Digital design for pharmaceutical product and process development
Posted: 1 July 2019 | Dana Barrasso, Debbie Hooper, Gavin Reynolds, Hervé Barjat, John Henderson, Mike Tobyn | No comments yet
New digital design and manufacturing techniques offer pharmaceutical manufacturers the possibility of a systems-based, digital approach to substantially streamline their design, development and manufacturing processes. The ADDoPT collaborative consortium has been working to explore how emerging process understanding and predictive models can be applied and embedded within industrial workflows to enhance decision making and accelerate development.
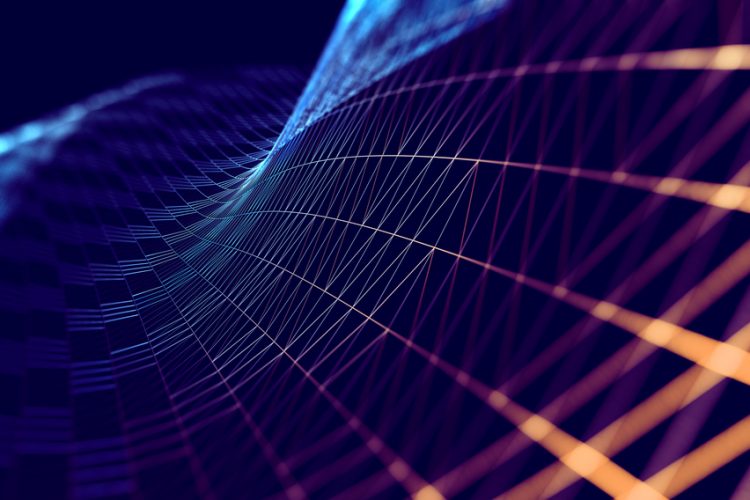
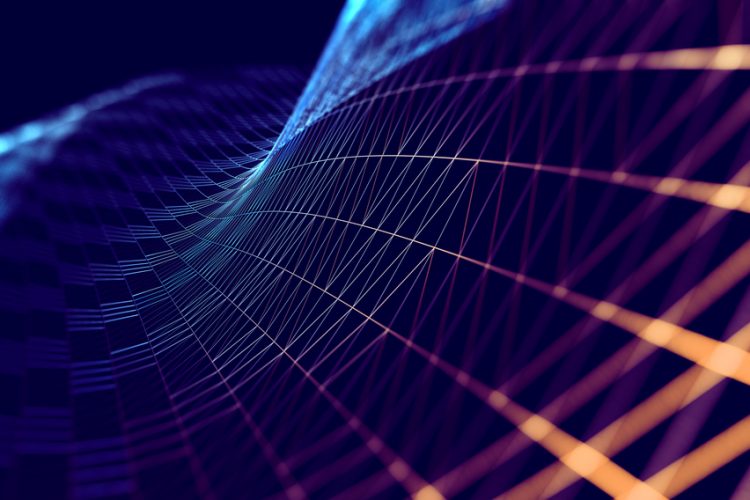
IN AN INCREASINGLY digitalised world, it is evident that pharmaceutical manufacture is still built on heuristics. Process and product development is mostly achieved by “making and testing”, development cycles are long and processes efficiency is sub-optimal. As the sector transitions from the era of “blockbuster” drugs and market expectations change with the drive towards personalised medicines, sector leaders clearly recognise the need to transform the capability, flexibility and speed of their development workflows and supply chains. ADDoPT1 (Advanced Digital Design of Pharmaceutical Therapeutics) is a major UK-based supply chain project established in response to the challenges faced by the industry. The four-year, £20.4m government-industry-academia collaboration has enabled partners from across the pharma value chain to define a system for top-down, knowledge-driven digital design and digital operation for drug products and their manufacturing processes. The aim is to integrate a wide range of predictive models and insight from industrial case studies at four major pharmaceutical companies, allowing more targeted future experimentation, a better understanding of risk and subsequent improved design and scale-up for robust products and processes. In the words of the former chair of the Medicines Manufacturing Industry Partnership, ADDoPT’s objective is to “create virtual medicine manufacturing systems to make sure they are effective and efficient before creating them in the real world”.2
This desire for change is set in the context of a regulated industry, which has used outsourcing/ offshoring extensively to reduce costs. Whilst these may be regarded as braking forces, this is not necessarily so. Regulators like the FDA and EMA support Quality by Design (QbD), an approach that aims to ensure the quality of medicines by employing statistical, analytical and risk-management methodology in their design, development and manufacture. QbD calls for all sources of variability affecting a process to be identified, explained and managed by appropriate measures, ensuring that the finished medicine is consistently delivered “right first time”. Unfortunately, the assurance provided by QbD generally demands substantial amounts of supporting data, which is a significant challenge under the current heuristic, experimentally-driven paradigm.
