Ensuring patient safety during clinical trials; translation to preclinical drug discovery
Posted: 3 September 2012 |
Ensuring patient safety during clinical trials is of paramount consideration with stringent monitoring built into trials (and beyond) and the design and interpretation of safety outcomes subject to a large amount of regulation. As a result, it is rare for clinical trials to produce extreme adverse drug reactions but it is also quite common for new medicines to fail in clinical testing due to unacceptable patient safety within a given indication. This is because once a new drug reaches clinical testing, its safety profile is already ‘locked in’, and clinical testing can only discover issues that already exist. The ideal way to ensure the safety of patients is to only progress new medicines into clinical testing which do not have unacceptable safety or tolerability issues. However, to reach this ideal means using learning in the clinic to influence design and development in the laboratory. In this short article, we discuss the practical challenges in doing this and in ‘translating’ patient safety observations such that they can impact on drug design and early development…
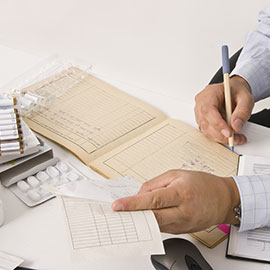
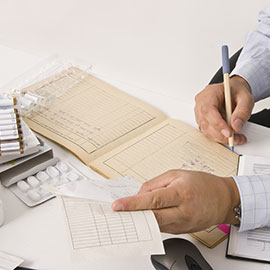
Ensuring patient safety during clinical trials is of paramount consideration with stringent monitoring built into trials (and beyond) and the design and interpretation of safety outcomes subject to a large amount of regulation. As a result, it is rare for clinical trials to produce extreme adverse drug reactions but it is also quite common for new medicines to fail in clinical testing due to unacceptable patient safety within a given indication.
This is because once a new drug reaches clinical testing, its safety profile is already ‘locked in’, and clinical testing can only discover issues that already exist. The ideal way to ensure the safety of patients is to only progress new medicines into clinical testing which do not have unacceptable safety or tolerability issues. However, to reach this ideal means using learning in the clinic to influence design and development in the laboratory. In this short article, we discuss the practical challenges in doing this and in ‘translating’ patient safety observations such that they can impact on drug design and early development.
The safety of the patient is a paramount consideration during the development and clinical testing of new drugs. Early clinical trials are set up to carefully consider the safety and tolerability of new pharmaceuticals and patient monitoring for safety continues throughout the later clinical testing phases and beyond. Prior to this, new pharmaceutical agents are subjected to a battery of preclinical tests and must overcome strict safety hurdles before a single patient receives a dose.
Appropriate safety is also a key regulatory consideration and demonstrating an acceptable safety profile for a new medicine is a prerequisite for pharmaceutical regulation. Although such extreme adverse drug reactions (ADRs) as demonstrated during the phase I testing of TGN14121 are luckily very rare, patient safety concerns account for a significant number of candidate drug failures during clinical testing as well as drug withdrawals or significant limits on drug use post-marketing2,3. Such outcomes can be seen as a success of our current approaches to monitoring patient health but they also highlight the challenges of translating safety from preclinical studies to patients and from trial populations to the ‘real world’ and how learning gained in the clinic influences the discovery and preclinical development of new drugs.
Discovered in the laboratory and tested in the clinic
The ideal way of ensuring patient health in clinical trials is to only progress molecules into clinical testing that have an acceptable patient safety profile. The reality is though that we may progress molecules with patient safety issues into clinical testing for two reasons; firstly, drug discovery and early development occurs in the laboratory and is dependent on the use of models and screens to define safety and efficacy profiles, models and screens that are incomplete in their ability to detect and predict all patient safety issues, and secondly because there is no better alternative and there is a significant unmet medical need that makes the associated risk acceptable. However, once a drug reaches clinical testing it is too late to influence its design; any safety issues are present, we just may not know what they are yet. So influencing the development of new medicines to reduce their potential of having an unacceptable patient safety profile requires us to evolve preclinical safety approaches, which in turn means effective learning (‘back translation’) from clinical trials.
