Integrating preclinical data into early clinical development
Posted: 3 September 2012 |
One of the important goals in preclinical and early clinical drug development is to reduce attrition rates and to improve our ability to pick winners and drop potential loser drug candidates. By being able to efficiently translate preclinical data and observations into possible clinical outcomes, one can make the drug development process more cost-effective. Identifying preclinical models – in silico, in vitro, in vivo – or assays that can best predict clinical observations is not trivial. It requires understanding of preclinical-to-clinical correlations and the success of translational science may vary depending on the therapeutic area where one is working. For example, anti-infectives or cancer therapeutic areas have validated biomarkers which can be useful in selecting the right drug candidate in early drug development…
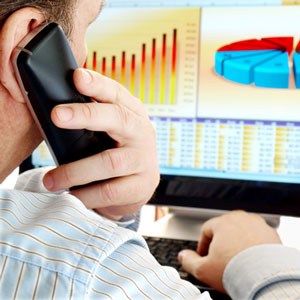
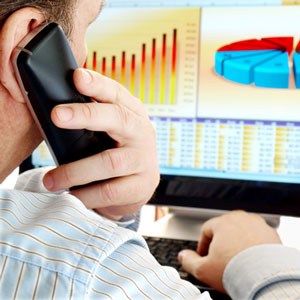
One of the important goals in preclinical and early clinical drug development is to reduce attrition rates and to improve our ability to pick winners and drop potential loser drug candidates. By being able to efficiently translate preclinical data and observations into possible clinical outcomes, one can make the drug development process more cost-effective. Identifying preclinical models – in silico, in vitro, in vivo – or assays that can best predict clinical observations is not trivial. It requires understanding of preclinical-to-clinical correlations and the success of translational science may vary depending on the therapeutic area where one is working. For example, anti-infectives or cancer therapeutic areas have validated biomarkers which can be useful in selecting the right drug candidate in early drug development.
However, it is extremely challenging to translate the preclinical pharmacological models into clinical signals for drugs in the neuroscience area. Selection of drug candidates with optimal pharmacokinetic (PK) parameters in early drug discovery is essential for convenient dosing regimens and effective therapy in patients. During drug discovery, considerable resources are required to assess the PK properties of potential drug candidates via in vivo and in vitro preclinical studies.
Traditionally, the pharmaceutical industry has relied on empirical approaches such as allometry for predicting human PK before first-in-man studies. However, in recent years, there has been a growing interest in methods that improve predictions of human PK via mechanistic understanding of the underlying processes impacting a drug’s PK disposition.
First-in-Human dose selection
One of the important aspects of translation of preclinical data is in selection of clinical dose for estimation of a first-in-human (FIH) study. Selection of the starting dose in humans is a complex process, as it must fall within an optimal window. The starting dose must be low enough to be safe but high enough to avoid excessive dose escalations, which are costly and time-consuming. Given the importance of safe guarding human subjects, regulatory guidance documents have been issued by the Food and Drug Administration (FDA) and the Committee for Medicinal Products of the European Medicines Agency (EMEA). The intent of these guidance documents related to first-in-human starting dose is to provide a robust scientific rationale for the dose selection to avoid toxicity in the first dose cohort. The most widely used method for FIH dose estimation is based on no observable adverse effect levels (NOAELs) in multiple species.
NOAELs are determined in relevant animal studies, normalised to body surface area and then extrapolated to human equivalent doses (HEDs). The HED from the most appropriate species is then divided by a safety factor to generate the maximum recommended starting dose (MRSD) in humans. However, the NOAEL-based approach relies on a somewhat arbitrary safety factor to estimate the starting dose, and the method is very conservative for FIH dose estimation. In contrast, pharmacokinetic-guided approaches provide a more mechanistic rationale and are becoming more widespread in their use across many pharmaceutical companies and institutes. An alternative to scaling the NOAEL dose involves the use of the pharmacologically active dose (PAD). This approach may be particularly useful when the effects in humans may arise from exaggerated pharmacology (i.e., anti – coagulants, vasodilators and biologics). Scaling the PAD to a HED may give rise to an estimate that is lower than the MRSD derived from the NOAEL. In particular, demonstration of a robust dose–response relationship is needed and consideration of the translation of the animal response to humans is integral to the use of PAD.
