PAT Series: Predictive monitoring and control approaches in biopharmaceutical manufacturing
Posted: 3 September 2015 |
Predictive monitoring is a key feature of biopharmaceutical manufacturing; making predictions about the key process end points such as process performance indicators or quality attributes using a process model offers the unique advantages of process improvement and optimisation, and helps give insights into variability.
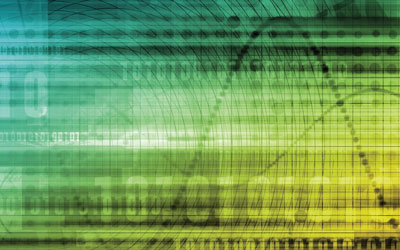
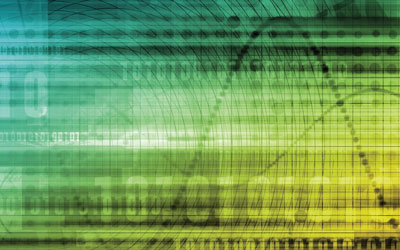
However, whilst model-predictive monitoring is advantageous, it is also desirable to apply model predictions for closed loop control of biologics manufacturing using various process analytical technology (PAT) tools. We summarise some of our experiences with predictive monitoring, closed loop control using in situ Raman spectroscopy and state-space methods for model predictive control of cell culture bioreactors.
Introduction
As in other process industries, biopharmaceutical manufacturing processes generate a lot of data during the clinical and commercial production runs collected over many stages on many different variables. It is imperative to monitor these variables across the stages and batches in real-time to detect any developing trends that may affect batch performance or lead to loss of a batch. It also is of great interest to understand trends in process variables that may impact on product quality attributes (PQA) and key performance indicators (KPI). Regulatory Agency guidelines also promote the lifecycle concept linking product and process development with the commercial manufacturing process. We have previously discussed and presented very powerful PATs such as application of real-time multivariate statistical process monitoring in the open-loop mode of operation where many variables across many stages in a biopharmaceutical manufacturing process are monitored and weak signals are detected.
While detecting weak signals is important to enable engagement in process monitoring and troubleshooting, it is also highly desirable to control the process performance and product quality via closed-loop approaches using model-based predictive techniques. As described in the PAT guidance, some of these control applications may involve process chemistry tools and analysers, whilst others may depend on soft-sensors and other predictive technologies. This aligns with a quality-by-design approach and ensures the target product profile is met by establishing an effective control strategy. Ample literature exists in closed loop and model predictive control in various industries including fermentation technologies. However, actual industrial applications of model-based or model-predictive control are not yet very common in biopharmaceuticals manufacturing.
We shall summarise some of our experiences with open- and closed-loop models in the following areas:
- Multivariate predictive monitoring;
- Raman probe-based monitoring and model predictive control; and
- State-space model-based monitoring.
Multivariate predictive monitoring
This method involves using readily available and frequently sampled or discrete data on measured variables in the bioprocess equipment. These may include in-line probes, on-line instruments and offline sample assay results. For instance, in a bioreactor, typical frequently measured variables include various gas sparge rates (O2, CO2, Air), dissolved O2, pH, pressure, working and feed volumes and temperature. Typical discrete variables measured infrequently are metabolites, viable cell density, cell viability and dissolved CO2. Soft-sensors are often used to estimate variables that are unmeasurable, replace more time consuming or costly techniques and improve frequency of data availability. Lastly, pre-processing and calculation of additional derived variables, such as specific growth rate or metabolite production, can improve a model’s predictive capability.
Predictive models may be developed only if each significant operational variable spans a sufficient range (and/or if there is a first principles model describing the relationship) that will allow for correlation to process performance and product quality8,10,12-13. These predictive models, acting as soft-sensors as shown in Figure 1 (page 00), allow for predictions about KPIs and PQAs in near real-time.
One example of this methodology uses a predictive multivariate model to estimate KPIs and PQAs in near real-time, where they were traditionally measured through offline process sampling and analytics, for use in a commercial manufacturing facility to improve batch productivity, operational capacity and flexibility by optimising the harvest timing of the bioreactor. If the length of the bioreactor culture duration can be increased within the operating range, unit productivity will be increased. However, this must be balanced with key variables in the process and performance that must be met to ensure product quality.
In this example, the KPIs modelled are glucose, cell viability and a PQA. Glucose is critical to the growth and production within cells. Due to inherent variability within the cells and raw materials, consumption of glucose can vary from batch to batch. Therefore, it is imperative that glucose in the medium will not be depleted. Prediction of the time of glucose depletion confirms the longest the culture may be operated. Cell viability reflects the state and health of the cell culture. The prediction of this KPI ensures that the culture will not fail the KPI prior to harvesting. Lastly, one of the key PQAs (that is impacted by the cell culture conditions) measured in the downstream purification process was identified due to the significant correlation with the cell culture process. Predicting that the PQA is within its limits is the last step to determine the optimal culture duration.
