What’s next for big data?
Posted: 5 January 2018 | Dr Chris Barber (CEO Lhasa Limited) | No comments yet
The emergence of big data, technological innovations and advancements in scientific research is set to be transformative for the pharmaceutical industry, providing faster access to insights that can drive more informed decision making and accelerate drug development. Dr Chris Barber, CEO of Lhasa Limited, looks at what is next for big data and how pharmaceutical companies can make the most of its associated technologies…
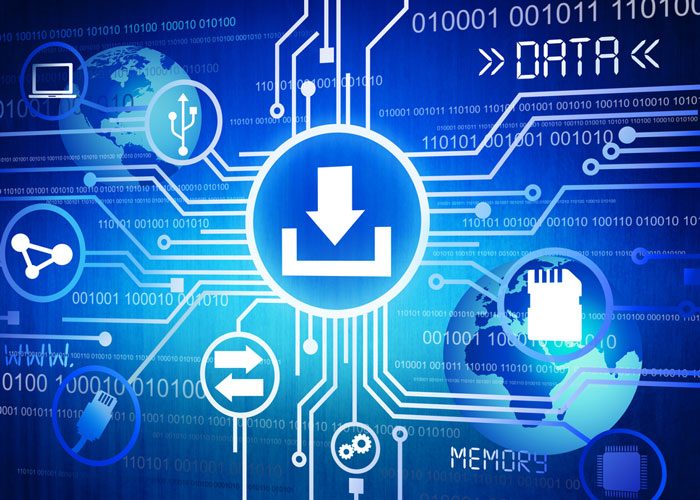
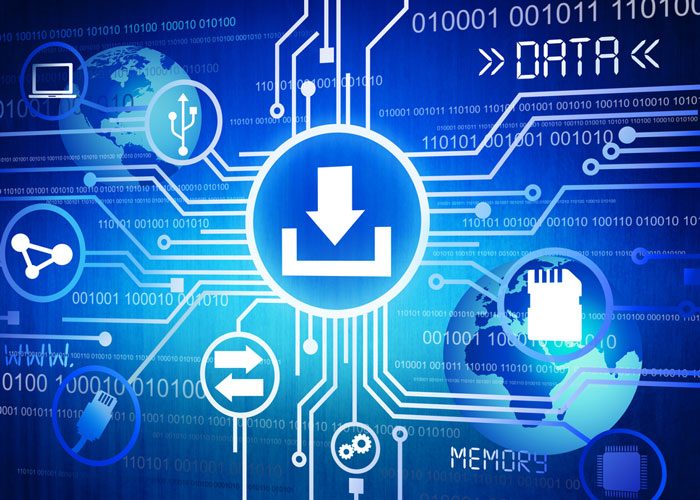
It’s no secret that drug development is often a long and expensive process, with the Association of the British Pharmaceutical Industry estimating that it costs an average of £1.15bn, and takes 12 years to bring the drug to market.
However, despite the high expenditure and lengthy time span involved, big data-driven developments, such as Artificial Intelligence (AI) are conceivably able to increase efficiency. This is showcased through AI’s ability to learn from the existing body of knowledge/data more effectively than any human could hope to do unaided. This potential is being recognised by many of the world’s leading drug companies who are now looking to AI to help streamline their drug development processes, with the ultimate aim of reducing both cost and time.
Nonetheless, in our experience, the distinction between AI and Machine Learning (ML) is often poorly made; our view is that machine learning is encoding a predefined algorithm into a computer, which is then ‘fed’ data to build a model. In contrast, AI attempts to imitate human thinking by enabling the system to recognise the correct approach given the question and the available data.
Currently, ML needs data of a minimum size and consistency to produce dependable results and, in many cases, there are simply too few well-structured datasets. Ultimately, this means ML is unable to work outside of narrow areas of chemical space. With that being said, AI methods are becoming evermore effective at learning from huge sets of unstructured big data, developing the ability to build statistically-based models, which an expert can then verify as being of scientific relevance.
These AI models are not typically ones that a human would derive, so they need to be understood to an appropriate level before they can be used. The experts can then use these predictive models either in their own right, or as a spur to further knowledge discovery.
This emergence of techniques designed to process and analyse big data – which in our field can include text data from published articles and reports, or any one of the ‘omics datasets – means companies can apply these evolving AI techniques to gain a new understanding into drug development.
The rest of this content is restricted - login or subscribe free to access
Why subscribe? Join our growing community of thousands of industry professionals and gain access to:
- bi-monthly issues in print and/or digital format
- case studies, whitepapers, webinars and industry-leading content
- breaking news and features
- our extensive online archive of thousands of articles and years of past issues
- ...And it's all free!
Click here to Subscribe today Login here