An eight-step Six Sigma toll-gate approach to PAT implementation
Posted: 21 September 2007 | | No comments yet
The FDA’s recent guidance regarding Process Analytical Technology (PAT) offers the pharmaceutical and biotech industries an unprecedented opportunity to leverage hard-won experience with scientific inquiry and innovation. However, the leap to PAT is significant for even the most rigorous development program. Many aspects of Six Sigma; including its use of statistical tools and its phase- or toll-gate approach to project management, can facilitate and accelerate a PAT initiative. Rather than advocating company-wide Six Sigma adoption as a prerequisite to effective PAT implementation, an eight-phase Design for Lean Six Sigma approach is recommended that can be used on a project-by-project basis.
The FDA’s recent guidance regarding Process Analytical Technology (PAT) offers the pharmaceutical and biotech industries an unprecedented opportunity to leverage hard-won experience with scientific inquiry and innovation. However, the leap to PAT is significant for even the most rigorous development program. Many aspects of Six Sigma; including its use of statistical tools and its phase- or toll-gate approach to project management, can facilitate and accelerate a PAT initiative. Rather than advocating company-wide Six Sigma adoption as a prerequisite to effective PAT implementation, an eight-phase Design for Lean Six Sigma approach is recommended that can be used on a project-by-project basis.
The FDA’s recent guidance regarding Process Analytical Technology (PAT) offers the pharmaceutical and biotech industries an unprecedented opportunity to leverage hard-won experience with scientific inquiry and innovation. However, the leap to PAT is significant for even the most rigorous development program. Many aspects of Six Sigma; including its use of statistical tools and its phase- or toll-gate approach to project management, can facilitate and accelerate a PAT initiative. Rather than advocating company-wide Six Sigma adoption as a prerequisite to effective PAT implementation, an eight-phase Design for Lean Six Sigma approach is recommended that can be used on a project-by-project basis.
The shift from product control to process control
Prior to 2002, regulatory oversight focused primarily upon adherence to pre-defined procedures, record keeping and an audit trail, as a means for ensuring product safety and efficacy. Due to the emphasis on oversight control, most firms would ‘lock-down’ their processes and control methods once process validation was complete. Product quality was achieved through off-line inspection, rather than through identifying, understanding, controlling, and optimising critical process parameters. The FDA reinforced this mindset by requiring regulatory pre-approval before any changes could be made to the process. The FDA Modernisation Act of 1997 initiated a change in policy and thinking that culminated in the release in 2002 of the guidance document Pharmaceutical cGMPs for the 21st Century – A Risk Based Approach. To streamline the regulatory approval process and enhance patient safety, this document proposed a shift to a science-based compliance model, integrating the disciplines of quality, safety, and risk management.
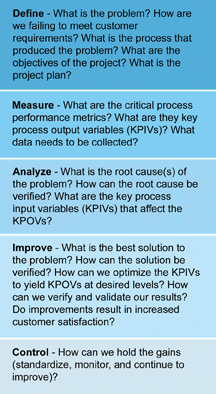
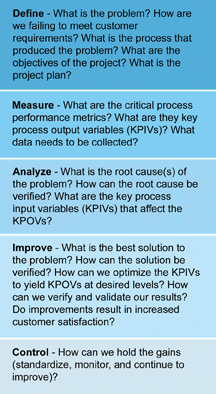
Figure 1: The Six Sigma DMAIC model.
Since 2002, the FDA has released guidance documents on risk-based inspections, Part 11 electronic records and signatures, quality systems approach to pharmaceutical cGMPs, and Process Analytical Technology (PAT). The FDA was not the only regulatory body to recognise this need and solicited input from its counterparts in Canada, Europe, and Japan, and from industry and academics worldwide. Several key guidance documents from the International Conference on Harmonisation (ICH), ICH Q8 (Pharmaceutical Development), ICH Q9 (Quality Risk Management), and the forthcoming ICH Q10 (Quality Management) have become the de facto standard for transforming organisations that aspire to the highest degree of scientific rigor in the product development process. These documents comprise the basis for shifting manufacturing and regulatory philosophy from inspection and oversight to managed risk: a scientific approach capable of providing a higher level of product quality assurance.
