The application of skip testing to drug substance manufacture
Posted: 29 February 2016 | | No comments yet
Skip testing is a process employed to reduce the analytical drugs testing burden and lends itself to processes with high frequency batch production. Rather than test all batches within a given interval, pre-selected batches are assessed and the other batches ‘skipped’. This reduction is justified as it is shown that there is a low risk of any batches failing specification. In this article, a process is described (supported by an example) that could be followed to justify the use of skip testing. The process involves identifying attributes that are candidates for skip testing, performing a statistical evaluation to confirm there is a low risk of batch failure if skip testing is instigated and making ongoing assessments to confirm the process remains highly capable and the attribute(s) is predicted to be well within specification.
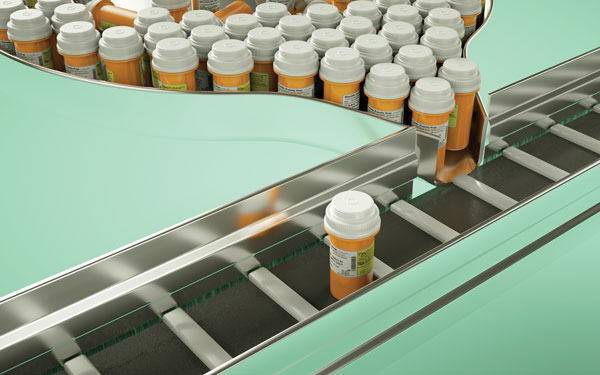
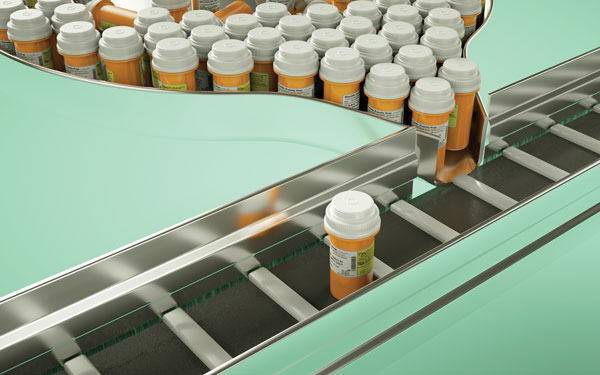
Introduction Skip (or periodic) testing is defined by The International Council for Harmonisation of Technical Requirements for Pharmaceuticals for Human Use (ICH)1,2 as conducting ‘‘specified tests at release on pre-selected batches and/or at predetermined intervals, rather than on a batch-to-batch basis, with the understanding that those batches not tested should still meet all the acceptance criteria established for that product’’. This represents a less than full schedule of testing and therefore for registered tests this must be justified, presented to, and approved by regulatory authorities before implementation. Following the inclusion of skip testing in ICH guidance1,2 there has been much discussion in the literature relating to its use3,4,5. Skip testing must only be implemented for low risk scenarios. This could be based upon a strong scientific rationale detailing why the skip-test is inherently unlikely to fail and/or using process capability data to show that the process is under control. Ongoing verification should also be applied to confirm the process is still capable of meeting specification. In addition, the following should be documented prior to implementation:
- Alternative measures which are in place to control the quality attribute if it is no longer to be confirmed through testing every batch;
- Scientific rationale which shows that the quality attribute has a low impact on safety and/or efficacy;
- Scientific rationale (supported by risk assessment) which demonstrates that the attribute is not critical to quality (i.e., it is not a Critical Quality Attribute7);
- Justification of skip testing intervals and batch selection (where selection should be made randomly within each interval);
- Process to be followed if a batch selected for testing fails to meet specification.
Examples of the first point – documenting other measures that are in place to control the quality attribute – include the use of an orthogonal (independent) analytical technique, or having a strategy in place to control the attribute6, e.g., through the use of a Design Space7 or via an in-process control such as a process analytical technology.
In addition to the above, the regulatory position on skip-testing for the particular test of interest needs to be understood. Skip-testing is typically considered for the analysis of low risk attributes in high frequency batch production processes. The skip-testing approach proposed in this article employs a high frequency batch production process, using as an example a drug substance process where over 3,000 batches of product a year are manufactured.
