may phosphorylate the same substrates and substrates in one cell line or tissue may not be phosphorylated in other cell types. Thus, informatics tools need to be developed in order to infer pathway activity based on phosphoproteomics data. Approaches based on motif analysis are encouraging32. We have also shown an approach, named Kinase Substrate Enrichment Analysis (KSEA), which can be used to infer kinase pathway activation from phosphoproteomics. Application of the technique to infer heterogeneity of signalling in primary leukaemia cells showed that the most frequently activated pathways in this malignancy were the PI3K/Akt, Casein Kinase and PAK. Feeding readouts of kinase activity into mathematical models showed that it was possible to predict the degree or sensitivity of primary leukaemia to an inhibitor of PI3K with unprecedented accuracy33.
Concluding remarks
MS-based proteomics is reaching maturity. These techniques allow measuring signalling in primary tissues with unprecedented depth and accuracy. Further improvements in bio – informatics and biomarker characterisation will allow measuring how signalling networks are wired in individual patients with high accuracy and penetration, thus paving the way for the development of personalised therapies based on the rational assessment of tumour biochemistry.
References
- Sawyers, C.L., The cancer biomarker problem. Nature, 2008. 452(7187): p. 548-52
- Cho, S.H., J. Jeon, and S.I. Kim, Personalized medicine in breast cancer: a systematic review. J Breast Cancer, 2012. 15(3): p. 265-72
- Ross, J.S., et al., The HER-2 receptor and breast cancer: ten years of targeted anti-HER-2 therapy and personalized medicine. Oncologist, 2009. 14(4): p. 320-68
- Tan, A.R. and S.M. Swain, Ongoing adjuvant trials with trastuzumab in breast cancer. Semin Oncol, 2003. 30(5 Suppl 16): p. 54-64
- Yarden, Y. and G. Pines, The ERBB network: at last, cancer therapy meets systems biology. Nat Rev Cancer, 2012. 12(8): p. 553-63
- Hanahan, D. and R.A. Weinberg, Hallmarks of cancer: the next generation. Cell, 2011. 144(5): p. 646-74
- Sawyers, C.L., et al., Imatinib induces hematologic and cytogenetic responses in patients with chronic myelogenous leukemia in myeloid blast crisis: results of a phase II study. Blood, 2002. 99(10): p. 3530-9
- Dar, A.C. and K.M. Shokat, The evolution of protein kinase inhibitors from antagonists to agonists of cellular signaling. Annu Rev Biochem, 2011. 80: p. 769-95
- Sawyers, C.L., Shifting paradigms: the seeds of oncogene addiction. Nat Med, 2009. 15(10): p. 1158-61
- Alcolea, M.P., et al., Phosphoproteomic analysis of leukemia cells under basal and drug-treated conditions identifies markers of kinase pathway activation and mechanisms of resistance. Mol Cell Proteomics, 2012. 11(8): p. 453-66
- Dan, S., et al., Correlating phosphatidylinositol 3-kinase inhibitor efficacy with signaling pathway status: in silico and biological evaluations. Cancer Res, 2010. 70(12): p. 4982-94
- Janku, F., et al., PI3K/AKT/mTOR inhibitors in patients with breast and gynecologic malignancies harboring PIK3CA mutations. J Clin Oncol, 2012. 30(8): p. 777-82
- Knight, Z.A., H. Lin, and K.M. Shokat, Targeting the cancer kinome through polypharmacology. Nat Rev Cancer, 2010. 10(2): p. 130-7
- Serra, V., et al., PI3K inhibition results in enhanced HER signaling and acquired ERK dependency in HER2- overexpressing breast cancer. Oncogene, 2011. 30(22): p. 2547-57
- Qi, J., et al., Multiple mutations and bypass mechanisms can contribute to development of acquired resistance to MET inhibitors. Cancer Res, 2011. 71(3): p. 1081-91
- Amit, I., R. Wides, and Y. Yarden, Evolvable signaling networks of receptor tyrosine kinases: relevance of robustness to malignancy and to cancer therapy. Mol Syst Biol, 2007. 3: p. 151
- Davies, H., et al., Mutations of the BRAF gene in human cancer. Nature, 2002. 417(6892): p. 949-54
- Samuels, Y., et al., High frequency of mutations of the PIK3CA gene in human cancers. Science, 2004. 304(5670): p. 554
- Keyse, S.M., Dual-specificity MAP kinase phosphatases (MKPs) and cancer. Cancer Metastasis Rev, 2008. 27(2): p. 253-61
- Gao, T., F. Furnari, and A.C. Newton, PHLPP: a phosphatase that directly dephosphorylates Akt, promotes apoptosis, and suppresses tumor growth. Mol Cell, 2005. 18(1): p. 13-24
- Malumbres, M. and M. Barbacid, RAS oncogenes: the first 30 years. Nat Rev Cancer, 2003. 3(6): p. 459-65
- Greenman, C., et al., Patterns of somatic mutation in human cancer genomes. Nature, 2007. 446(7132): p. 153-8
- Bozic, I., et al., Accumulation of driver and passenger mutations during tumor progression. Proc Natl Acad Sci U S A, 2010. 107(43): p. 18545-50
- Joyce, J.A. and J.W. Pollard, Microenvironmental regulation of metastasis. Nat Rev Cancer, 2009. 9(4): p. 239-52
- Straussman, R., et al., Tumour micro-environment elicits innate resistance to RAF inhibitors through HGF secretion. Nature, 2012. 487(7408): p. 500-4
- Hu, Q., et al., The Orbitrap: a new mass spectrometer. J Mass Spectrom, 2005. 40(4): p. 430-43
- Thingholm, T.E. and M.R. Larsen, The use of titanium dioxide micro-columns to selectively isolate phosphopeptides from proteolytic digests. Methods Mol Biol, 2009. 527: p. 57-66, xi
- Montoya, A., et al., Characterization of a TiO(2) enrichment method for label-free quantitative phosphoproteomics. Methods, 2011. 54(4): p. 370-8
- Cutillas, P.R. and B. Vanhaesebroeck, Quantitative profile of five murine core proteomes using label-free functional proteomics. Mol Cell Proteomics, 2007. 6(9): p. 1560-73
- Cox, J. and M. Mann, MaxQuant enables high peptide identification rates, individualized p.p.b.-range mass accuracies and proteome-wide protein quantification. Nat Biotechnol, 2008. 26(12): p. 1367-72
- Timms, J.F. and P.R. Cutillas, Overview of quantitative LC-MS techniques for proteomics and activitomics. Methods Mol Biol, 2010. 658: p. 19-45
- Linding, R., et al., Systematic discovery of in vivo phospho rylation networks. Cell, 2007. 129(7): p. 1415-26
- Casado, P. and P.R. Cutillas, Kinase Substrate Enrichment Analysis provides insights into the heterogeneity of signaling in leukemia cells. Submitted