As well as cost benefits, outsourced manufacture brings associated supply chain risks and mitigation costs
As well as cost benefits, outsourced manufacture brings associated supply chain risks and mitigation costs. Manufacturers want to hand over robust, predictable, reproducible process systems to CMOs with peace of mind. In both cases, digital design tools offer a potential change in approach through reduced data requirements as well as the ability to consider many more factors and identify true critical process parameters and material attributes. Due to the cost and risk pressures, and the need for quality assured products, the pharma sector is actively exploring solutions such as flexible manufacturing and advanced process modelling and control. However, these approaches are not routinely embedded at this point. Manufacturers are nevertheless gradually, but increasingly, coming to regard their development and process data as key assets in the journey towards digital operations. The challenge now is how to systematically harness the potential of advanced materials and process modelling and data analytics to transform decision making in product and process design and control.
Case studies in pharmaceutical digital design
The ADDoPT definition of digital design combines research insight and qualitative and quantitative mechanistic modelling to provide links between raw materials, manufacturing processes and the needs of the patient. It spans all unit operations, processes and procedures during the manufacture of medicines, as well as their impacts, both upstream on the efficiency of product and process design and downstream on product performance. The project partners have worked together to co-ordinate the development of a suite of case studies providing coverage of relevant application areas across this space, in order to evaluate and illustrate the use of new modelling tools and approaches to address a series of relevant, real-world challenges. The following examples provide a flavour of the work undertaken.
Example 1: using computational modelling and big data to overcome tablet sticking
Adherence of an active pharmaceutical ingredient (API) to tablet punches during compression, often referred to as tablet sticking, has been identified as a common and long-standing processing challenge for the pharmaceutical industry that impacts manufacturing efficiency.3,4 Resolution of a tablet-sticking issue is usually complex and rooted in a multi-factorial cause, which therefore needs a holistic approach to finding a solution as part of continuous process improvement. In this case study an approach combining computational modelling at a molecular and materials level with ‘big data’ analysis of the manufacturing process and materials is being applied (see Figure 1). The study covers most of the breadth of the ADDoPT remit from API properties through to drug product manufacturing performance.
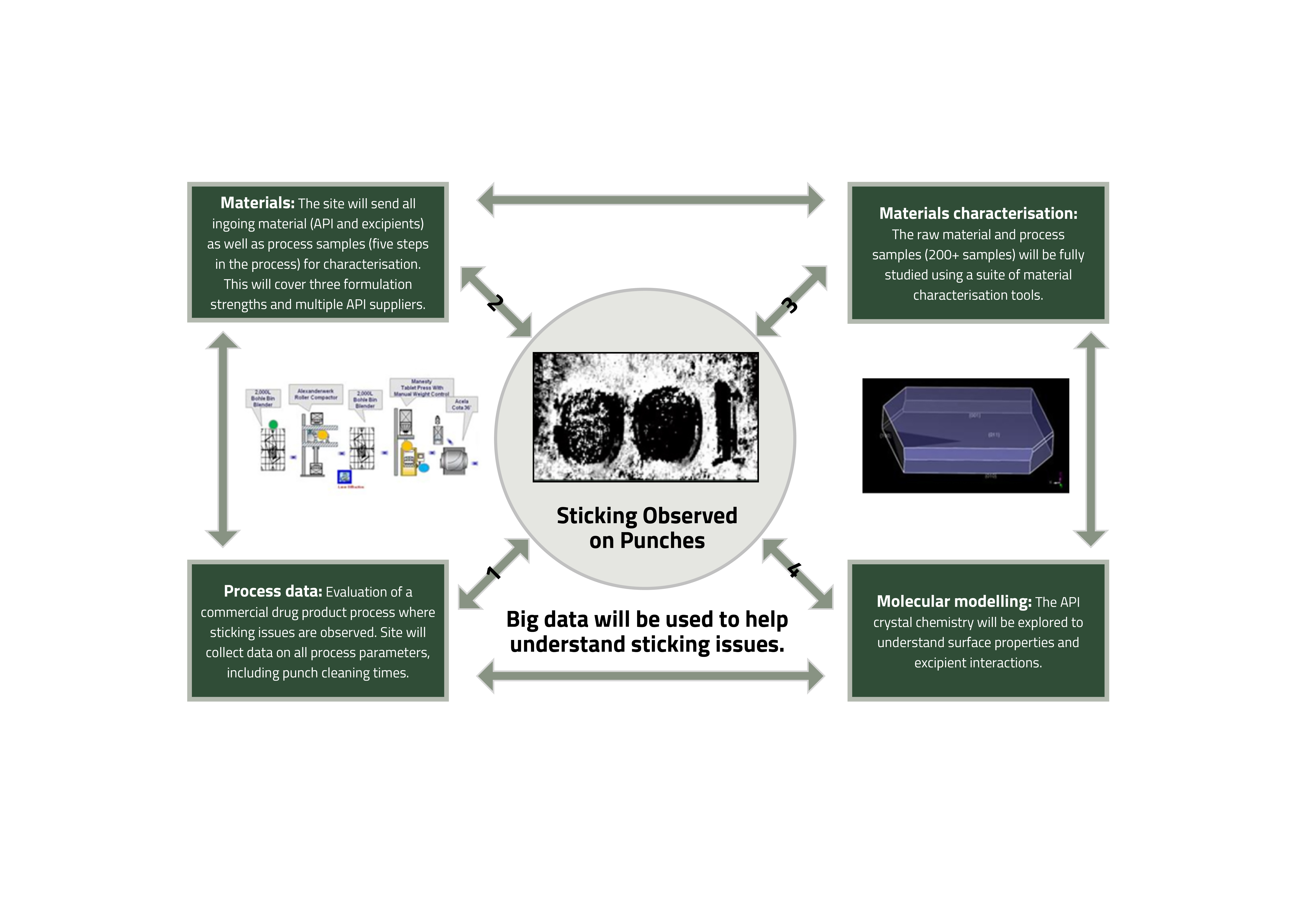
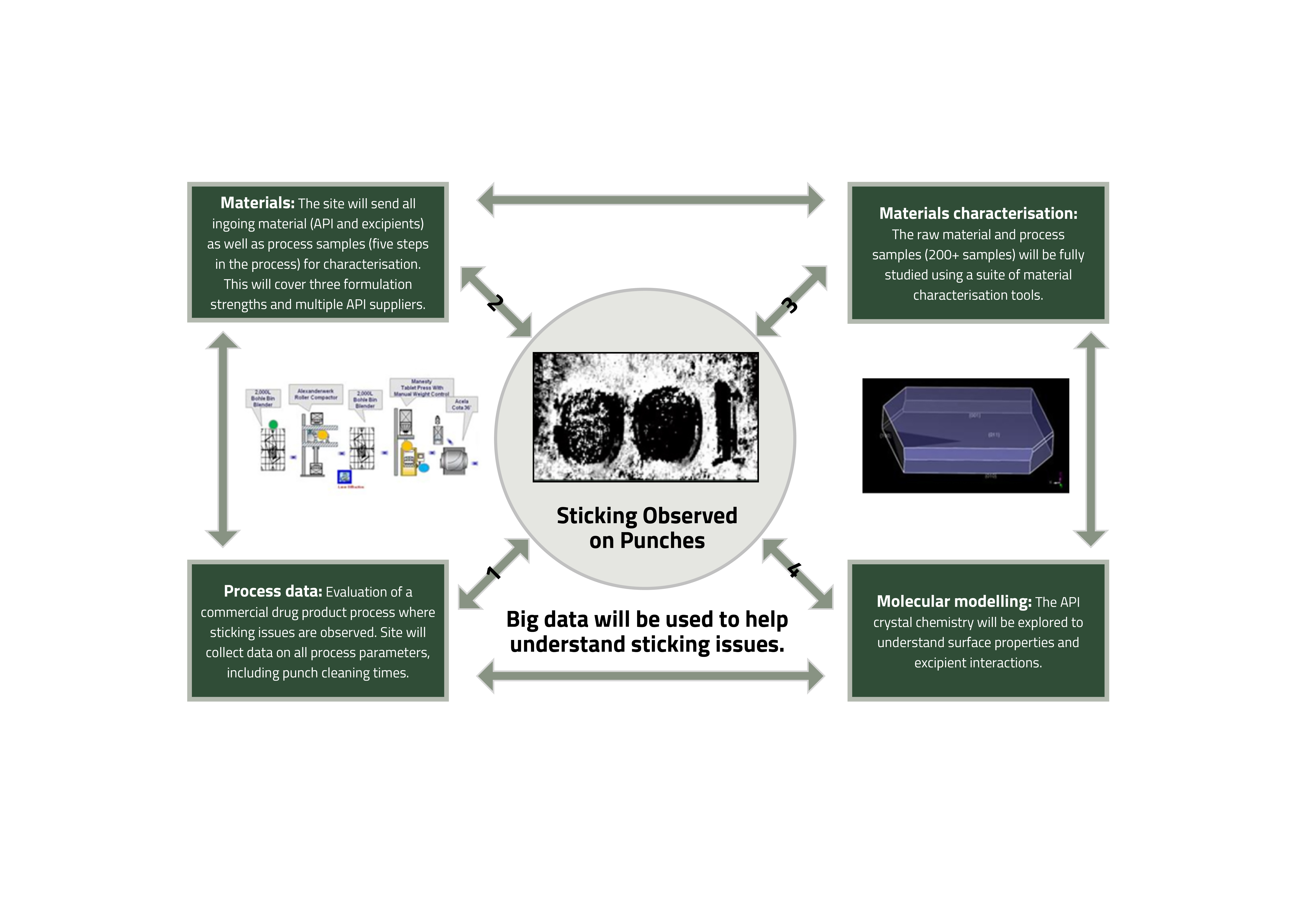
Figure 1: Linking material and processing data analysis to molecular modelling.