When implementing a skip-test strategy, consideration should be given to the frequency of testing/how many batches are not tested between tests (the frequency of the ‘skipping’). Historically the ‘square root (n) + 1’ has often been applied8,9. It should be noted, however, that this rule was developed for ‘skip-lot’ testing where it is lots within a batch, rather than individual batches, which are skipped. Lots within a batch are likely to be more similar than different batches, and hence there is a lower risk of making an error when skip-testing in this setting.
Given that batch-to-batch variability is higher than within-batch variability, there is always a risk that an individual batch may fail specification. To guard against this, the statistical assessment described as follows can be considered to be very conservative. However, by only allowing skip-testing to be applied when the risk of failure is considerably smaller than the level deemed to be reasonable (regardless of the choice of statistical assessment), there is no need to precisely quantify the risk.
Skip-testing strategy
The proposed strategy for implementing skip-testing implementation can be broken down into a four-stage procedure, as expanded on in each of the sections as follows (see also the flow diagram of the process in Figure 1; page 00).
Stage 1 – Selection of attributes via risk assessment
To begin with, the risks associated with the control of the critical quality attribute(s) and attributes are considered. Only attributes deemed low risk will be considered for skip-testing. These considerations include:
- Risk assessment: Impact of not testing a batch that fails specification (patient outcome, quality, yield, batch recall strategy, etc.)
- Related tests: the ability of an alternative test (that will be performed) to predict the ‘skipped’ test
- Robust control strategy: a strategy is in place to control the attribute, implying that an out-of-control process is not expected
- Historical knowledge: previous knowledge suggests the attribute routinely passes specification.
Other factors to consider in the initial risk assessment include whether batches can be retained until the outcome of the next batch being tested is known; and the ability to identify a change in the manufacturing process that could increase the risk of failure and the consequence of product recall (reputational damage and interrupted supply to patients).
Example – a high frequency batch production process (Stage 1)
In our example of over 3,000 batches being manufactured a year, comprehensive analytical trending information will be accumulated. It can be argued that with such a large number of batches being manufactured (a much smaller number of batches is typical for most drug substance manufacturing processes) some of the analytical testing associated with low-risk attributes can potentially be reduced. This could significantly reduce the cost of full release testing, as some attribute tests (i.e., analytical methods) require high labour/resource to operate, whilst maintaining acceptable product quality for the patient. In this high frequency production process, residual solvents can be considered for a reduced frequency of testing because they are considered to be low risk and can be reliably controlled procedurally within the drying stage of the process.
Stage 2 – Assessment of attributes
Candidate attributes are assessed to confirm the process is under control for that attribute (i.e., there is little or no evidence of special-cause variability10). This can be achieved by fully testing a sufficient number of batches so that the common-cause variability10 of the process attributes can be estimated under standard operating conditions, using multiple batches of starting material, etc. The number of batches tested should be selected in order to obtain a reasonable estimate but also to increase the chance of identifying any sources of special-cause variability10. The time span required to collect these batches should be sufficient and also include commonly-occurring process variables, for example, all starting material sources.
Separately for each attribute, a statistical assessment of the process is made by considering the following criteria:
- A control chart of the process
Generating a control chart enables assessment of whether there is any special-cause variability present in the process and how the common-cause variability compares with the specification limits. The visual assessment is aided by flagging observations on the chart that fail various statistical process control-based tests10, such as no observation outside the ‘mean ±3 sigma’ region, eight points in a row on same side of centre line, four of five samples in a row outside mean ±1 sigma region and two of three samples in a row outside mean ±2 sigma region.
- An assessment of the process performance index (Ppk) and process capability index (Cpk)
If the Ppk10 and Cpk10 are reasonably similar, (as a pragmatic rule within 20% of each other), then this implies that there is little special-cause variation in the process and that the majority of the variability present is common-cause. Additionally, the process will only be deemed suitable for skip-testing if the lower 95% confidence interval of the Ppk measure is greater than 1.5. This equates to an approximate failure rate of 3.4 in 1 million. A Ppk much greater than 1.5 might allow a greater difference between the Ppk and Cpk to be accepted, dependent on the likely causes of the special-cause variation and whether its influence is sufficiently accounted for in the Ppk estimate (i.e., the distribution can be adequately estimated).