Preclinical safety
Preclinical safety can be roughly divided into having two broad purposes; the first is to influence target selection and the ‘design, make, test’ (DMT) cycle of pharmaceutical development to minimise as far as possible the likelihood of a new drug having an unacceptable safety profile and the second is to provide a comprehensive nonhuman safety testing package for candidate drugs that are delivered from this process prior to first time in human (FTIH) testing. This latter activity can be considered as a set of safety ‘quality control’ checks that are used to establish any overt dose-related toxicities associated with a candidate drug in a number of non-human species. These are used to set the margins, monitoring and constraints for early clinical testing. These tests are usually only performed on candidate drugs at the end of their development phase, are subject to significant regulation and are in general built upon a range of pathological and functional examinations looking for any drug-related effects on key organs or physiological systems4. However, by this phase of drug development, design choices have been made and so these safety tests do not themselves influence a drug’s development per se, except to prevent further progression of those candidates that are demonstrated to have an unacceptable safety profile. If we therefore wish to try and develop safer drugs then it has to be through influencing the stages prior to comprehensive non-human testing i.e. target selection and the DMT cycle, in other words connecting the patient safety outcomes back to the earliest phases and decisions in research and development.
Influencing target selection
The choice of target for a new drug discovery and development project is the single most important decision taken in that project; it is the project! Simplistically it might be thought that the easiest way of back-translating adverse patient safety finds to influence these decisions would be through exclusion of the targeted mechanism of these failed trials. Thus, we would generate a growing list of ‘bad’ targets / mechanisms and hence protect patients from future ADRs by the simple expedient of not developing drugs to these targets. However, taking this approach is rarely appropriate because it ignores two important confounding features; the patient context and the pharmaceutical intervention used.
For any new therapy, there is always a consideration of the balance of benefit of treatment with the risk to the patient. A key component in this balancing act is the disease being treated, so what would be an acceptable safety risk in the treatment of life-threatening indications such as cancer or sepsis would be unacceptable in a chronic one like obesity or mild asthma. As a result, simply stopping progression of drug development against a target based on unacceptable ADRs in one patient population may actually prevent the development of an effective (and acceptably safe) medicine in another one. The second problem in taking a ‘bad target’ approach to the translation of clinical safety findings is that ADRs are produced in response to a specific drug and we infer any potential target-related issue based on an assumption of specificity of the agent. However, it is not always a safe assumption that the ADR is driven through the ‘primary’ target (the one to which the drug was developed) as even highly ‘selective’ pharmaceuticals may actually have significant potency against other targets (see for example Norris et al5). Alternatively, the drug may have other properties, such as forming reactive metabolites, and it is these ‘off-target’ or ‘secondary’ effects that are the causes of patient ADRs6. Ultimately, the complexity of the actual impact that a drug has on the physiology of a patient means that it is sometimes difficult to easily back translate an inference of target involvement in a patient safety response.
Influencing DMT
The DMT describes the iterative process of candidate drug development where new molecules are designed, synthesised and then tested in a range of preclinical models and screens, the results of which are then used to influence the next round of design. In order for safety to be a realistic consideration during DMT, safety screens and models need to be appropriate to the timelines of the DMT cycle in order to effectively influence it. These cycle times may be measured in days or weeks and demand the ability to test large numbers of compounds in parallel and so, even putting aside the ethical concerns of animal usage, it is for this practical reason that simply deploying non-human comprehensive safety testing earlier in DMT is not possible. As a result, many of the screens used in DMT are either in vitro or in silico (computational) models and developing these in order to predict and ‘screen-out’ a particular patient safety concern requires an understanding of the mechanisms / processes that contribute to or cause a particular ADR. Unfortunately, gaining this insight starting from a patient safety observation can be a significant undertaking and may lag many years behind the observation itself. For example, although thalidomide was withdrawn from the market in 1962, it was not until 2009 that an explanation for its severe effects on embryo development was published7 with the molecular target for these effects only being identified in 20108. Thalidomide also highlights the problem of ‘applicability’ i.e. having identified the molecular mechanism for thalidomide-induced toxicity, is this mechanism one that is broadly applicable to other chemical series or is it specific to thalidomide and its close analogues? A second problem could be termed ‘doability’; is it practical to deploy learning from the clinic as a preclinical screening tool? For example, the CB1 antagonist Rimonabant was removed from the market for treatment of obesity due to unacceptable psychiatric effects, notably depression9. How could such an observation be back translated to in vivo models let alone in vitro or in silico models that might be deployed during DMT? The problems of ‘doability’ and ‘applicability’ together with the potentially long timelines to gaining a mechanistic under standing summarise the major challenges in translating a patient ADR into useful knowledge and practical application in early drug discovery and development.