‘Big data’ gathering and analysis
Process data
In order to evaluate a commercial drug product process where sticking issues are observed, a manufacturing site has collected a wide range of process parameters, including punch cleaning times.
Materials characterisation
All in going material (API and excipients) have been tested using 16 characterisation techniques. This will cover three formulation strengths and multiple API suppliers, producing data that is a mixture of numerical results, patterns, traces and images. In-process samples from four sampling points for 49 batches of product are being tested using a suite of material characterisation techniques. In addition, characterisation will be completed on the final tablet.
Data analysis
A range of analytical and statistical tools are being employed, firstly to determine the similarity/ consistency of material properties of ingoing API and excipients, in-process samples and tablets across batches. The results will then be used to identify the characteristics that link through the process to the processing behaviour of the batch. For any hypothesis that relates to the API properties, the consistency of the prediction with the material modelling described below will be considered. The intention of the analysis is to deconvolute the relative importance of the material attributes and process parameters to sticking.
Material modelling
This consists of studies starting at the molecular level, examining predicted surface properties, how the surfaces may interact with excipients and the effect of crystal morphology on the relative proportions of key features. Initial work has indicated that the surfaces have different behaviours providing some key hypotheses that can be tested using the data above. In addition, modelling of the impact of solvents on the predicted morphology of the API would provide a good starting point for API crystal engineering studies, if required. Achieving these combined computational modelling activities has involved a multi-disciplinary approach including a team from the University of Leeds and colleagues from both R&D and commercial manufacturing groups at Pfizer. This type of multi-disciplinary, orthogonal approach to a computational tool is a powerful way to solve multi-factorial issues such as tablet sticking.
Example 2: from powder properties to powder flow
Continuous manufacture of oral solid dosage forms is now a reality but has not been fully optimised, and some of those “continuous” processes may require enablement by batch pre-blending. For continuous direct compression, a primary requirement is that powders flow reliably and predictably within the loss in weight feeders and mixers that lead to the mixers and press. If an API has poor flow it may have to be premixed with another component in a batch stage prior to the continuous process, a sub-optimal arrangement. A better understanding is needed of what characteristics lead to reliable flow; however, direct measurement of these characteristics often requires testing on ‘real life’ equipment, which requires kilogrammes of material to make a reliable assessment. At development costs and scales this can be a prohibitive requirement. In addition, the measurement of flow and its correlation to performance in feeders and mixers has not been fully aligned in the industry.
If an API has poor flow it may have to be premixed with another component in a batch stage prior to the continuous process, a sub-optimal arrangement.
The fundamental powder properties linked to this performance have been examined, but generally on model materials with properties significantly different from active APIs. In many cases the latter are micronised to a size of around one micron, whereas model materials may be in the hundreds of microns range, and in silico modelled systems tend to be based on single sizes or shapes. Within ADDoPT the consortium has been able to attack this challenge. The unique synergy between large pharmaceutical companies, academic groups and data centres has allowed the programme to:
- Define the characteristics of a powder that could be successfully fed in a continuous feeder, and how these can be assessed without using direct measurements in the feeder
- Agree harmonised methodologies on how to measure the fundamental properties (size, shape, surface area and surface energy) of powders, which either met, or did not meet, these criteria. This was done on state-of-the-art apparatus available at all the industrial partners
- Devote resources to making both the flow measurements and the fundamental measurements using the harmonised methods, with a significant number of materials with both acceptable and unacceptable properties
- Analyse the large body of data generated, using the data systems and capabilities of the STFC Hartree Centre, to build innovative models of powder properties likely to lead to success in a feeder.