Six Sigma and PAT
In September 2004, the FDA issued its final guidance, “PAT: A Framework for Innovative Pharmaceutical Development, Manufacturing, and Quality Assurance.” The fact that the guidance extends beyond a pure hardware solution underscores the Agency’s desire to shift product quality assessment away from a product-centric approach, based on inspection and final testing, to one that is more process-centric and built upon understanding the variables that affect overall product quality. Process Analytical Technology (PAT) represents the culmination of a true process-centric quality system. Unfortunately, the industry has had difficulty embracing the total vision for PAT, partly because of its radical departure from historical methods of process and product development, and partly because of the lack of a definitive implementation model.
A criticism leveled at some PAT implementations is that efforts have focused on the application of on-line analytical technology (as a replacement for off-line laboratory testing), rather than on understanding control and reduction of variation1. In other words, the focus has been on the measurement, rather than the improvement of product quality. Statistical tools for characterisation and optimisation of manufacturing processes have been quietly in use in industry for more than 50 years, although often confined to use by corporate statisticians. A renaissance in the more widespread use of industrial statistics by non-statisticians came with the advent of the improvement methodology of Lean Six Sigma in the mid-1980s.2 A search of the PAT literature reveals an emphasis on the use of statistical tools, particularly multivariate methods.3,4,5 However, the Lean Six Sigma approach to projects provides many advantages over isolated use of statistical tools. Among those advantages are:
- Selection of limited scope improvement projects, according to verified bottom line cost savings and increased customer satisfaction, achievable in two to six months.
- Use of cross-functional teams led by a Six Sigma Black Belt (not a degreed statistician although trained in the use of statistical tools).
- Sponsorship of projects by a corporate executive champion, whose role is to remove political, financial, and other barriers that stand in the way of the team’s success.
- Structuring the project in phases or stages, with each phase having defined statistical and lean tools, and objective criteria and metrics for the success of each phase. The most common approach is known by the acronym DMAIC; which stands for Define, Measure, Analyse, Improve, Control.
- Toll-gate review meeting at the end of each phase. During this meeting, the team presents progress to date to members of senior management. If the team pays the “toll” by meeting the criteria and metrics for the phase, management raises the “gate” and allows the team to move to the next phase.6
The improvement methodology of Six Sigma provides an effective framework for the process characterisation and optimisation required by PAT that is superior to the use of statistical tools in isolation. A company-wide conversion to Six Sigma and its requirements for significant cultural change is not required. A less resource-intensive alternative is to use a Six Sigma project structure and associated statistical tools to characterise, control, and reduce process variation to achieve more consistent product quality. It is the authors’ opinion that a phased Six Sigma toll-gate approach to product and process improvement significantly enhances and accelerates PAT implementation.
Based upon an actual PAT deployment initiative facilitated by Pharmatech Associates, the methodology employed and challenges encountered in the course of a PAT implementation project for a business unit of a major pharmaceutical company will be presented.
Business problem
The business unit had identified a number of improvement opportunities in its manufacturing process flow at one of its solid dosage manufacturing plants. The product was a controlled-release tablet which utilised a high molecular weight polymer to control the diffusion of the drug. The release profiles of the drug had been inconsistent since its market introduction two years previously resulting in rejected lots and a higher than desirable incidence of stage two dissolution testing. The inconsistent performance presented a potential regulatory risk to the product unless the variable dissolution performance could be addressed. In addition, the product demand was growing and the yield impact contributed to an erosion of plant capacity causing missed shipments and lost revenue to the business unit. Based upon projections there would be insufficient capacity to meet next year’s demand.
A series of characterisation studies was initiated using orthogonal experimental designs, evaluating API, granulation and compression parameters to identify the key parameters which affect dissolution performance. The investigation identified the root cause as periodic over-mixing of the lubricant during the final blending step, resulting in more hydrophobic surface properties. The inconsistent mixing performance was ultimately attributed to varying raw material properties, in particular particle size distribution, due to alternate lubricant suppliers. This source of variation was addressed through the establishment of a particle size distribution specification. In addition, only suppliers that demonstrated a process capability greater than 1.0 against the specification were qualified for the process.