Assuming the process is under control, then
- An assessment of the probability of missing an attribute that fails to meet specification will be generated, where
Probability of not testing an attribute that misses specification | = | Probability of manufacturing a batch with an attribute outside specification range | X | Probability of not testing an attribute that is outside specification |
Note the first probability on the right hand side of the equation is calculated from the control chart (by fitting an appropriate probability distribution, e.g., the Normal distribution, to the data and using the cumulative density function to predict the proportion which falls outside the specification limits) and the second is based on the skip strategy (frequency of the skipping).
Taken together, these three criteria will only be satisfied when the risk of an attribute failing a specification test is small. While it may be possible to justify a skip-test strategy where the risk of failure is higher, it can be argued that this strategy should not be pursued (it would require a very good statistical estimate of the risk, which may not be easy to calculate accurately, given that the prediction involves employing the tails of any probability distribution). Using the strict criteria given in this article implies that predicting the failure rates with great precision is not required since the level of risk associated with attributes that satisfy these criteria is negligibly small.
To collect sufficient information to assess the suitability of the attribute for skip-testing, it is recommended that a minimum of 200 batches should be tested at this stage. Hence, this step will require at least 200 production batches to be manufactured with full analytical testing being performed on each one. This project-specific number corresponds to a sufficient period of time that corresponds to the full lifecycle of the process so that any potential causes of special-cause variability will be encountered.
The control chart of the residual solvents of 280 batches is given in Figure 2 (page 00). The Ppk was calculated as 8.4 (lower 95% CI 7.8) and the Cpk was 15.1. Although the difference between these two results was greater than 20%, the absolute size of them indicates that these statistics are not a cause for concern, and considering them alongside the control chart, it was deemed that the process was capable of controlling residual solvents, i.e., under statistical control. For example, assuming the process is under control, the probability of not testing a batch that does not meet the residual solvents specification when testing one in every 100 batches, using the equation given previously, was estimated to be approximately (1×10-20)*(99/100), which is <0.0001% (this approximates to less than one batch failure every 300 years).
Stage 3 – Routine application of skip-testing
If the conditions described so far are satisfied, then skip-testing can be implemented. Stage 3 involves the routine running of the process, with ongoing verification to confirm there are no batch failures or significant trends observed. To begin with, the frequency of skipping will be selected to ensure that the probability of not testing an attribute which misses specification is small. This frequency should align with the number of batches that can be held, e.g., testing one in every 12 batches (per interval) because 12 batches are manufactured each day. Additionally, the choice of which batches to test and which to skip should be made randomly within each interval, ensuring those tested do not align with other systematic factors, e.g., always testing the batches manufactured on a Monday.
All skipped batches can either be held until the next batch tested is confirmed to have passed or released with acceptance that there is a very small risk that they may need to be recalled. If, after a suitable period of time, the process has remained under control and capable, and the skip strategy has proved successful, the frequency of testing may be reduced further (the level of increased skipping will depend on the process). During this stage, along with testing the batches to confirm the attributes are within specification, a check is also made that the process is still under control and capable (preferably by statistical process control monitoring with periodic review).
It was decided that initially one in 10 batches (which relates to one per day) would be tested. In practice, on each day one of the 10 batches was selected at random for testing prior to manufacture to avoid any unforeseen time-related effects affecting the assessment. If, one year later, the process continues to remain under control and capable, with the skip strategy thus proven to be successful, the level of testing could be reduced further.
Stage 4 – Change control and remediation
If an attribute fails to meet specification during the ongoing verification of tested batches, then skip-testing should be suspended and full specification testing reinstated. The batches that were not tested (between the previous batch tested, where the attribute passed specification, and the latest batch that has failed) also need to be tested. An investigation can be performed to identify why the attribute failed to meet specification. Once identified and preventative action has been taken, the testing strategy can return to Stage 2. The number of batches tested may be reduced from the original total assessed in Stage 2, depending on the cause of the failure, the outcome of the investigation and the knowledge of the process gained since the initial assessment. In the example presented earlier, up to 200 further batches (this number being dependent on the cause of the failure) may be tested and the process re-evaluated.