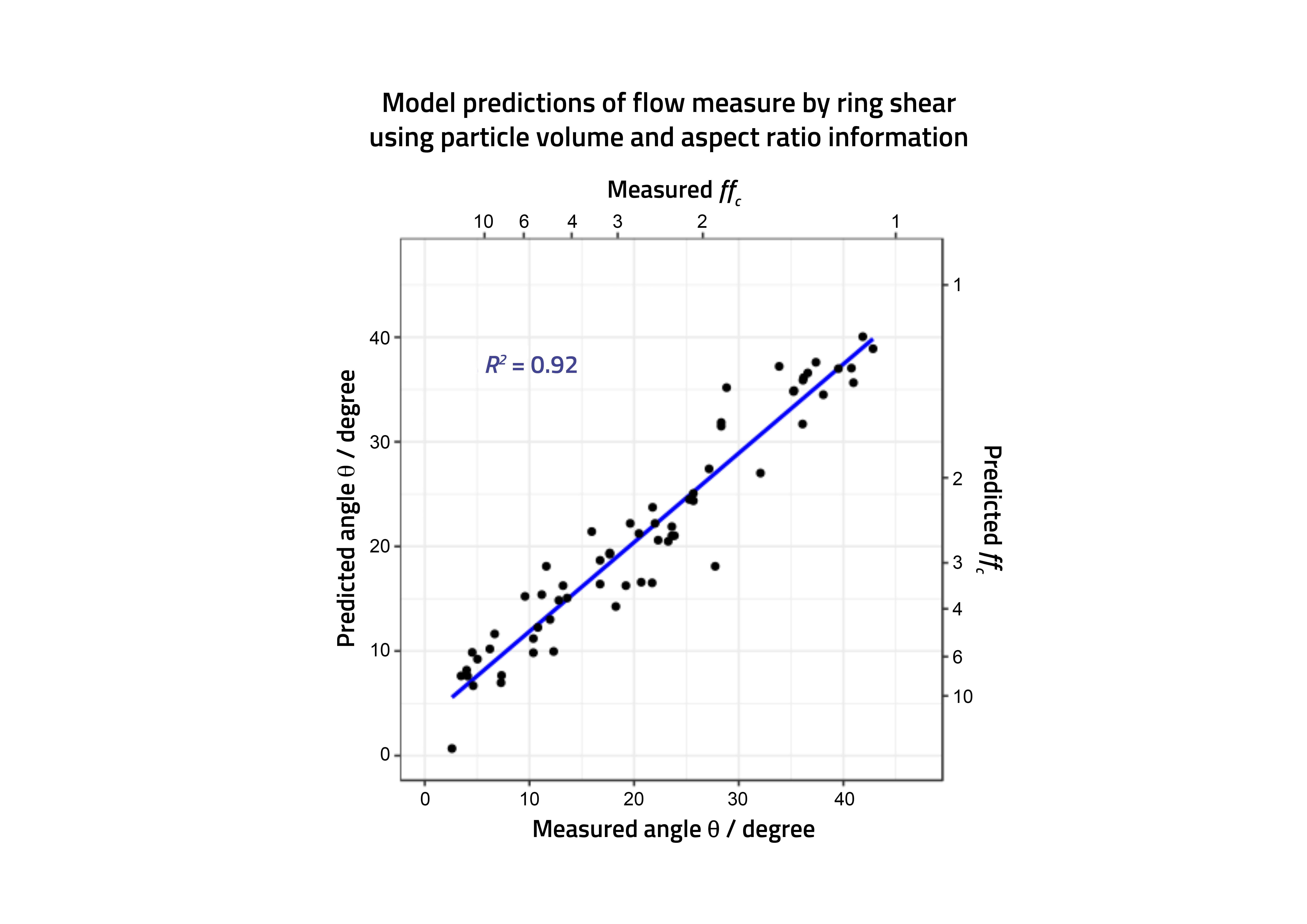
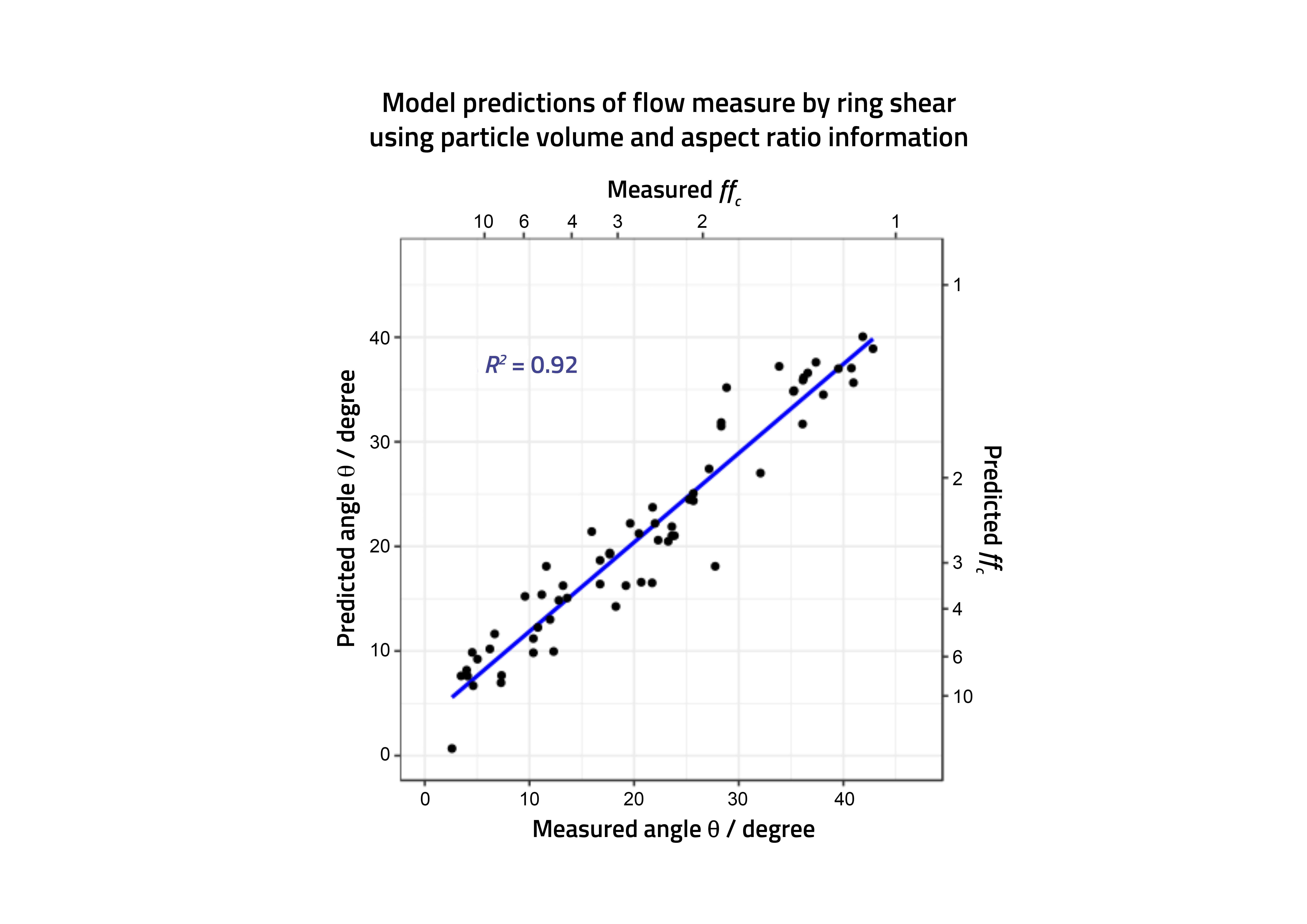
Figure 2: Actual and predicted powder performance (shear cell angles) for a range of pharmaceutical materials.
With these models available it was possible to predict, for the first time across a range of pharmaceutically relevant materials, which systems would be likely to have the necessary flow characteristics to deliver the required performance in a loss in weight feeder (Figure 2). Most importantly, this could be applied not only to actual materials where measurements were available (even on a small amount of material) but also to profile materials, the properties of which (eg, size, shape) could realistically be made, allowing the formulator to direct manufacturing colleagues to make, procure and specify suitable materials capable of performing in continuous direct compression.