Faced with the looming regulatory risk and capacity shortfall, management decided to initiate a PAT program to determine if the inconsistent product performance issue could be addressed through the use of in-line analytical measurements and closed loop control. The decision was made to deploy a PAT team to implement improvements with the objective of eliminating or significantly reducing process instability.
Plant process flow
The process flow for manufacturing is shown in Figure 2. The major unit operations are compounding (API addition in solution), granulation, milling, blending, and tableting. Given the results of the root cause analysis exercise, the project focused upon the final blending step for the PAT project.
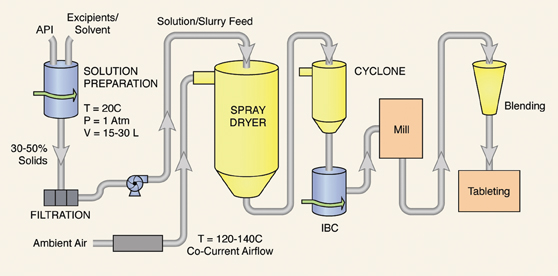
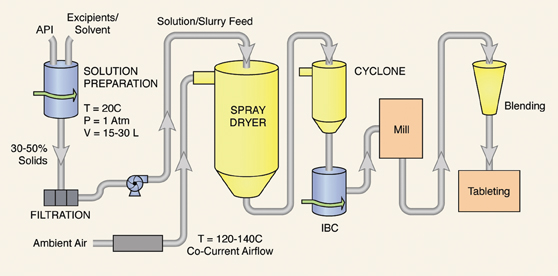
Figure 2: Controlled release tablet process flow
PAT team
To deploy the project, the business unit established a PAT project team that consisted of experts from across the business unit, an outside pharmaceutical consulting firm, and an automation supplier. Although they were not a formal Lean Six Sigma organisation, the pharmaceutical company had experience using many of the Lean Six Sigma statistical tools. The PAT team decided to apply the Six Sigma structure to the project from the outset because of the perceived advantage of a toll-gate approach with its built-in checks and balances. The Six Sigma approach allowed the team to clearly articulate success metrics for the individual stages of the project, as well as for the project as a whole, and align the project with current business objectives and strategy. The toll-gate approach, with its use of incremental success metrics, was instrumental in garnering senior management support from across the organisation throughout the project. The team’s first task was to define the deliverables and ensure consistency with current business and regulatory objectives. As described earlier, the plant was suffering from a capacity shortfall. The team met and summarised the situation as follows:
- At the current manufacturing rate, the inconsistent tablet dissolution profile was costing the company $20 million on an annual basis, not including the cost of poor quality associated with handling rejected material.
- Rejected lots needed to be reduced to no more than 5% in order to recover the necessary manufacturing capacity for the coming year.
- Estimated cost to the business unit due to dissolution failures or Stage 2 testing requirements was approximately 8% of the standing Work-In-Process (WIP) cost.
Based upon this assessment, the team determined it was appropriate to proceed with the PAT project. It is important to note that the objective in this case, from a business perspective, was not to replace the quality overhead associated with the tablet release, but rather to prevent the loss of product due to poor dissolution. This greatly simplified the initial regulatory strategy for the project, while leaving the door open for a future filing to replace product release testing with an in-process control strategy.
Six Sigma or design for Six Sigma?
The team explored several models to evaluate the application of Six Sigma to the project. The classic Six Sigma DMAIC model provides a good framework for objective scientific inquiry and is typically used to improve existing processes (and products). However, the team decided that Design For Lean Six Sigma (DFLSS), with its focus on the development of new products and processes, would be a more appropriate approach for the PAT project. Subsequently, the team evaluated several of the current DFLSS Models as alternatives to the classic DMAIC model. DFLSS models provide a structured, phased approach to the design of a product, process, or service with Six Sigma Quality (target of 3.4 defects per million opportunities) and efficiency as key design criteria. Risk management is easily incorporated in the approach, as Failure Modes and Effects Analysis is a standard DFLSS tool. Integration of DFLSS with PAT answers the criticism of some current PAT implementations that focus too much on on-line analytical instrumentation rather than on the sources of process and product variation. This DFLSS toll-gate approach to PAT provides the additional advantage of a set of measurable success criteria for completion of key milestones within each phase of the process so the “gate” can be closed. Comparison of progress with such criteria provides objective evidence of incremental team success (that can be celebrated and communicated to the rest of the organisation) and helps prevent team self-delusion. The DFLSS models7 under consideration were Define, Measure, Analyse, Design, Verify (DMADV), Identify, Design, Optimise, Verify (IDOV), and Define, Characterise, Optimise, Verify (DCOV) – Figure 3.
