Advancing autonomous API reaction optimisation
Posted: 5 September 2022 | Hannah Balfour (European Pharmaceutical Review) | No comments yet
A novel flow reactor platform was able to self-optimise a range of variables within a multi-step reaction by leveraging process analytical technologies (PAT) and chemometric models.
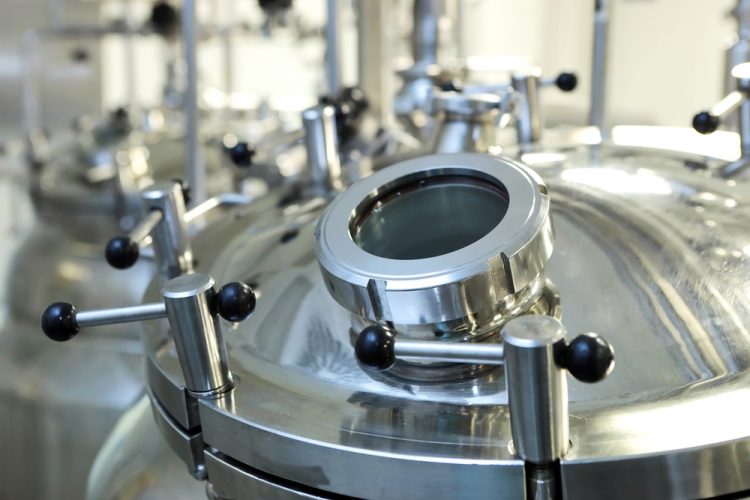
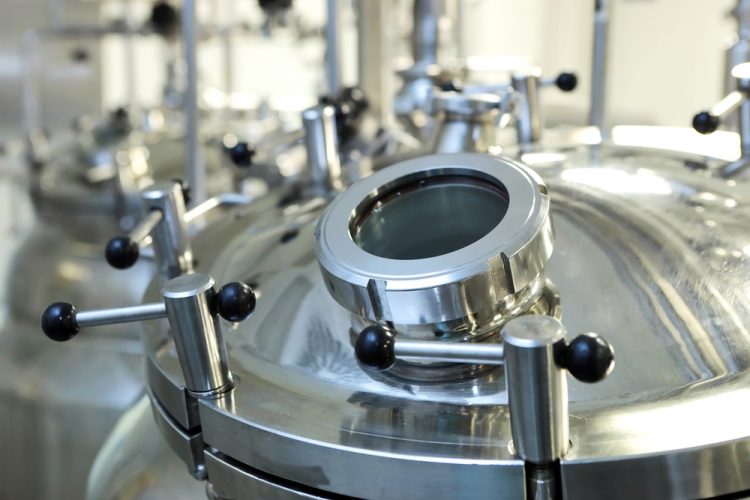
Researchers have developed a self-optimising flow reactor platform for complex reactions. Based on rapid nuclear magnetic resonance (NMR) and Fourier-transform infrared spectroscopy (FTIR) spectroscopic measurements and processed using chemometric models, the platform was able to provide optimal reaction parameters with minimal background knowledge.
Though autonomous flow reactors are becoming more widely used for pharmaceutical synthesis, the complexity of chemical reactions and limitations of analytical methods remain a challenge. Continuous flow processing provides an opportunity for automated (closed-loop) experimentation, but though many self-optimising flow reactor systems has been disclosed, they typically focus on a single reaction step, with a single analysis point and only optimise up to four variables.
In a study published in Advanced Science, Sagmeister et al. sought to expand the applicability and utility of autonomous optimisation in flow reactors by increasing the number of optimisable variables. The researchers report the use of multi-objective optimisation algorithms to create a modular reaction platform that can integrate multiple PAT tools with chemometric processing.
Sagmeister et al. leveraged two near real-time spectroscopic techniques, NMR and FTIR, to facilitate measurements to be taken on- or in-line. Combining such PAT measurements with chemometric modelling enabled the quantification of component concentrations, even in cases of complex mixtures with overlapping components.
The team first tested the platform with a four-variable single-step reaction, called nucleophilic aromatic substitution, to determine the most effective optimisation methodology. They found that beginning self-optimisation with minimal background knowledge – just a single experiment in the centre of the design space – provided the optimal reaction parameters within the shortest operational time (14 iterations).
To show the utility of this approach, they then carried out the multi-objective self-optimisation of the synthesis of the amyloid lateral sclerosis drug edaravone. This was a two-step process involving imine formation and cyclisation, with seven optimisable variables and three competing optimisation objectives (namely: maximising solution yield and space-time yield, while minimising reagents).
Despite the complexity of the latter experiment, the platform achieved “excellent results”, following 85 iterations run over an approximately 26-hour period. A >95 percent solution yield of the intermediate and up to 5.42 kgl−1h−1 space-time yield of the active pharmaceutical ingredient (API) edaravone, whilst minimising reagent excesses.
The researchers concluded that the use of multi-objective optimisation algorithms leveraging multiple measurement points increase the coverage and applicability of experimental results, and that their findings represent “a marked advance in the complexity of autonomous flow reactors in both the quantity of obtained data and the size of the optimisable design space”.