Example 3: utilisation of a process model for twin screw granulation
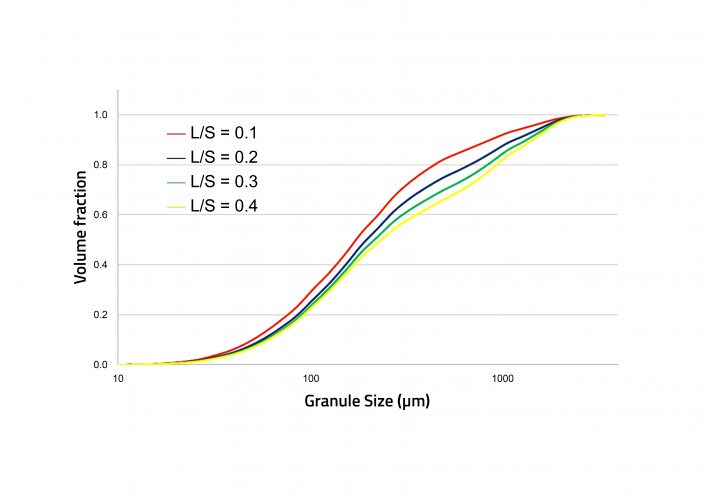
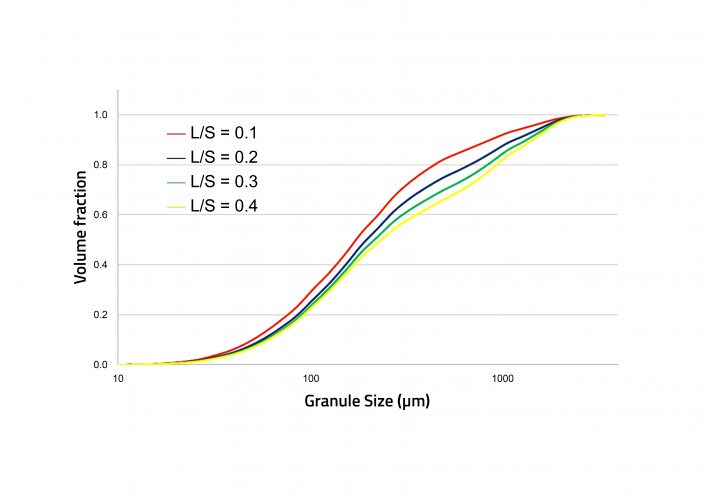
Figure 3: Simulated cumulative granule size distributions with increasing liquid-tosolid ratio (L/S).
Traditional pharmaceutical manufacturing has frequently made use of batch wet granulation processes to manufacture oral solid dosage forms.5 More recently, twin screw granulation (TSG) has gained importance as a continuous, scalable form of wet granulation with many associated benefits.6 In TSG, powdered formulation components are transported through a barrel containing two co-rotating screws, contacted with liquid and discharged into a fluid bed dryer. Typically, the layout of screw conveying, mixing and kneading elements is highly configurable and this along with other variables (powder feed rate, screw speed and liquid feed rate) leads to a large number of possible combinations. Finding an optimal design and operational space for a new formulation can potentially consume significant time and material if done in a purely experimental manner. Many parallel and competing rate processes, such as granule formation, agglomeration, breakage and consolidation, occur during wet granulation. A population balance modelling approach using compartmentalisation along the length of the TSG barrel has been developed to describe these processes. Through ADDoPT, a TSG model was implemented in gPROMS
Formulated Products, based on previously published work.7 A case study utilising a dataset generated from a proprietary pharmaceutical formulation was developed, which sought to answer the following questions:
- Is it possible to calibrate and validate a model of the TSG process that is suitably predictive for industrial use?
- Would having a process model for the TSG change the current experimentally-driven process development workflow?
Data was available from 24 experiments where liquid-to-solid ratio, screw speed and powder feed rate had been systematically varied, along with five experiments that included changes in screw configuration and liquid-to-solid ratio.
Model validation
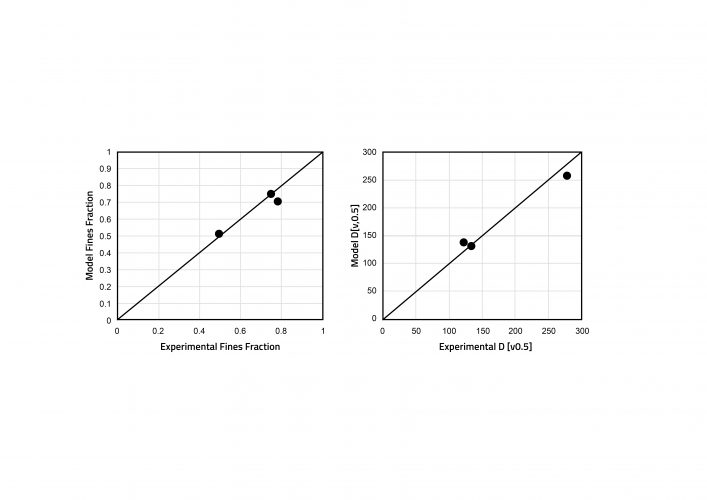
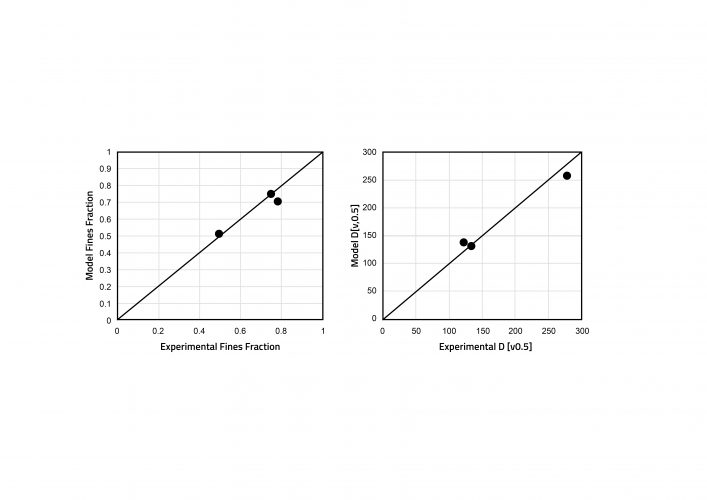
Figure 4: Parity plots showing experimental and simulated granule fines fraction and D (v0.5) for a screw
configuration of 6K elements. Model parameters were estimated from 3K and 6K6K screw configurations.