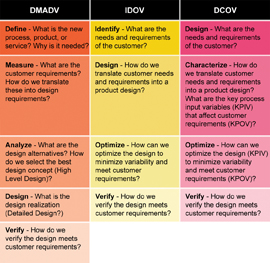
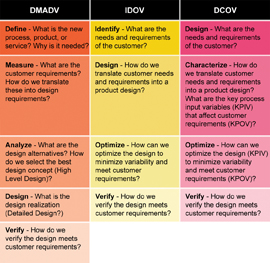
Figure 3: Lean DFSS models
Six Sigma PAT
In a review of the literature and with the recommendation of the pharmaceutical consulting firm, the team decided to use the DCOV DFLSS model with its focus on process characterisation and optimisation. The team expanded the DCOV roadmap into the following eight phases: Identify, Characterise, Define, Optimise, Measure, Automate, Verify, and Validate. The modified DCOV project management approach allows the business to make the best possible decisions with the available data and resources. The purpose behind each step of the eight-phase process is as follows:
- Identify: clearly identify key elements of the project, including: regulatory strategy, regulatory commitment to Key Process Output Variables (KPOVs).
- Characterise: what are the Key Process Input Variables (KPIVs) that have been characterised as they relate to the KPOVs?
- Define: what is the defined design space for the process?
- Optimise: what is the control space that defines the allowable KPIV levels in order to maintain the process within the design space?
- Measure: what analytical solutions are possible surrogates for the existing offline measurement systems?
- Automate: what control solutions can be applied to leverage?
- Verify: prepare a proof-of-concept, process model.
- Validate: complete the IQ, OQ, PQ, method validation and comparability study.
Within each of the phases, there are a set of deliverables that must be completed to ensure all project requirements are met. Each will be discussed as follows:
Identify
In the identify phase, the PAT team is tasked with determining the design criteria for moving forward with the PAT strategy. The key process parameters, such as API physical characteristics, granulation process/control, and compression force/tablet hardness had previously been determined not to be the source of dissolution variation, leading the team to focus on the blending step. On the process side, the team developed a flow chart to identify the Process Input Variables and the Process Output Variables to design into the PAT solution. The input variables for the blending process identified were as follows:
- Granulation Particle Size Distribution
- Mixing Time
- Intensifier Arm
- Lubricant PSD
- Lubricant concentration
The process utilised a 60 cu.ft. mixer. The Chemistry, Manufacturing, and Controls (CMC) commitment during the original drug filing was to mix for five to 15 minutes, at a mixing speed of 10 rpm with the intensifier arm on. The KPOVs filed in the NDA for this step were content uniformity and tablet dissolution at two, four, and eight hours. The specification was 10 to 20%, 21 to 60%, and 61 to 100% respectively for these time points. The team determined it would use the tablet dissolution, API content uniformity, and concentration of lubricant as benchmarks for evaluating the content uniformity of the lubricant and the mixing effectiveness during that stage of the process. The regulatory strategy initially focused on establishing a control range within the NDA commitment. Since PAT focuses on a feedback control architecture, the intent was to establish a scientifically rigorous comparability data set using the optimised control range, then steer the target metrics for PAT automation to the same endpoints.
At this and subsequent phases, success metrics were established. Progress and metrics were presented to management at a toll-gate review meeting with management’s charter to give the team approval to move to the next phase or to take additional action to resolve any open issues. For any open issues, the team would submit a formal corrective action to get management’s approval to move to the next phase. This process continued through the subsequent seven phases.