During the assessment of process changes (both planned and unplanned deviations), it is important that the assessment of the change considers any impact on the skip-testing strategy. In the event of a process deviation or if another routinely tested attribute (which is not part of the skip-testing regime) fails specification, then any impact on skipped batches will be assessed and full testing of all skipped batches should be considered. This change control process may also be followed if there is a potentially impactful change to the process.
Conclusion
The authors have described a four-stage approach where skip-testing can be introduced for attributes deemed to be at low risk of failing specification, based on considerable manufacturing experience. A rigorous risk-based approach, supported by a statistical evaluation to investigate the suitability of attributes as candidates for skip-testing, is recommended. Once implemented, ongoing verification of the process should be performed to ensure it is under control. The skip frequency should be reviewed if significant changes to the process are made. Ideally, skipped batches should not be released for commercial use until the next batch is analysed. In the event of a batch failure, full testing of all affected skipped batches should take place and the skip-testing strategy must be reviewed.
References
- International Conference on Harmonisation; “Specifications: Test procedures and acceptance criteria for new drug substances and new products: Chemical substances. Q6A”, 1999
- International Conference on Harmonisation; “Assessment and Control of DNA Reactive (Mutagenic) Impurities in Pharmaceuticals to Limit Potential Carcinogenic Risk. M7”, 2014
- Carlin B, Carter D et al. Joint Position Paper on Pharmaceutical Excipient Testing and Control Strategies. Pharmaceutical Technology, 31(9), 2007, pp 84-104
- Ciurczak E. Putting Q6A to the Test: Upon Dissection, Guidance Needs an Update. Pharmaceutical Manufacturing, Feb 11, 2009
- Swartz ME and Krull IS. Handbook of Analytical Validation. CRC Press, Taylor & Francis Group, 2012
- Borman P, Popkin M, Oxby N, Chatfield M, Elder D. Analytical methods and control strategies: The forgotten interface? Pharmaceutical Outsourcing, 16(1) 2015
- International Conference on Harmonisation; “Pharmaceutical Development. Q8(R2)”, 2009.
- Torbeck L. Statistical Solutions: Square Root of (N) + 1 Sampling Plan. Pharmaceutical Technology, 33(10) 2009, 128
- Saranadasa H. The Square Root of N Plus One Sampling Rule. Pharmaceutical Technology, 27(5), 2003, 50-62.
- Wheeler DJ and Chambers DS. Understanding statistical process control. Vol. 37. Knoxville, TN: SPC press, 1992
Biographies
Phil Borman is a Chartered Chemist with more than 19 years of experience in the pharmaceutical industry, having obtained a Masters in Chemistry from UMIST (Manchester) University and a Masters in Industrial Data Modelling from De Montfort (Leicester) University. Phil is currently the Informatics and Data Lead within Platform, Technology and Science at GlaxoSmithKline (GSK). Previous to his current role, Phil pioneered the adaptation of QbD principles to Analytical methods as well as leading the design and implementation of novel chromatographic platforms which are now commonplace across GSK. Phil has published widely in the field of Analytical Chemistry and Applied Statistics.
Simon Bate studied in London, gaining a PhD in cross-over design theory before joining GlaxoSmithKline in 2000. He has supported biologists and chemists, helping them gain a better understanding of their experimental designs and statistical analysis through consultancy, project-related support and the delivery of training courses. He has worked with external groups including the NC3Rs (National Centre for the Replacement, Refinement and Reduction of Animals in Research) and is co-author of a text book ‘The Design and Statistical Analysis of Animal Experiments’.
Keith Freebairn studied Applied Chemistry at Newcastle upon Tyne polytechnic and has a PhD in Physical Chemistry from the University of Edinburgh. Keith joined GlaxoSmithKline in 1988 and, over the past 28 years, has worked as an Analytical Chemist supporting chemical process development activities. He has particular interests in separation science, analytical instrument automation and process analytical technology.
Issue
Related topics
Drug Manufacturing, ICH guidelines, Process Analytical Technologies (PAT)