The use of multiple environmental, KPI and PQA prediction models allows operations to make more informed business decisions earlier in the production of a batch. Figure 2 (page 00) illustrates how the predictions allow for determination if an early harvest of the cell culture might be needed to ensure KPIs and PQAs meet limits or to extend the cell culture duration for productivity improvement.
A current use case (Batch A and B) demonstrates the ability to extend batches for increased production. First, the PLS scores, Hotelling’s T2 and Distance to Model X (DModX) values are verified to be within acceptable limits for assurance of prediction. Figure 3 (page 00) shows that both batches meet data fit criteria. Next; viability, glucose and the PQA predictions determine batch extension capability. Only Batch B could be extended as Batch A had a viability prediction indicating that an extended culture would fall below its action limit.
Another example where predictive modelling provided commercial manufacturing the tools to make more informed decisions comes from an investigation into a lower-than-expected KPI at the final stage of a multi-stage purification process. An evaluation into over 100 input variables from the entire process (Figure 4a; page 00) identified many variables that contribute significantly to the KPI (green bars), and others that do not (red bars). Due to the large number of variables across all stages, understanding how the variables are related is difficult to discern. A more efficient way to tackle the problem is to understand how the KPI across the six different stages influence the final KPI (Figure 4b; page 00). This focuses the investigation away from stages that do not influence the final KPI (red bars), and allows a more concerted effort into understanding the stages that do influence the KPI (green bars). Stage-specific models can then be built to better understand which variables should be monitored and controlled to impact the KPI (Figure 4c; page 00).
Raman probe-based monitoring and control
Raman spectroscopy is a powerful PAT tool to enhance process monitoring capabilities in the biopharmaceutical industry. An online Raman spectroscopy probe can supplement existing process and operating data and enhance the predictive capability of online monitoring systems. To achieve online monitoring using Raman spectroscopy, calibration models need to be created that translate Raman spectra into useful process parameters (i.e., acting as a real-time soft-sensor). We achieved this by combining multivariate (MV) modelling tools with Raman spectroscopy15-16. An example of a calibration model – for glucose – is provided in Figure 5 (page 00). Similar calibration models are created for other critical cell culture parameters such as viable cell density, viability, lactate, glutamate, glutamine, ammonium and osmolality.
Once the calibration models are developed, they can be integrated to a Distributed Control System or Data Historian to perform online monitoring of the ongoing process (Figure 6; page 00).
Model predictive control (MPC) can be used to optimise batch trajectory based on historical and real-time results (Figure 7; page 00). The MPC model (batch trajectory optimisation) is a supervisory model which resides outside of the traditional open loop setup. The open loop may have additional MV models used to perform online monitoring which can be setup independently from the MPC model or feed into the MPC model as inputs. The MPC model takes information from the open loop as well as data from its internal training set to predict an optimised trajectory by suggesting new process set points.
Our MPC prediction model was used to optimise final day viable cell density (VCD) for a cell culture process run on a bench-scale seed bioreactor. In this example, temperature, pH and O2 are operational parameters that the MPC model used to optimise the process trajectory. The Scores, Hotelling’s T2 and DModX results are calculated and available for the scientist. The MPC model uses sample measurements from the process that is running at the time to predict an optimised trajectory for the process. When we simulated the closed loop control conditions, an estimated 23% higher final VCD (Table 1; page 00) was predicted in comparison to open loop control case14. While other controlled and uncontrolled load disturbances (such as raw material variation) need to be taken into account, this initial study at bench-scale provided affirmation that the MPC approach was promising.
State-space model-based monitoring and control
For both predictive monitoring and model predictive control, a compact and accurate description of the dynamic behaviour of the system is desirable. Dynamic models describing the system of interest can be constructed using the first principles of physics, chemistry and biology. While they are very strong and predictive, the first principles models are difficult and expensive to derive, as they require a deep and full understanding and knowledge of the processes. Moreover, the first principles model is often complex and simulations take a considerable amount of time. Thus, the first principles model may not be suitable for fast on-line applications.
An alternative way of developing models is via system identification17. In system identification, the goal is to estimate the dynamic models directly from the observed input data and output data. System identification approach leverages the ample data that we acquire from modern control systems, PAT instruments and data acquisition tools. State-space models derived from system identification are flexible, yield compact and accurate models that are suitable for fast on-line applications and for model predictive control.