Characterise
A retrospective review of the process development data indicated that there was no evaluation of the impact of granulation PSD or lubricant PSD. Lubricant concentration was evaluated, as was mixing time. Neither evaluation used an orthogonal experimental design; hence, the data could not be regressed. The development data evaluated 1% and 2% lubricant concentrations. Based upon this development work, the significant KPIV identified was mixing time with the KPOVs being tablet appearance and dissolution. Tablet appearance was representative of the tablet compression process. A final concentration of 1.5% was chosen.
Given the lack of information from the original development work, a characterisation study was initiated to evaluate the impact of lubricant concentration, mixing time, and whether the intensifier arm was used. The ICH Q8 guidance describes this evaluation as defining the knowledge space for the process. An orthogonal experimental design, using a blocked design for the intensifier bar, was performed. Mean granulation size and PSD and lubricant PSD data were measured and kept constant for the study. The results indicated that lubricant concentration and mixing time KPIVs were both significant at all three dissolution points. The intensifier bar did not have an effect. Key KPOVs measured were drug dissolution, drug content uniformity, and tablet appearance.
Define
ICH Q8 discusses identifying the optimum design space for the process. The design space is a subset of the overall knowledge space for the manufacturing process. A graphical representation of the relationship between the knowledge, design, and control space is shown in Figure 5. In evaluating the influence of key process inputs, the team focused upon a tiered approach to reducing PAT risk. It was agreed the minimum acceptance criteria was to achieve drug content uniformity. Once the control space was established, the behavior of the lubricant would be evaluated. The objective was to find a control space in which drug content and lubricant uniformity could be assured.
The knowledge space defines the boundaries within which the process inputs or KPIVs can be varied. However, within the knowledge space, some parameters at their limits may not produce acceptable product and some parameters may have no impact on the critical KPOVs (in this case study – content uniformity, dissolution, and lubricant content). The design space then represents the widest range of each of the KPIVs within which acceptable product meeting all of the KPOV specifications can be manufactured under ideal and controlled conditions. The control space represents a further tightening of the design space of the KPIVs in which acceptable product is assured of meeting specifications, allowing for process drift and measurement and sampling uncertainty.
The PAT team initiated a follow-on study, designed to characterise the design space. The lubricant concentration was fixed at 1.5%, and the intensifier bar was not used. In order to evaluate the impact of granulation PSD, the percentage fines was evaluated. Two different suppliers of lubricant also were evaluated. All satisfied the revised specification for the lubricant. The DOE evaluated the following possible input parameters:
- Mixing time: 7 to 12 minutes
- Granulation Percent Fines: 10 to 40%
- Lubricant Lots: 1 to 2
The study revealed that mixing time and granulation PSD were significant KPIVs for drug dissolution at the two, four and eight hour time points. The lubricant lots were not significant contributors to either drug content uniformity or lubricant content uniformity. All lots passed Level 1 dissolution testing.
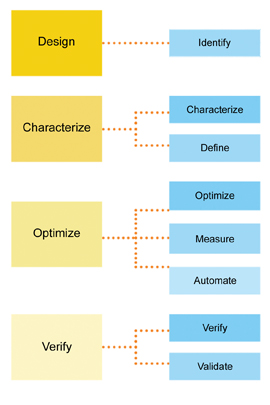
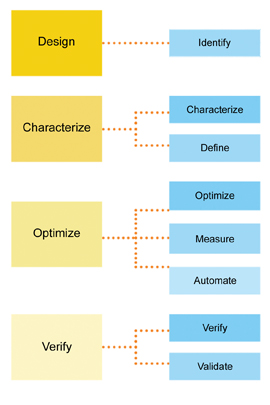
Figure 4: Design for Lean Six Sigma model applied to PAT
Optimise
The next step in identifying the final processing space is to identify the control space. The control space represents a range of critical parameters within which the process will yield an assured output within the KPOV specifications allowing for sources of statistical uncertainty. It also represents the basis for the control architecture to be adapted for the PAT solution. Powder mixing theory states that the components that impact blend uniformity are:
- Granulation/blend physical characteristics, including particle size distribution
- Shape and moisture content
- Powder bulk density
- Van Der Waals forces.