One of the most effective ways of influencing DMT is through the development of in silico models because such models can be deployed directly during the design phase, prior to any compound synthesis (Figure 1, opposite). Although models and modelling can appear impenetrable to many people, at their heart, models are simply summaries of knowledge and data captured in very explicit and formalised ways. As such, they reflect the data and knowledge that exists in a particular area and if these are inaccurate or incomplete, the model will reflect this. The majority of such models employed for safety in DMT are ones that capture chemical quantitative structure activity relationships (QSAR)10. QSAR models are chemically diverse but in general, biologically simple usually relying on data generated from specific pharmacological screens and as such the development of a QSAR model rep – resents the final destination of the journey that started with a patient ADR.
Case example of translating patient safety to preclinical action: reducing cardiac liabilities
Sudden cardiac death and recurrent syncopal syndromes have represented a significant challenge to medical science for many decades and may result from abnormalities of cardiac structure or electrophysiological function. One such group of inherited abnormalities manifests distinctly on the cardiac electorcardiogram (ECG) with prolongation of the QT interval, hence the term ‘Long QT syndromes’. The particular risk associated with this prolonged QT is that of fatal cardiac arrhythmia, particularly Torsades de point (TdP) and Ventricular Fibrillation (VF). Initially described by Romano in the mid 1960s11, the molecular basis of these hereditary syndromes has been elucidated in the past 15-20 years, starting in earnest with identification of mutations in the Human-ethera- go-go related gene (hERG)12. This gene has been confirmed as encoding a potassium channel, which is essential for normal repolarisation of the cardiac myocyte. Although other ion channels can be affected with similar outcomes, hERG is by far the most common gene for clinically significant mutations and, most importantly from an ‘applicability’ perspective, has also been found to be the most commonly implicated target in a number of drugs associated with QT prolongation.
This molecular mechanistic convergence has allowed development of screening cascades to support the DMT cycle and to reduce the QT liability of drugs progressing into clinical testing13. Figure 1 summarises such a screening approach, which includes both in vitro hERG assays to test new compounds for potential hERG activity and in silico models developed from these assays, which are in turn used to influence the compound design phase.
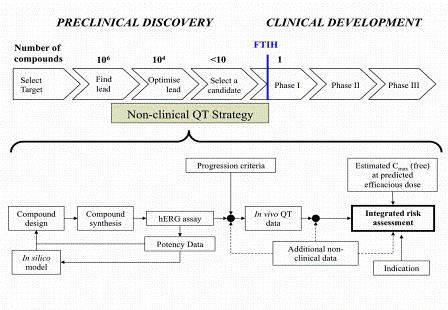
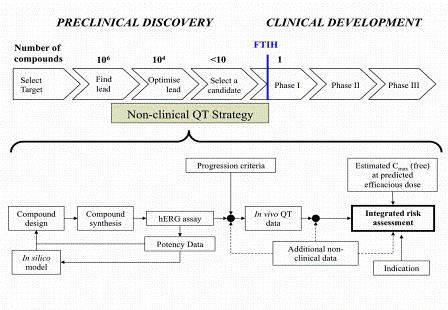
Figure 1: A generic drug discovery and development process in relation to a non-clinical QT strategy (Adapted from Pollard et al13 and reproduced here with kind permission of the authors).
The hERG story illustrates how effective screening processes can be implemented preclinically (albeit with significant cost and effort) when a common and well understood molecular pathway accounts for the majority of observed clinical adverse drug effects. Although, the implementation of hERG screening has undoubtedly influenced drug development, long QT and arrhythmias only account for a proportion of drug related cardiac safety concerns. What of a scenario where such molecular understanding is less complete or divergent with many potential mechanisms having the same patient outcome? Can screens be usefully developed in a cost effective and timely manner?
