Machine learning model enables adventitious agent detection in cell cultures
Posted: 20 June 2022 | Hannah Balfour (European Pharmaceutical Review) | No comments yet
Researchers have developed a rapid, label-free process analytical technology, called the anomaly detection model, to monitor microbial contamination in cell cultures in near-real-time.
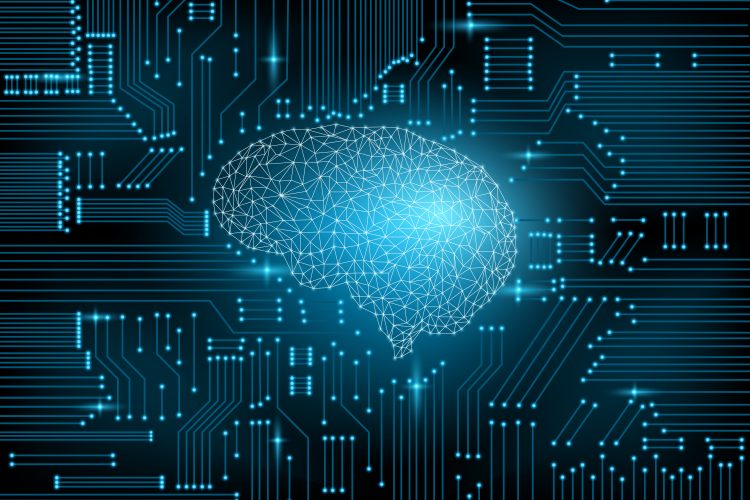
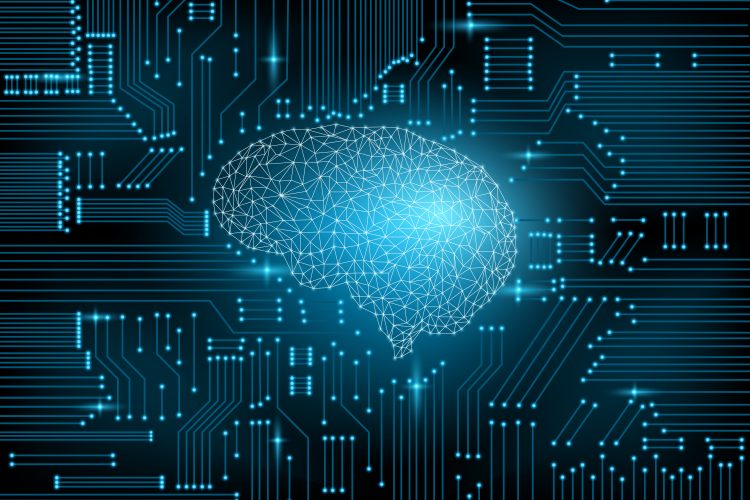
Leveraging machine learning (ML), Singaporean researchers have developed a new process analytical technology (PAT) for detecting adventitious microbial contamination in mesenchymal stromal cell (MSC) cultures, ensuring the rapid and accurate testing of cell therapy products intended for use in patients.
The anomaly detection model uses ML to predict if a culture is contaminated or not in a near-real-time-like manner. According to its developers, the “breakthrough method” could be used during the cell manufacturing process to overcome compared some of the inefficiencies of end-point testing.
Rising numbers of cell therapies are being developed or approved for a range of applications, with promise shown in treating cancers, autoimmune diseases, spinal cord injuries and neurological conditions, among other indications. As their utility expands, the manufacturing methods and processes are continually being refined to ensure the safety, efficiency and sterility of these products for patient use.
The anomaly detection model, a rapid, label-free PAT, was developed by the Critical Analytics For Manufacturing Personalized-Medicine (CAMP) Interdisciplinary Research Group (IRG) at Singapore-MIT Alliance for Research and Technology (SMART), MIT’s research enterprise in Singapore.
The ML model was developed by collecting sterile cell culture media samples from a range of MSC cultures of different culture conditions. Some of the collected samples were spiked with different bacteria strains at different colony-forming units (CFUs). Using ultraviolet-visible (UV-Vis) spectrometry the team obtained the absorbance spectra of the sterile, unspiked and bacteria-spiked samples, which were then used to train the model. Testing of the ML model with a mixture of sterile and bacteria-spiked samples demonstrated the model’s performance in accurately predicting sterility.
“The practical application of this discovery is vast: when combined with at-line technologies, the model can be used to continuously monitor cultures grown in bioreactors at good manufacturing practice (GMP) facilities in-process. Consequently, GMP facilities can conduct sterility tests for bacteria in spent culture media more quickly with less manpower under closed-loop operations. Lastly, patients receiving cell therapy as part of their treatment can be assured that products have been thoroughly evaluated for safety and sterility,” commented Shruthi Pandi Chelvam, lead author and Research Engineer at SMART CAMP who worked with Derrick Yong and Stacy Springs, SMART CAMP Principal Investigators, on the development of this method.
According to the developers, their anomaly detection model can be used to detect the presence of adventitious microbial contamination in cell cultures within a few minutes, overcoming one of the key challenges with cell therapy manufacture – the long (multiple day) incubation periods required for sterility testing. Other benefits of the in-process method include saving resources, as traditional methods usually test finished products and if contamination is detected, cultures must immediately be discarded and reconstructed.
“Our increased adoption of machine learning in microbial anomaly detection has enabled us to develop a unique test which quickly performs in-process contamination monitoring, marking a huge step forward in further streamlining the cell therapy manufacturing process. Besides ensuring the safety and sterility of cell products prior to infusion in patients, this method also offers cost and resource effectiveness for manufacturers, as it allows for decisive batch restarting and stoppage should the culture be contaminated,” added Yie Hou Lee, Scientific Director of SMART CAMP.
Moving forward, CAMP aims to develop an in-process monitoring pipeline in which this anomaly detection model can be integrated with some of the in-house at-line technologies that are being developed, which would allow for periodic culture analysis using a bioreactor.
The anomaly detection model was explained in an oral abstract (abstract 3), published recently in Cytotherapy.
Related topics
Analytical techniques, Artificial Intelligence, Biologics, Bioproduction, Drug Manufacturing, Drug Safety, Microbial Detection, Microbiology, Process Analytical Technologies (PAT), QA/QC, Spectroscopy, Technology, Therapeutics