Of these, granulation particle size is the most significant factor. Given that granulation PSD was identified as a significant contributor, a Six Sigma exercise was initiated to understand the variability in the final granulation. Milling steps upstream of the blend step were evaluated and modifications to the milling set-up to control the feed rate of granulation were made. A screening study was repeated to determine if the new granulation PSD was still a significant contributor to blend uniformity and it did not come up as significant at the 95% confidence interval. Based upon this, the control strategy established a baseline of lubricant distribution to serve as the comparability criteria for the PAT solution downstream. The team did not focus on the drug content uniformity since the knowledge and design space studies had moved the process away form the edge of failure, while characterising the variability around the KPIVs that would affect drug content uniformity.
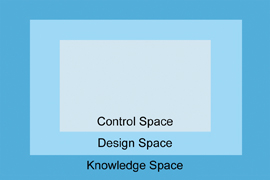
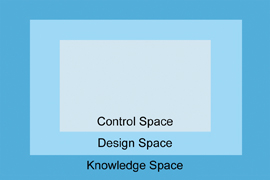
Figure 5: Relationship of knowledge, design, and control spaces
Measure
The challenge in developing an in-line metric for ensuring proper mixing of the lubricant was the lack of an off-line test currently being performed for lubricant content in tablets. A baseline examination of tablets manufactured during the control phase was performed using Mass Spectroscopy (MS) in order to understand the variability around the control space. Tablets which exhibited poor dissolution also were evaluated from the original failed lots. The results of the MS data are shown in Table A. The most striking observation is that the tablets which exhibited poor dissolution had significantly higher levels of lubricant.
Automate
The team had sufficient understanding of the behavior of the current process, its KPIVs and KPOVs, to move to identifying an automation solution. The PAT team included an external automation firm with a strong understanding of process, Design for Six Sigma, and GAMP 48 to complement their experience in custom automation. This is a significant consideration given the intimate relationship between the technical solution and quality and regulatory considerations for the project. Having a solutions provider that possesses the systems to integrate the requirements of Quality by Design (QbD) is a major advantage in developing the scientific argument that the in-line solution is an equivalent or superior surrogate to the off-line analytical solution and in generating the necessary documentation trail to support subsequent validation.
The team approached the automation solution in phases. The first phase was designed to ensure there was a solid understanding of the existing process performance using off-line analytical tools. Tablet performance was currently measured using HPLC for content uniformity and potency assessment. Tablet dissolution coupled with UV spectroscopy determined the tablet’s release profile. Currently no off-line assessment was performed on the lubricant. This had been found to be a key factor in achieving the desired tablet dissolution profile. The second phase was to establish a correlation with the new surrogate analytical method. The last phase was to demonstrate that the hardware solution and control algorithm resulted in tablets that satisfied the product’s release criteria. The team focused on establishing a correlation between an off-line and in-line method for lubricant concentration. Tablets were analysed using MS and FT-NIR to establish the correlation. The results are shown in Table B. Based on the results of the correlation study, the team demonstrated that the in-line solution was viable and they could proceed with developing an in-line solution.
Verify
The verify step is used to establish a basic proof of concept that the principles of the solution are viable. In the previous phase, the team attempted to establish a correlation between the offline and in-line measurement systems. Adherence to the Six Sigma methodology had narrowed the control space to keep the process sufficiently far from the edge of failure. The first focus was establishing a measurement for the lubricant in the blending step, which could be used to dictate the blending time. It is important to note we are not concerned with lubricant weighing errors; rather with the distribution of lubricant through-out the granulation. Lubricant integration with the granulation particle has been shown to impact the dissolution of some controlled-release tablets as mixing time increased.
Since confidence was high that material mixed for seven to 12 minutes resulted in tablets with acceptable dissolution, then the correlation with lubricant concentration could be one trigger used to prevent overmixing. A non-destructive test was required to demonstrate comparability. A sample size of 100 tablets was selected. With the selection of FT-NIR as the measurement tool, a blender was modified with a self-contained analytical probe and analyser and equipped with a wireless transmission system to deploy as a proof of concept system. The final control space screening study was repeated. Measurements were taken from the in-line sensor and tablets were tested using MS for lubricant content. In addition, tablets were tested for content uniformity, potency, and dissolution at the three, four, and eight hour time points.