A current challenge relates to a heterogeneous group of clinical adverse effects encompassed here by the term ‘Cardiac dysfunction’ e.g. overt heart failure, signs and symptoms associated with heart failure and abnormal cardiac imaging studies. Undoubtedly, these represent a serious concern to both cardiologists and to patients and can result in significant increases in morbidity and mortality as well as a burden of monitoring. Mechanistically these effects might reasonably suggest adverse drug effects on cardiac myocyte contractility. We understand mycocyte biology well and could postulate more specific molecular pathways for such effects. One could conceptualise a scenario where assays could be used to evaluate parameters such as myocyte shortening or calcium flux14 during the DMT phase of drug development. However, one of the key factors preventing the replication of the hERG screening cascade is the definition of clinical safety endpoint (the ‘forward translation’ of the preclinical screen). QT prolongation is relatively well defined and measured in a reproducible fashion in humans and multiple animal species. Syndromes of ‘cardiac dysfunction’ lack such precise definition and as such, consistency / ubiquity of translatable monitoring tools. A key learning point here is that to improve our predictive risk mitigation strategy requires prioritisation of resources as there are many adverse effects currently defying prediction. The prioritisation process for developing screens to prevent or reduce harm to patients should include an assessment of the probability of success and a critical element often overlooked by those developing assays in the pre-clinical environment is the complexity of the clinical scenario.
Conclusions
Clinical adverse events always have complex aetiology involving patient characteristics, drug properties and often many other factors, some of which we may never be aware15. Adverse events are ‘detected’ either by patient reports to healthcare professionals or through evaluation of ‘routine’ (standard) monitoring tests e.g. blood liver enzyme levels. There is no strict definition of which tests should be included in clinical trials but typically these would include blood, urine and some ECG monitoring pre-dosing, during treatment and after discontinuation of study therapy. The premise is that of monitoring vital organ function in case something undesirable happens i.e. they serve as a safety net to cover the unknown. A consequence of trying to account for this ‘unknown’ risk may be the requirement for larger and longer trials, beyond that needed to demonstrate efficacy, in order to capture potentially rarer safety observations, driving up costs. When such clinical events are determined to be drug related, mitigation steps can be taken to protect subjects from harm, largely by using the product label to define how to administer and monitor the drug. This ‘reactive’ clinical risk management paradigm is changing: questions rightly arise as to how to prevent such problems in future or at least how better to predict their appearance before clinical testing even begins; clinical drug developers challenge their pre-clinical colleagues to predict and reduce risk. Moving to this predictive and preventative approach where we clinically monitor for the ‘predicted’ as opposed to the ‘unknown’ will help to reduce unnecessary, potentially intrusive and stressful patient monitoring and drive down the costs in delivering clinical trials. As can be seen for hERG screening, this change is already underway.
However, delivering greater predictive power to translate from the laboratory to the clinic requires mechanistic research in order to reduce potentially complex patient outcomes into practical screens and models that can be deployed during the DMT cycle of early drug discovery and development, and in turn, produce meaningful predictions that can influence trial design. This approach is built on an assumption that there are only a finite number of mechanisms for drugs to induce similar adverse clinical outcomes. However, this is not that same as saying that we can have a simple one-preclinical-in-vitro-screen-for-everypatient- ADR and although hERG may be an example of this, it is likely that this is the exception rather than rule. This is because ADRs in response to a specific drug are multifactoral in their nature and may be dependent on a ‘perfect storm’ of diverse factors (see for example Chalasani N and Björnsson15) and could, perhaps counter-intuitively, include ‘protective’ effects that might actually act to attenuate a safety response (see for example Shell et al16). Understanding the complex interplay of many factors means adopting a different approach to the analysis and integration of preclinical data that takes a much more quantitative account of how many factors contribute to a patient response. New in silico modelling approaches are starting to tackle this challenge and aim to put the data generated by preclinical screens back into the patient context, albeit it a virtual one17 and in doing so make more effective predictions of patient risk.
In this article, we have tried to give a flavour of the challenges of meeting the ideal of producing safe drugs prior to clinical testing as ultimately the best way of ensuring patient safety. We may fall short of this ideal, but that does not mean we should not drive towards it. However, meeting this ambition will require close collaboration between clinical and preclinical scientists on a daily basis because the ‘causes and effects’ in pharmaceutical R&D are separated not just by time and space but also by discipline and often organisation. We would therefore argue that in addition to imple – menting new technical solutions to the prediction and understanding of patient drug responses, we also need to consider how we organise ourselves in R&D in order to best facilitate translation from clinical to laboratory and back again.