Integration of PK/PD information in clinical dose selection
In the aftermath of the TGN1412 case, the EMEA issued a guidance document on first-in-human dose selection which emphasised identification of factors influencing risk. In situations where the translation of toxicological data from preclinical species to human is uncertain, additional considerations are needed. Knowledge of the mode of action, the nature of the pharmacological target and relevance of animal models are additional considerations in assessing the potential for severe adverse reactions. The concept of the minimum anticipated biological effect level (MABEL) approach was introduced for medicinal products which fall into the high risk category. The MABEL approach integrates pharmacology and toxicology information into the selection of the FIH starting dose. In particular, use of PK/PD models is suggested to integrate available in vitro and in vivo information. The specific types of information to be integrated include (a) in vitro studies to characterise binding and affinity to the pharmacological target, (b) in vitro concentration–response evaluation in test species and human cellular systems, and (c) concentration–response in animal models. Several areas of the FIH study design can be enhanced with PK/PD modelling. Effective integration of the product of PK/PD predictions is an evolving area. As defined by the regulatory guidance, a safe starting dose should minimise the risk to healthy human subjects by avoiding concentrations that may produce profound pharmacological effects and toxicity. Combining both the PK and PD of a compound can help to avoid starting at unnecessarily low doses which would not be expected to offer any therapeutic benefit. PK/PD modelling can help to define the most appropriate concentration range to study drug response(s), inform the choice of dose escalation scheme through translation of preclinical dose-concentration-response, define doses associated with concentrations that may give rise to secondary pharmacology signals (such as QTc or blood pressure), and avoid doses that may exceed NOAEL exposure margins for healthy volunteer studies. Several study design elements can be supported with PK/PD model predictions. These elements include the selection of sample collection times for PK observations, pharmacodynamic observations, appropriate washout times, and the duration of study.
While the prediction of a starting dose is mainly driven by safety, the prediction of efficacious human doses is a complex process as biopharmaceutical, pharmacokinetic and pharmacodynamic properties must be projected from preclinical data. While the maximum recommended starting dose for FIH trials is covered by Food and Drug Administration guidance, the prediction of efficacious doses is performed at early and late development stages for several reasons, including (a) selection of clinical candidate back-up compounds with improved ADMET properties, (b) identification of safe and efficacious doses for FIH trials, (c) identification of formulation development risks, and (d) estimation of drug supply needed for toxicokinetic and FIH trials.
In the discovery setting, PK/PD models are being developed and used to integrate in vitro and in vivo data to predict efficacious plasma concentrations as part of the candidate selection process during lead optimisation. This additional effort, while not always a requirement, may help to prioritise lead molecules with respect to PK/PD properties, give early information about dosing requirements prior to or in parallel with the collection of safety data and support the selection of optimal dosing schedules.
Prediction of human pharmacokinetics from preclinical data
Accurate prediction of human pharmacokinetics (PK) is a vital part of drug discovery and contributes to internal compound progression decisions as well as therapeutic dose assessment. Huge efforts in both academia and industry have focused on developing reliable methods to predict human absorption, distribution and clearance as well as simulating accurate plasma concentration–time profiles from preclinical in vitro and in vivo data. Over the past few decades, many empirical and physiological approaches have been developed for quantitative prediction of human PK properties from preclinical data. In this context, different types of methods including allometry, in vitro–in vivo extrapolation (IVIVE), and physiologically based pharmacokinetic modelling (PBPK) are currently used within the industry to predict PK properties from preclinical data and simulate plasma concentration-time profiles in order to derive and guide dose selection for clinical studies. These predictive approaches typically require in vivo preclinical data, in vitro metabolism and disposition data obtained from animal and human tissues, and/or physicochemical parameters of the drug compounds.