One of the key metrics for process performance is a measure of process capability, Cpk. This metric for a process with a normal (or close to normal) distribution and a two-sided specification is described by the equation:
Where, USL/LSL = Upper/Lower Spec Limit
X = Mean
S = Standard Deviation (sigma)
Cpk assumes that the process is in statistical control. In simple terms, Cpk compares the spread of your process to the spread of your specification and how close the mean of that process is to the specification limits. A high number (>1.33) indicates that the probability of getting an out-of-spec product is very small. Cpk was calculated after determining that the process was in control through a control chart. All dissolution time points had a process capability greater than 1.33 (4 sigma process). The process capability chart for the eight hour time point is shown in Figure 6.
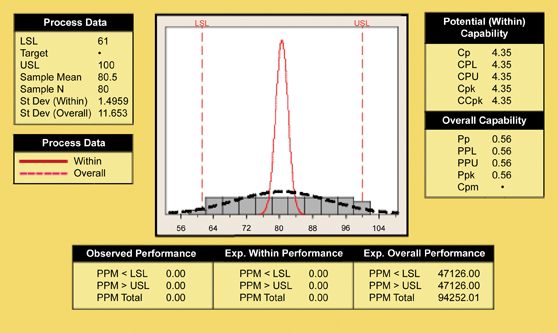
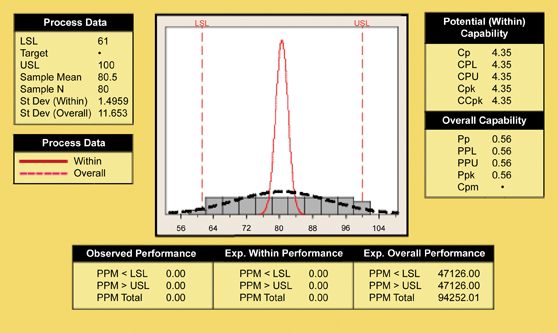
Figure 6: Process capability curve for eight hour tablet dissolution time point.
The results illustrate that the FT-NIR system was capable of controlling the process and delivering compliant product. Based upon these studies, a change control notice was initiated and the production equipment was modified.
Validate
The final step in the process was to validate the equipment and process. The Six Sigma process dictated the elements to be completed as follows:
- Generate the Final Development Report
- Baseline the Equipment
- Modify Operational SOP
- Modify Maintenance SOP
- Modify Calibration Program
- Software Validation-Part 11 Compliance
- IQ/OQ/PQ
- MS and FT-NIR Method Validation
- Regulatory Update
Conclusion
The statistical tools and toll-gate process of Six Sigma provides a best practice process for characterising, controlling, and reducing process variation that is necessary to successfully deploy PAT. The partnership of Six Sigma and PAT was intended to characterise and implement a control and measurement solution, which would minimise the likelihood of a controlled-release tablet failing dissolution. The team used a modified DCOV model subdivided into eight phases designed to ensure that the basic requirements of the ICH Q8 requirement for QbD were satisfied. This framework ensured all aspects of the project were addressed in an efficient and methodical manner with the scientific rigor necessary to implement an in-line control architecture integrated throughout the process.
About Pharmatech Associates, Inc
Founded in 1995, Pharmatech Associates, Inc. provides a full range of consulting services to the pharmaceutical, biotech, and medical device industries. Pharmatech’s end-to-end service capability is achieved through its four divisions: Validation Services, Consulting Services, the Regulatory Compliance Group, and Project Controls. Together this expertise spans the entire product development lifecycle, from pre-discovery to Phase 4 Pharmacovigilence and operational excellence. Pharmatech offers technical solutions fully informed by the business mandates of production processes. Our operational expertise enables life-sciences client organisations to meet the exacting requirements of U.S., Canadian, and European regulatory agencies.
