Seeing more from every sample: using AI image analysis to transform clinical decision-making
Posted: 10 December 2021 | Pahini Pandya (Panakeia Technologies) | No comments yet
Artificial intelligence (AI)-based image analysis tools have the potential to completely revolutionise cancer diagnostics, saving time and money by reducing the need for lab testing and expanding global access to cancer diagnostic services. Here, Pahini Pandya, CEO Panakeia Technologies, discusses such technologies and their potential benefits.
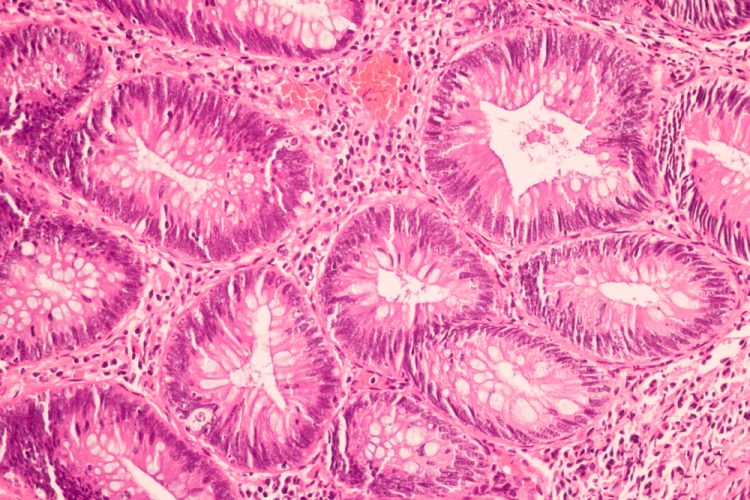
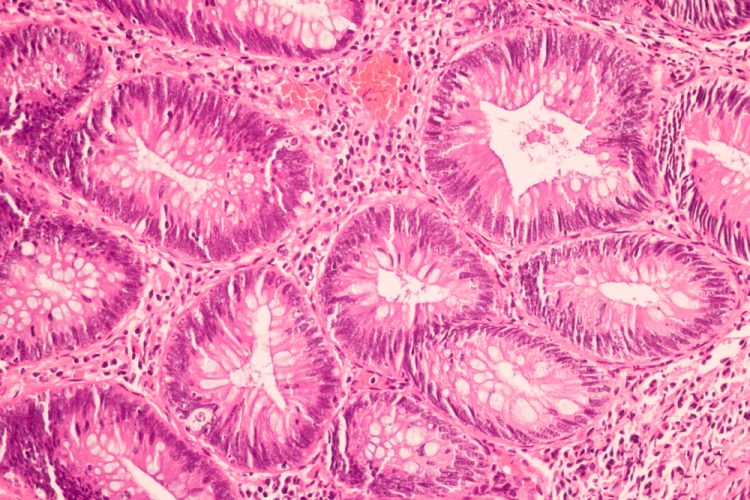
In diagnostic histology labs all over the world, breast tumour samples are cut into wafer thin slices, mounted on glass slides and stained with pink and purple haematoxylin & eosin (H&E) dyes. A pathologist will then examine the slide down a microscope or pore over a digital image to answer two vital questions: ‘What am I looking at?’ and ‘What do we need to do about it?’
The first question is relatively simple for a trained eye or digital image analysis software to answer. Breast cancer cells have specific characteristics that make them easy to pick out against their healthy counterparts, and there are several computer vision algorithms coming onto the market that can identify whether or not a sample is likely to be malignant.
The second is more challenging. While H&E staining can reveal the likely presence of rapidly proliferating rogue cancer cells, a straightforward visual check does not provide any information about the underlying properties of those cells and the best approach for treatment. Instead, once cancer is confirmed, the next step is to send a further sample for laboratory testing using immunohistochemistry (IHC), polymerase chain reaction (PCR) and/or next-generation sequencing (NGS). These tests cost several hundred pounds and can take several days or weeks to return an answer.
Due to the need for expert pathologists and lab testing, cancer diagnosis and treatment decisions can take a month or more. COVID-19 has also had a significant impact on cancer services, from referral and workup through to treatment – for example, experts suggest it could take more than a decade to clear the backlog resulting from the pandemic in England.1
Slow-moving lab testing adds an unacceptable and stressful delay when dealing with a fast-growing malignancy. Every month delayed in cancer treatment raises the risk of death by around 10 percent,2 highlighting the need for faster diagnostic workup that can reduce the time taken to get each patient started on the most appropriate therapy.
Predicting treatment from routine pathology
Recent advances in image analysis using artificial intelligence (AI) technologies such as convoluted neural networks are now able to glean much richer information from routine H&E pathology samples, revealing vital information about the underlying molecular state of the cancer cells and the likely best treatment options.
Modelling analysis shows that using this kind of high-sensitivity AI image analysis for determining microsatellite instability could save around $400 million in diagnostic and first-line treatment costs for metastatic colorectal cancer”
Numerous studies in recent years have shown that molecular alterations in cancers can cause changes in cell structure and the tissue microenvironment,3-5 suggesting that it should be possible to predict the underlying characteristics and biomarker status of cancers – and therefore the most suitable treatment – from their physical appearance in routine pathology images. However, these visual changes are usually too subtle or too varied to be detected by eye, requiring the development of deep learning computer vision algorithms capable of analysing digital pathology images and inferring the underlying molecular status.