The general form of a state-space model17 is given as:
,, x,t+1.=Ax,t.+Bu,t.+Ke,t.- y,t.=Cx,t.+Du,t.+e,t…
where u is the process input vector, y is the process output vector, x is the state vector, e is the noise vector. A, B, C, D and K are state-space matrices and t denotes the time step. Given the process input and output data, the state-spaces matrices can be estimated by system identification. Once the state-spaces matrices are estimated, the state-space model is ready for use. It is flexible in a way that the prediction of the output can be made at every time step.
We take the mammalian cell culture processes as an example here. The process input vector is
u=,,-,–2. -,–2.-..
where gluc denotes the glucose concentration, ,–2. the partial oxygen pressure, and ,–2. the partial carbon dioxide pressure.
The process output is defined as
y=,.
where VCD denotes the viable cell density.
Both the input and output data are collected from the bioreactor measurements. MATLAB system identification toolbox18 was used to estimate the state-space matrices. After the state-space matrices are estimated from system identification, the estimated state-space model is applied to predict VCD. The predicted VCD and the experimental data are shown in Figure 8 (page 00). The prediction shows agreement with the experimental data, with a root mean square error (RMSE) of 10.18.
MPC can be built on a state-space model. The objective function in our case is to maximize the VCD trajectory while penalised by the control action move. The pH, PO2, PCO2 and glucose are subject to the physical constraints. The objective function is expressed as follows:
where NP is the prediction horizon and NC is the control horizon.
We perform the following procedures:
- In the time step t, compute an optimal input signal by maximizing the objective function over a certain prediction horizon in the future using the state-space model;
- Implement the first step of the optimal input signal;
- Move to the next step t+1, compute an optimal input signal by maximizing the objective function over a certain prediction horizon in the future using the state-space model;
- Implement the first step of the optimal input signal;
- Continue move on with the above procedures for the whole batch process duration.
The simulation results show that after MPC is applied, the final VCD could be increased by 20%, and daily-averaged VCD could be increased by 6%.
Conclusions
Applying model predictive monitoring and control has unique advantages in the biopharmaceutical operations which can be generalised into a broader set of other biopharmaceutical manufacturing applications. The key aspect is having a robust model that would be representative of the process behaviour and could handle the controlled and uncontrolled disturbances. Finally, the models used for prediction could take various forms such as empirical, input-output, state-space, first principles mechanistic and a combination of these. A well-developed predictive control model offers advantages on controlling KPIs and PQAs, improving process capability and productivity.
Acknowledgements
The authors would like to thank Sinem Oruklu, Chris Garvin their colleagues at Amgen, and their collaborator Chris McCready of Umetrics.
References
- US Food and Drug Administration (January 2011). Guidance for Industry. Process Validation: General Principles and Practices (FDA, Rockville, MD)[Web][cited 6/17/2015]; Available from: http://www.fda.gov/downloads/Drugs/GuidanceComplianceRegulatoryInformation/Guidances/UCM070336.pdf
- International Conference on Harmonization (ICH), Harmonized Tripartite Guideline, Pharmaceutical Development Q8(R2), (August 2009). (ICH, Geneva, Switzerland) [Web] [cited 6/17/2015]; Available from: http://www.ich.org/fileadmin/Public_Web_Site/ICH_Products/Guidelines/Quality/Q8_R1/Step4/Q8_R2_Guideline.pdf
- Undey, C, Ertunc, S, Mistretta, T, Looze, B. Applied advanced process analytics in biopharmaceutical manufacturing: Challenges and prospects in real-time monitoring and control, Journal of Process Control, 2010, 20:1009-1018
- Undey, C, Looze, B, Oruklu, S, Wang, T, Woolfenden, R. Process Analytics Experiences in Biopharmaceutical Manufacturing, European Pharmaceutical Review, PAT Supplement, 2012, 17(3): 2-9
- US Food and Drug Administration (September, 2004). Guidance for Industry: PAT — A Framework for Innovative Pharmaceutical Development, Manufacturing, and Quality Assurance (FDA, Rockville, MD). [Web] [cited 6/17/2015]; Available from: http://www.fda.gov/downloads/Drugs/GuidanceComplianceRegulatoryInformation/Guidances/UCM070305.pdf
- Yu, L. Pharmaceutical Quality by Design: Product and Process Development, Understanding, and Control, Pharmaceutical Research, 2008, 25(4): 781-791
- Molony, M, Undey, C. PAT Tools for Biologics: Considerations and Challenges. In: Quality by Design for Biopharmaceuticals, Editors, A. Rathore, R. Mhatre, John Wiley & Sons, Hoboken, NJ, 2009
- Undey, C, Low, D, Menezes, JC, Koch, M. PAT Applied in Biopharmaceutical Process Development and Manufacturing: An Enabling Tool for Quality-by-Design, Taylor & Francis, CRC Press, Boca Raton, FL, 2011
