AI uses patient data to administer less toxic treatments
Posted: 13 August 2018 | European Pharmaceutical Review | No comments yet
The researchers found that doses were often missed, with some treatments being scheduled twice a year instead of monthly…
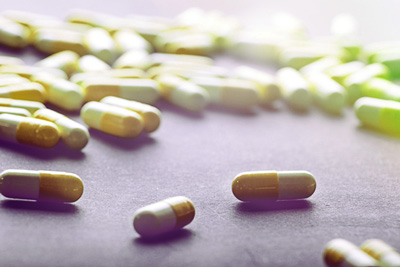
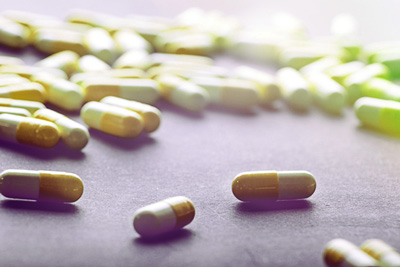
Using simulated trials, researchers at Massachusetts Institute of Technology (MIT) designed novel machine learning techniques to improve the quality of life for patients with glioblastoma.
Glioblastoma is the most aggressive form of brain cancer and appears as a malignant tumour in the brain or spinal cord, with prognosis for patients being no more than five years.
The researchers aimed to produce dosing regimens specific for each patients in order to make drugs less toxic but still effective. The model is powered by a ‘self-learning’ machine learning technique, using treatment regimens currently used and adjusting the doses, eventually identifying an ‘optimal treatment plan’ with the lowest potency and frequency of doses that still reduces tumour size similar to that of traditional treatments.
The researchers found that doses were often missed, with treatments being scheduled twice a year instead of monthly. The treatment cycle still maintained the tumour size reduction.
“We kept the goal, where we have to help patients by reducing tumour sizes but, at the same time, we want to make sure the quality of life – the dosing toxicity – doesn’t lead to overwhelming sickness and harmful side effects,” says Dr Pratik Shah, a principal investigator at the Media Lab, and supervisor of this research.
A combination of drugs was used for the trial, such as temozolomide (TMZ), lomustine and vincristine (PVC) for the Reinforced Learning (RL) technique that was used to ‘teach’ the machines the outcome desired, with the artificial agent receiving ‘rewards’ or ‘penalties’ for each action taken.
To train the model, around 20,000 ‘trial-and-error’ runs were conducted for each of the 50 patients, allowing the model to ‘learn’ the parameters of optimal regimens. The team also used medical data for each patient to train the model to treat each patient individually.
“We said [to the model], ‘Do you have to administer the same dose for all the patients?’ And it said, ‘No. I can give a quarter dose to this person, half to this person, and maybe we skip a dose for this person.’ That was the most exciting part of this work, where we are able to generate precision medicine-based treatments by conducting one-person trials using unorthodox machine-learning architectures,” Dr Shah said.
The study was presented at the Machine Learning for Healthcare conference 2018 at Stanford University.
Related topics
Analytical techniques, Artificial Intelligence, Clinical Development, Clinical Trials, Drug Development, Research & Development (R&D)