Using deep learning to predict drug metabolites and toxicity
Posted: 6 October 2020 | Hannah Balfour (European Pharmaceutical Review) | No comments yet
Researchers have developed Metabolite Translator, a system that can predict what the in vivo metabolites of a drug could be in order to identify its potential toxicity.
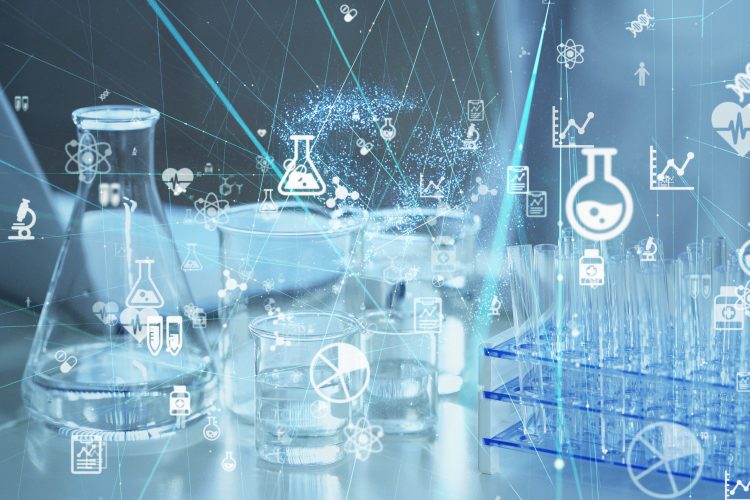
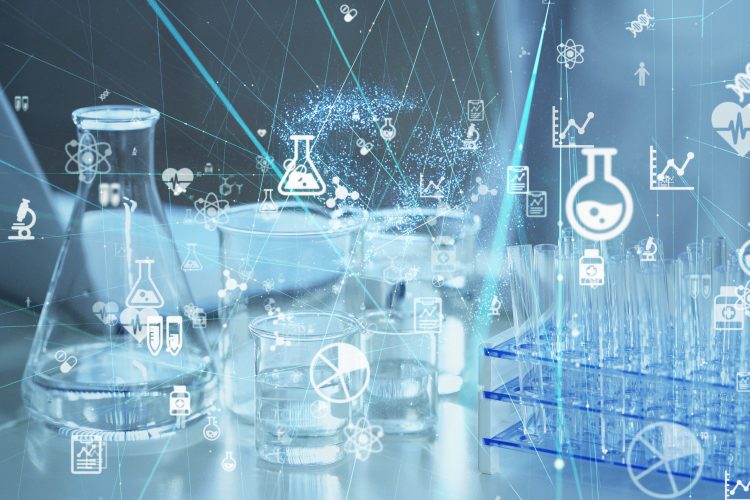
Computer scientists have leveraged deep-learning methods to develop Metabolite Translator, a computational tool that predicts what metabolites will result from interactions between enzymes and small molecules such as drugs.
The tool, created at Rice University’s Brown School of Engineering (US) in the lab of Lydia Kavraki, was designed to enable pharmaceutical companies to better understand how their experimental drugs will perform in vivo in the human body.
Using deep-learning methods and the availability of massive reaction datasets, the team developed a system which they say is not constrained by rules that companies use to determine metabolic reactions, opening a path to novel discoveries.
“When you are trying to determine if a compound is a potential drug, you have to check for toxicity. You want to confirm that it does what it should, but you also want to know what else might happen,” said Kavraki, the Noah Harding Professor of Computer Science, a professor of bioengineering, mechanical engineering and electrical and computer engineering and director of Rice’s Ken Kennedy Institute.
The researchers trained Metabolite Translator to predict metabolites by pre-training it on 900,000 known chemical reactions and then fine-tuning its capabilities with data on human metabolic transformations. As a result, they say it can predict metabolites through any enzyme.
Metabolite Translator is based on SMILES (simplified molecular-input line-entry system), a notation method that uses plain text to represent chemical molecules, rather than diagrams.
To validate Metabolite Translator, they used it to analyse the SMILES sequences of 65 drugs and 179 metabolizing enzymes, then compared the results with those produced by several other predictive techniques. Though Metabolite Translator was trained on a general dataset not specific to drugs, the researchers said it performed as well as commonly used rule-based methods specifically developed for drugs. It also identified enzymes that are not commonly involved in drug metabolism and were not detected by existing methods.
Kavraki concluded: “Using a machine learning-based method, we are training a system to understand human metabolism without the need for explicitly encoding this knowledge in the form of rules. This work would not have been possible two years ago.”
The paper was published in Chemical Science.
Related topics
Artificial Intelligence, Drug Development, Drug Safety, Informatics