Allometric scaling is one of the most widely used tools for the prediction of human pharmacokinetic parameters. Most interspecies scaling predictions are based on the combination of empirical approaches and in vivo animal data. As with all approaches that are empirically based, allometry is not without shortcomings. The allometric scaling method based on simple bodyweight (simple allometry) using in vivo data generated from the preclinical species frequently fails to accurately predict human pharmacokinetics. Over the years, several different correction factors, both empirical and mechanistic in nature, have been proposed as global solutions to this difficult problem. The various approaches that have been proposed to improve the accuracy of pharmacokinetic parameter predictions using allometric scaling include the ‘rule of exponents’ the ‘unbound oral clearance (CL/F) approach’, the ‘unbound fraction (fu) corrected intercept method’ (FCIM) and ‘correction for in vitro CL’.
In the last decade or so, a more mechanistic physiologically-based pharmacokinetic modelling (PBPK) approach has been increasingly used as a tool to improve a drug development process. PBPK models employ in vitro and/or in silico data inputs to predict concentration versus time profiles in plasma and tissues. The physiological framework provided by a PBPK model can integrate all input data and aid in understanding PK characteristics of potential drug candidates. An important differentiating feature is that, unlike empirical approaches, PBPK based approaches are derived from an understanding of the underlying anatomy and physiology of the species. Hence, the basic model equations representing anatomy and physiology that are shared in common are not drug specific but common to mammalian species, thereby facilitating interspecies scaling.
PBPK models divide the body into anatomically and physiologically meaningful compartments that integrate system specific properties (e.g. blood flow, organ mass) and drug properties (e.g. binding affinities, permeability) with a structural model reflecting the anatomical arrangement of the tissues connected by perfusing blood (Figure 1).
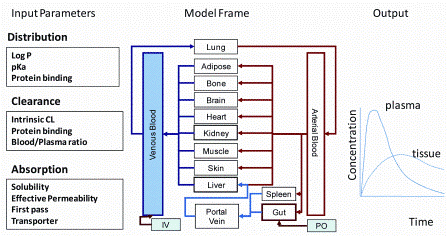
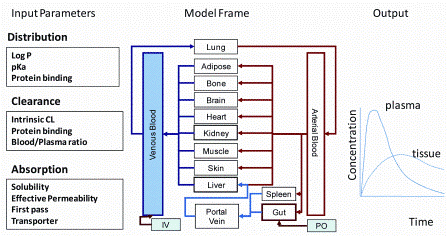
Figure 1: Components of PBPK modelling approach
These models use physiologically relevant parameters describing absorption, distribution, metabolism and excretion (ADME) to predict the overall pharmacokinetic (PK) profile of a compound. The use of PBPK models has increased significantly in recent years, and reports of successful retrospective application with large industrial data sets are forthcoming. An important aspect of PBPK modelling for compounds cleared primarily by the liver is the application of IVIVE methods to predict in vivo clearance from in vitro metabolic data. The utility of IVIVE for hepatic metabolic clearance has been well established in the literature and is developing to improve poor predictions by incorporating hepatic uptake and/or biliary excretion.
Until relatively recently, the application of PBPK modelling in support of drug discovery and early development was somewhat limited due to the resource-intensive ADME data required to fully populate PBPK models and the mathematical complexity of the model itself. However, advances in in silico / in vitro-based prediction tools, in particular those for absorption, distribution and hepatic clearance, have enabled the use of PBPK modelling at an earlier stage of drug discovery. In addition, the emergence of software tools, such as GastroPlus™ (Simulations Plus, Lancaster, CA), PK Sim® (Bayer Technology Services, Leverkusen, Germany) and the Simcyp® simulator (Simcyp, Sheffield, UK) allow for a broader use of PBPK models. These specialised software packages eliminate the need to build complex PBPK models from scratch and greatly reduce the amount of data that need to be imputed by the user. Together, the advances in prediction and software tools have brought PBPK modelling and simulation forward as a significant contributor in the drug design process and have enabled more-informed decision making, particularly during the clinical candidate selection process.