References
- Suntharalingam G et al. (2006). Cytokine storm in a phase 1 trial of the anti-CD28 monoclonal antibody TGN1412. N Engl J Med 355, 1-11
- Kola I and Landis J (2004). Can the pharmaceutical industry reduce attrition rates? Nature Reviews Drug Discovery 3, 711-716
- Issa, AM et al. (2007). Drug Withdrawals in the United States: A systematic review of the evidence and analysis of trends. Current Drug Safety 2 177-185
- http://www.ich.org/
- Norris et al. (2005). Selectivity of SB203580, SB202190 and Other Commonly Used p38 Inhibitors: Profiling against a multi-enzyme panel. Letters in Drug Design & Discovery 2, 516-521
- Jaeschke H et al. (2012). Oxidant stress, mitochondria, and cell death mechanisms in drug-induced liver injury: lessons learned from acetaminophen hepatotoxicity. Drug Metab Rev. 44(1), 88-106
- Therapontos C, et al. (2009). Thalidomide induces limb defects by preventing angiogenic outgrowth during early limb formation. Proc Natl Acad Sci U S A. 106(21), 8573–8578.
- Ito T, et al. (2010). Identification of a primary target of Thalidomide teratogenicity. Science. 327, 1345-1350
- http://www.emea.europa.eu/docs/en_GB/ document_library/Press_release/2009/11/WC500014 774.pdf
- Przybylak KR and Cronin MT (2012) In silico models for drug-induced liver injury-current status. Expert Opin Drug Metab Toxicol, 8(2), 201-217
- Romano C (1965). Congenital cardiac arrhythmia. Lancet. 285(7386), 658-659
- Curran ME (1995). A molecular basis for cardiac arrhythmia: HERG mutations cause long QT syndrome. Cell. 80(5), 795-803
- Pollard CE et al. (2010). An introduction to QT internal prolongation and non-clinical approaches to assessing and reducing risk. Br J Pharmacol. 159(1), 12-21
- Abassi YA et al. (2012). Dynamic monitoring of beating periodicity of stem cell-derived cardiomycocytes as a predictive tool for preclinical safety assessment. Br J Pharmacol. 165, 1424-1441
- Chalasani N and Björnsson E (2010). Risk Factors for Idiosyncratic Drug-Induced Liver Injury. Gastroenternology 138, 2246-2259
- Shell SA et al. (2008). Activation of AMPK is necessary for killing cancer cells and sparing cardiac cells. Cell Cycle. 7(12), 1769-75
- Cook, D. (2010) Applying systems biology and computer simulations to predicting idiosyncratic DILI Feature Review. European Pharmaceutical Review. Issue 4
Figure 1 further information: The upper chevrons show a generic description of a small molecule drug discovery and development process divided into the Preclinical Discovery phases (Target Selection to Candidate Selection) and Clinical Development phases separated by First Time in Humans (FTIH) testing. The scale of the numbers of compounds being tested and considered in each phase is indicated. The lower flow diagram summarises a non-clinical strategy for the relevant Preclinical Discovery phases as indicated by the green box. The left hand side focuses on the influence of the DMT cycle and is dependent on the availability of a suitable hERG assay that can be deployed to test new compounds during lead optimisation. Data from this assay over many projects can be used to develop an in silico model(s) that can be deployed to influence chemical design prior to testing. The right hand side summarises the additional preclinical QT testing that can be used on candidates later in testing as part of a comprehensive preclinical assessment prior to FTIH. Readers are directed to Pollard et al13 for full details
About the authors
Dr David Cook has a background in biochemistry, immunology and molecular biology with a PhD from Imperial College, London and three years of post-doctoral experience. Dr Cook has worked in the pharmaceutical industry for AstraZeneca for more than 16 years in a wide variety of R&D roles. He initially worked in the respiratory and inflammation area and was responsible for identifying the mode-of-action for a new class of immuno – suppressive. He was one of the founders of AstraZeneca’s systems biology group and led the pathway analysis capability in this department for five years. Dr Cook moved into the safety functions six years ago to lead the development of computational biology approaches in support of improving the translational, prediction and understanding of the safety of new medicines.
Dr James Milligan joined AstraZeneca after training in hospital medicine and neuro-immunology research. His interests from clinical practice include cardiology, neurology and rheumatology and he has industry experience covering routine pharmacovigilance at all stages of drug lifecycle as well as successful NDA and MAA submissions. Dr Milligan currently leads a global group with more strategic focus on scientific enablement and improvement across the ‘safety’ continuum. Challenges in which the group is currently engaged include: integration of thinking, working and information exchange between clinical and pre-clinical safety groups, benefit-risk quantification, safety biomarker validation and qualification, quantitative risk prediction and modelling.
Issue
Related topics
Clinical Trials, Drug Development, Drug Discovery, Drug Safety, Preclinical Research