Pharmatech differs from other service providers to the life sciences via:
- Methodology: the leader in applying empirical data-driven analytics
- Industry expertise: Deep hands-on technical and management experience in biopharma and device manufacturing operations
- Operational Support Capability: Trained staff capable of supporting organizations on the factory floor
- Regulatory Compliance Understanding: Former FDA professionals, architects of Agency policies and procedures for the last quarter century
Our clients include:
- Access Closure
- Alza
- Aradigm
- Avigen
- Baxter Healthcare
- Biomarin
- Boehringer Mannheim
- Boericke & Tafel
- Centaur Pharma
- DepoMed
- Elan Pharmaceuticals
- Fusion Medical Technologies
- Genitope
- Gilead Sciences
- Guidant Corporation Impax Laboatories
- Layton Biosciences
- Nektar Therapeutics
- Neutrogena
- Onyx
- Orquest
- Powderjet Technologies
- Rigel Pharmaceuticals
- Roche Molecular Systems
- SanBio
- Shaklee Corporation
- SuperGen
- UCSF
- Watson Laboratories
- Xoma
- Yamanouchi Pharma America
References
- Maes, I. and B. Van Liedekerke, “The Need for a Broader Perspective if Process Analytical Technology Implementation is to be Successful in the Pharmaceutical Sector,” Journal of Pharmaceutical Innovation, Volume1, Number 1, September/October 2006, pp. 19-21.
- Kourti, T., PhD, “Process Analytical Technology and Multivariate Statistical Process Control: Wellness Index of Product and Process – Part 1,” PAT Journal, Volume 1, Issue 1, September/October 2004, pp. 13-19.
- Kourti, T., PhD, “Process Analytical Technology and Multivariate Statistical Process Control: Wellness Index of Product and Process – Part 2,” PAT Journal, Volume 2, Issue 1, January/February 2005, pp. 24-29.
- Kourti, T., PhD, “Process Analytical Technology and Multivariate Statistical Process Control: Wellness Index of Product and Process – Part 3,” PAT Journal, Volume 3, Issue 3, May/June 2006, pp. 18-24.
- Harry, Mikel and R. Schroeder, Six Sigma: The Breakthrough Management Strategy Revolutionizing the World’s Top Corporation, Doubleday, 2000.
- Pyzdek, T., The Six Sigma Handbook: A Complete Guide for Green Belts, Black Belts and Managers at All Levels, Revised and Expanded, McGraw-Hill, 2003.
- Brue, G., Design for Six Sigma, McGraw-Hill, 2003
- GAMP 4: The Good Automated Manufacturing Practice Guide for the Validation of Automated Systems, International Society for Pharmaceutical Engineering (ISPE), Version 4, December 2001.
Reprinted from Pharmaceutical Engineering. The official magazine of ISPE
March/April 2007. Vol. 27, No. 2
Bikash Chatterjee
President of Pharmatech Associates, Inc
Bikash Chatterjee is President of Pharmatech Associates, Inc. He has been involved in the bio-pharmaceutical, pharmaceutical, medical device, and diagnostics industry for more than 20 years. Most recently, he served as the Vice President, Pharmaceutical Operations for Aradigm Corporation where he was responsible for establishing their process development, engineering, validation, facilities, and manufacturing capabilities. Prior to joining Aradigm, Chatterjee was the plant manager responsible for manufacturing Boehringer- Mannheim’s disposable coagulation testing system. Chatterjee has designed, built, and qualified multiple facilities throughout his career, ranging from centralised warehousing to large capacity BSL-2 manufacturing facilities. His expertise includes site selection, project management, and design and validation of facilities for both US and European regulatory requirements, and he has been involved in the design and validation of biopharmaceutical facilities in the US, Europe, and Asia. From 1988 to 1992, he held a number of senior manufacturing positions at various pharmaceutical companies, including Syntex Corporation. Chatterjee is a certified ISO 9000 Lead Assessor, a Six Sigma Master Black Belt, and has more than 15 years of experience in the implementation of Lean Manufacturing programs in the life sciences industry. He holds a BA in biochemistry and a BS in chemical engineering from the University of California at San Diego.
Dr. Jeremy D. Green
Senior Consultant for Pharmatech Associates, Inc.
Dr. Jeremy D. Green is a Senior Consultant for Pharmatech Associates. He is a Certified Lean Six Sigma Master Black Belt, American Society for Quality Certified Six Sigma Black Belt, and Certified Quality Engineer with 20 years of experience in quality and manufacturing management. His background includes work in the pharmaceutical process automation, food, semi-conductor, and electronics industries. Dr. Green received his PhD from Indiana State University and his BA from the University of Illinois.