Advances in the development of physiologically based prediction tools to assess the required input parameters such as tissue-plasma partition coefficient, intrinsic Clearance (CLint), and rate and extent of absorption solely from in vitro data, has now greatly extended the application of PBPK. Unlike empirical modelling, PBPK models are not purely descriptive but may also account for the mechanistic basis of the observed data. For example, prediction failures with preclinical species can guide experimental efforts to understand the compound’s properties and achieve a higher level of prediction accuracy before first-inhuman studies. Sometimes more complex models that take into account factors such as involvement of active transporters may be required. The validation of model assumptions in a preclinical PBPK model such as a rat or dog may help in mechanistic understanding of pharmacokinetic behaviour of a drug before first-in-human studies.
PBPK based approaches are now commonly being used in answering critical questions in early and late phases of drug development. The application of PBPK modelling may vary from early discovery to full clinical development of the compound depending on the questions posed during the drug’s development pathway. Some of the common uses are listed below:
- To estimate compound’s physico-chemical properties and associated PK parameters based on chemical structure (such as Vss, plasma protein binding, effective permeability, solubility, Log P, pKa etc.)
- in vivo drug disposition in plasma and target tissues
- Human PK prediction for FIH study dose selection
- Drug-drug interaction potential
- Regional drug absorption and formulation evaluation
- Drug disposition in special populations
- Answering ‘what if questions’ (e.g., what if the particle size is reduced or dissolution profile of compound is changed, what will be its effect on PK properties)
Conclusions
Drug development is an information-rich endeavour, and it is the role of researchers to ensure that they are leveraging on important findings from internal and external sources. This can be achieved by taking informed decisions about compounds in the pipeline, thus helping to improve the likelihood of success as candidate drugs move into clinical develop – ment. Translation needs to happen in both directions: translating from preclinical studies to patient studies, and also in reverse direction by providing constant feedback to the preclinical team based on our clinical findings. The biggest challenge today is indeed finding or accessing all the relevant and necessary data and information, and more often, we spend more time getting to the data than using it. Maintaining an on-going dialogue between preclinical and clinical scientists so they can improve the predictivity of preclinical models is challenging, nevertheless is the key factor. Because all of us within the industry are dealing with similar issues, several consortia have come together to join efforts and create larger datasets. With large enough datasets, researchers can identify more of the factors that contribute to the success or failure of a drug, or a particular class of drugs, thus gaining better insights.
In recent years there has been a significant progress in model based drug development efforts. With the advancement of science and shrinking budgets for performing expensive clinical studies, more and more pharmaceutical companies are relying on modelling and simulation before embarking upon any clinical study. There has been a tremendous support and encouragement from regulatory authorities as well on this front. Modelling and simulation can be a very promising and effective approach, however one should recognise the fact that without proper mechanistic insight into any model considerations, it can act as a double edged sword. Hence proper model verification and physiological rationale in model assumptions is of utmost importance prior to using this wonderful tool for any go-no go decisions.
Allometric scaling is simple to use, however due to its empirical nature, physiologically based prediction methods are increasing in popularity and use because of their mechanistic rationale. Unlike empirical estimation methods, these model-guided approaches have a mechanistic rationale to understand the effect of physiological variables or disease status on pharmacokinetic parameters. Dose selection for clinical studies should follow a prudent approach using all the available information at hand irrespective of the methodology applied. A smartly designed clinical development program will require the knowledge of the compound PK characteristics, the PK/PD link and safety tolerability data for smarter dose escalation schemes without compromising the safety of the subject.
Reference and suggested readings
- FDA, Guidance for industry-estimating the maximum safe dose in initial clinical trials for therapeutics in adult healthy volunteers. 2012
- EMEA/CHMP. Guidelines on strategies to identify and mitigate risks for first-in-human clinical trials with investigational medicinal agents
- Clinical Trial Simulations – Applications and Trends. AAPS Advances in the Pharmaceutical Sciences Series 1, 2011
- Sinha VK, Mackie Claire. Dose Selection Based on Allometric Approaches, AAPS J, 2012 (submitted article)
- Rowland M, Peck C, Tucker G. Physiologically based pharmacokinetics in drug development and regulatory science. 2011 Ann Rev Tox & Pharm 51: 45-73