Experimental data was required to estimate rate parameters for the mechanisms occurring during TSG. Parameters were estimated for drop nucleation, breakage and layering, by fitting with dried granule size distributions. Other model inputs included the feed powder particle size distributions and bulk densities, the flowrates of powder and liquid, and the screw configuration and elements. The residence time distribution in each screw element was estimated from published data.8 Following parameter estimation, the model was found to be able to capture the influence of liquid-to-solid ratio on the granule size distribution. In the experiments, an increase in liquid-to-solid ratio led to an increase in granule size, which was also captured by the model. In the dataset containing different screw configurations, the configurations were made up of a single block of the kneading elements (3K), a single block of six kneading elements (6K) and two blocks of six kneading elements (6K6K). All remaining elements were conveying. The 3K and 6K6K experiments were used for parameter estimation and the 6K experiments for model validation. Figure 4 shows an excellent level of prediction for the 6K experiments.
Utilisation of a process model
Rate parameters were estimated using the full 24 experiment dataset and then with a subset of five experiments. The simulation results showed comparable levels of prediction for variation of granule size with liquid-to-solid ratio. This shows how use of the process model could be used to reduce the experimental burden of process development. A smaller number of scoping experiments could be used to build the model, which can then be used to help identify a suitable operating space. Exploring different screw configurations can be particularly time consuming and costly in material wastage due to both the large number of possible combinations and the associated equipment dismantling and reassembly. The results from the model show it may be possible to use experiments from just a couple of screw configurations to estimate the relevant model parameters that can then be used to explore other potential screw configurations in silico. Overall, the case study demonstrates how development of a complex process can be made more efficient by an integration of digital design with development workflows. In this case, optimisation of the process around granule size was demonstrated. Further development of downstream unit operation and product models to enable simulation of the tablet properties will further aid the application of digital design to the pharmaceutical development process.
Conclusions
These case studies exemplify both the challenges and opportunities that exist in bringing together mechanistic materials and process modelling techniques with broader statistical analysis as part of the digital transformation in pharmaceuticals development and manufacturing. In addition, they illustrate the importance of an ecosystem that brings together colleagues from academia, industrial end-users and solution providers. This approach is being extended across further industrial case studies due for completion in 2019 (the final year of the ADDoPT project).
Biographies
development and application of PSE’s technologies for drug product manufacturing processes.
References
- ADDoPT project information. Available at: https://www.addopt.org Accessed 23rd April 2019.
- McCubbin I. UK BioIndustry Association guest blog, 15th February 2017. Available at: https://bioindustryassociation.wordpress.com/2017/02/15/guest-blog-handing-over-the-helm-of-mmip/ Accessed 23rd April 2019.
- Hooper D, Clarke F C, Docherty R, Mitchell J C, Snowden M J. Effects of crystal habit on the sticking propensity of ibuprofen – a case study. J. Pharm. 2017 Oct 5; 531(1):266-275.
- Paul S, Taylor L J, Murphy B, Krzyzaniak J, Dawson N, Mullarney M P, Meenan P, Sun C C. Mechanism and Kinetics of Punch Sticking of Pharmaceuticals. J Pharm Sci. 2017 Jan;106(1):151-158.
- Leane M et al. Manufacturing Classification System in the real world: factors influencing manufacturing process choices for filed commercial oral solid dosage formulations, case studies from industry and considerations for continuous processing. Pharm. Dev. Technol. 23, 964–977 (2018).
- Seem T C, et al. Twin screw granulation — A literature review. Powder Technol. 276, 89–102 (2015).
- Barrasso D, El Hagrasy A, Litster J D, Ramachandran R. Multi-dimensional population balance model development and validation for a twin screw granulation process. Powder Technol. 270, Part, 612–621 (2015).