Long thought to be an impossibly complex task, this idea is now a reality. Pioneering work by Jakob Kather and colleagues has demonstrated that it is possible to infer a wide range of genetic mutations, molecular tumour subtypes, gene expression signatures and standard biomarkers directly from routine H&E pathology images across a broad range of cancer types including lung, colorectal, breast and gastric cancer.6-8
Modelling analysis shows that using this kind of high-sensitivity AI image analysis for determining microsatellite instability could save around $400 million in diagnostic and first-line treatment costs for metastatic colorectal cancer by acting as a first line clinical decision-making tool.
This AI approach works for more than 80 percent of cases with 97 percent diagnostic accuracy and offers the potential for reaching an average time to treatment initiation of less than one day, with PCR or IHC testing only required for those samples that cannot be resolved by the algorithm.9
A global impact on cancer care
H&E staining has been used by pathologists for more than a century to reveal the appearance of cells and tissues under the microscope.10 AI image analysis has the potential to equip today’s pathologists with something akin to a visual superpower, allowing us to ‘see’ the underlying molecular state of those cells and transform the way that routine samples are used to inform clinical decision-making.
The falling costs and growing uptake of digital pathology, together with the use of online services for remote image analysis, will democratise access to precision medicine approaches”
Precision treatment of cancer currently relies on the accurate assessment of underlying biomarker status through laboratory tests, and clinical guidelines in many countries now recommend molecular testing for most patients with advanced solid cancers. However, lack of laboratory capacity and lengthy turnaround times are a bottleneck in cancer services, while the high costs of molecular testing act as a barrier to access in less wealthy countries.
Furthermore, while an initial tumour biopsy will usually be enough for standard H&E pathology analysis, there can be insufficient tissue remaining for further testing, requiring an additional biopsy (which may not be feasible) and further delays in treatment decision-making.
The falling costs and growing uptake of digital pathology, together with the use of online services for remote image analysis, will democratise access to precision medicine approaches in many low and middle-income countries.11 Additionally, though the costs of the latest targeted therapeutics may be out of reach, rapid and cheap assessment of biomarker status could expand the more effective use of widely-available generic treatments such as tamoxifen for breast cancer.
Applying AI algorithms to analyse routinely gathered H&E samples has the potential to revolutionise the speed at which cancer treatment decisions are made, significantly reducing the costs of laboratory testing without sacrificing accuracy.
About the author
Pahini Pandya PhD is the Chief Executive Officer and co-founder of Panakeia Technologies, a MedTech company and developer of an AI-enabled rapid diagnostics platform intended to reduce the cost, number of tests and time taken to establish a cancer diagnosis. After training as a cancer researcher at King’s College London and the University of Cambridge, Pahini studied at Stanford and Cambridge University business schools. She has been recognised as Global Shaper by the World Economic Forum and received multiple awards for entrepreneurship.
References
- Patel P, Thomas C. Building back cancer services in England [Internet]. IPPR. 2021 [cited October 2021]. Available from: https://www.ippr.org/research/publications/building-back-cancer-services
- Hanna TP, King WD, Thibodeau S, Jalink M, Paulin GA, Harvey-Jones E, et al. Mortality due to cancer treatment delay: systematic review and meta-analysis. BMJ. 2020 Nov 4;371:m4087.
- Shia J, Schultz N, Kuk D, Vakiani E, Middha S, Segal NH, et al. Morphological characterization of colorectal cancers in The Cancer Genome Atlas reveals distinct morphology-molecular associations: clinical and biological implications. Mod Pathol Off J U S Can Acad Pathol Inc. 2017 Apr;30(4):599–609.
- Greenson JK, Huang S-C, Herron C, Moreno V, Bonner JD, Tomsho LP, et al. Pathologic Predictors of Microsatellite Instability in Colorectal Cancer. Am J Surg Pathol. 2009 Jan;33(1):126–33.
- Orgaz JL, Crosas-Molist E, Sadok A, Perdrix-Rosell A, Maiques O, Rodriguez-Hernandez I, et al. Myosin II Reactivation and Cytoskeletal Remodeling as a Hallmark and a Vulnerability in Melanoma Therapy Resistance. Cancer Cell. 2020 Jan 13;37(1):85-103.e9.
- Kather JN, Heij LR, Grabsch HI, Loeffler C, Echle A, Muti HS, et al. Pan-cancer image-based detection of clinically actionable genetic alterations. Nat Cancer. 2020 Aug;1(8):789–99.
- Schrammen PL, Ghaffari Laleh N, Echle A, Truhn D, Schulz V, Brinker TJ, et al. Weakly supervised annotation-free cancer detection and prediction of genotype in routine histopathology. J Pathol [Internet]. [cited 2021 Oct 22];n/a(n/a). Available from: https://onlinelibrary.wiley.com/doi/abs/10.1002/path.5800
- Bera K, Schalper KA, Rimm DL, Velcheti V, Madabhushi A. Artificial intelligence in digital pathology — new tools for diagnosis and precision oncology. Nat Rev Clin Oncol. 2019 Nov;16(11):703–15.
- Kacew AJ, Strohbehn GW, Saulsberry L, Laiteerapong N, Cipriani NA, Kather JN, et al. Artificial Intelligence Can Cut Costs While Maintaining Accuracy in Colorectal Cancer Genotyping. Front Oncol. 2021;11:2066.
- Titford M. Progress in the Development of Microscopical Techniques for Diagnostic Pathology. J Histotechnol. 2009 Mar 1;32(1):9–19.
- Jahn SW, Plass M, Moinfar F. Digital Pathology: Advantages, Limitations and Emerging Perspectives. J Clin Med. 2020 Nov 18;9(11):3697.
Related topics
Analytical techniques, Artificial Intelligence, Imaging, Informatics, Technology