- Lauri, D, Lennox, B, Camacho, J. Model predictive control for batch processes: Ensuring validity of predictions, Journal of Process Control, 2014, 24:239-249
- Aumi, S, Corbet, B, Clark-Pringle, T, Mhaskar, P. Data-Driven Model Predictive Quality Control of Batch Processes, AIChE Journal, 2013, 59(8): 2852-2863
- Ashoori, A, Moshiri, B, Khaki-Sedikh, A, Bakhtiari, MR. Optimal control of a nonlinear fed-batch fermentation process using model predictive approach, Journal of Process Control, 2009, 19: 1162–1173
- Cinar, A, Parulekar, SJ, Undey, C. and Birol, G. Batch Fermentation: Modeling, Monitoring and Control, Chemical Industries Series, Marcel Dekker, New York: NY, 2003
- Undey, C, Ertunc, S, Cinar, A. Online Batch/Fed-batch Process Performance Monitoring, Quality Prediction and Variable Contributions Analysis for Diagnosis, Ind. Eng. Chem. Res., 2003, 42(20), 4645-4658
- Wang, T. Model predictive monitoring and control for cell culture bioreactor operations, IFPAC Annual Meeting, Arlington, VA. Jan 21-Jan 24, 2014
- Abu-Absi, NR, et al., Real Time Monitoring of Multiple Parameters in Mammalian Cell Culture Bioreactors Using an In-Line Raman Spectroscopy Probe, Biotechnology and Bioengineering, 2011, 108(5), 1215-1221
- Whelan, J, Craven, S, Glennon, B. In Situ Raman Spectroscopy for Simultaneous Monitoring of Multiple Process Parameters in Mammalian Cell Culture Bioreactors, Biotechnology Progress, 2012, 28(5):1355-1362
- Ljung, L. System Identification: Theory for the user. Englewood Cliffs, New Jersey: Prentice-Hall, Inc., 1999
- Mathworks MATLAB Documentation on the System Identification Toolbox
Biographies
Cenk Undey, PhD, is an Executive Director of Process Development at Amgen. He leads the Digital Integration and Predictive Technologies group within the CMC Lifecycle Management, advancing the process analytical technologies, implementation of real-time multivariate statistical process monitoring and control for biopharmaceutical manufacturing processes. His responsibilities include establishing digital integration of data, information and knowledge produced during process and product development. His group also focuses on first principles modelling, applying machine learning, pattern recognition and data mining technologies to identifying, tracking and controlling variation in the value chain. He has conducted his postdoctoral studies at Illinois Institute of Technology, Department of Chemical and Biological Engineering in the area of Intelligent Systems. He has BS, MS, and PhD all in Chemical Engineering from Istanbul University in Turkey.
Tony Wang is a Senior Engineer in Process Development at Amgen in the Digital Integration and Predictive Technologies group within the CMC Lifecycle Management. He is responsible for multivariate data analysis and advancing process analytical technologies, including Raman, NIR applications in raw materials and cell culture monitoring, as well as model predictive control. Previously he has worked in cell culture within Process Development and also as a process engineer within Facility Engineering at Amgen. Tony earned his BS degree in Chemical Engineering and MS degree in Biomedical Engineering both from University of Calgary in Canada. He is a licensed Professional Engineer within APEGA.
Bryan Looze is a Senior Engineer in Process Development at Amgen in Digital Integration and Predictive Technologies group within the CMC Lifecycle Management. His responsibilities in process analytical technologies include implementing real-time multivariate statistical process monitoring, multivariate data analysis and remote monitoring systems. He obtained his BS degree in Chemical Engineering from the University of Massachusetts.
Yingying Zheng, PhD, is a Senior Engineer in Process Development at Amgen in Digital Integration and Predictive Technologies group within the CMC Lifecycle Management. Her responsibilities include implementing real-time multivariate statistical process monitoring, multivariate data analysis and developing and deploying model-based predictive control technologies. Prior to joining Amgen, she worked in The Dow Chemical Company and in Invensys Operations Management. Yingying holds a BS degree in Polymer Materials and Engineering from Tsinghua University in China, an MS degree in Electrical Engineering and a PhD degree in Chemical Engineering both from University of Southern California.
Myra Coufal, PhD, is a Principal Scientist in Process Development at Amgen in Digital Integration and Predictive Technologies group within the CMC Lifecycle Management. Her responsibilities include developing multivariate models to support commercial manufacturing investigations and process improvement projects, as well as internally promoting the use of multivariate and first principles models during process and product development. Previously, she led a Process Development cell culture team supporting mammalian commercial manufacturing. Myra earned a BA degree in Biochemistry from Harvard University, and a PhD degree in Biology from the Massachusetts Institute of Technology.