What do we need from PAT?
Posted: 18 December 2017 | Chris Edlin, Darren Whitaker | No comments yet
Pharmaceutical manufacturing in the modern era is facing unprecedented demands, including in-depth scrutiny of production methodology, and inefficiencies in current practices with respect to waste, energy usage and time management. Coupling this with increased complexity in the manufacture of newer products and the decline of blockbuster drugs, results in an industry striving to drive down overhead costs and increase plant efficiency and capacity on a daily basis.
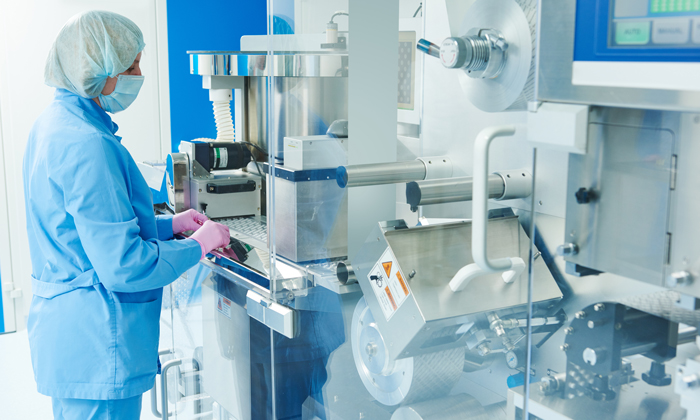
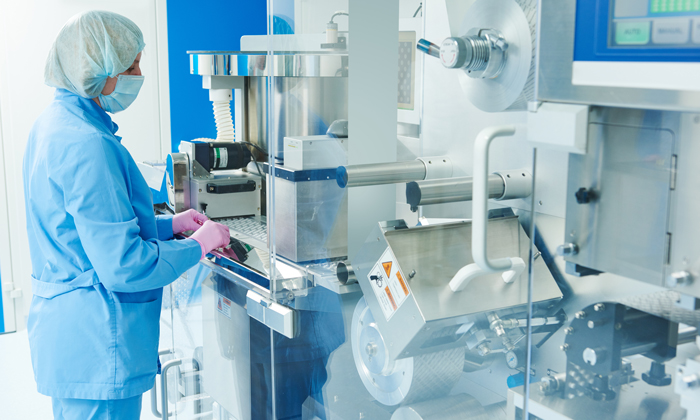
THE use of process analytical technology (PAT) is nothing new. In 2004 the US Food and Drug Administration (FDA) outlined the use of PAT, using the original definition: “PAT (is) a system for designing, analysing, and controlling manufacturing through timely measurements (ie, during processing) of critical quality and performance attributes of raw and in-process materials and processes, with the goal of ensuring final product quality”.1 This definition allows a broad scope to be taken when defining measurement/sensing technology and analysis techniques. Additionally, three process domains can be identified within the FDA’s vision: process development (design), process monitoring (analysing), and continuous optimisation/control (controlling). Since its introduction, PAT has proven useful for many process unit operations within both primary (API) and secondary (final dosage form) manufacturing.2,3
Shifting manufacturing landscape
Several drivers changing the context of the pharmaceutical industry significantly impact the manufacturing section of the value chain. According to Stegemann,4 the major drivers in the scientific domain are the advent of systems biology and medicine, plus advances in genetics leading to personalised therapies. The rise of personalised medicines has also precipitated the advent of new drug platforms including biologics, antibody-drug conjugates (ADCs) and gene therapy. Additionally, society is more involved with healthcare decisions driven by access to information and augmented by real-time collection of biometric data, and shifting demographics are creating patient populations characterised by older age, multi-morbidity, polypharmacy and frailty.
The net result of these context-shifting drivers is that manufacturing organisations need to adapt and respond by developing and implementing new production techniques. In the medium- to long-term the advent of personalised medicine will necessitate low volume, high potency continuous manufacturing using novel platforms to deliver non-traditional therapeutic molecules, which will require exquisite process control and decontamination procedures. The current trend towards continuous manufacturing while striving to increase quality, reduce production footprints and costs, needs to be supported by appropriate enabling PAT and appropriate control models.
Is PAT keeping up?
Examples of PAT application within an industrial setting tend to follow a similar path:
1. Use quality by design (QbD) protocols to define what key quality attribute (KQA) needs to be measured
2. Decide how to use the data (classification or quantification)
3. Apply some statistical (chemometric) transformations (principal component analysis (PCA), linear discriminate analysis (LDA), partial least squares (PLS), etc)
4. Perform calibration experiments
5. Finally, deploy model to be tested in production.
While this has proven effective for batch manufacturing, continuous campaigns will not necessarily yield results in the same way and there is an expectation that PAT will evolve as the industry makes a move towards Industry 4.0.
While the ‘questions’ being asked of PAT remain similar, the control aspects will differ substantially. Considering a blending operation as an example: within the batch environment a spectroscopic technique can be used to detect the batch endpoint based on the defined spectral signature of blend uniformity. Once this is detected, the control system can be instructed to stop the process. However, in the continuous environment there is no endpoint. The signature of uniformity needs to be measured continuously and fed back into a control system or to the operator to display that the process is at a steady-state and maintaining uniformity. Any deviations need to be detected with input parameters varied to return the process to steady-state. This need for diagnosis of deviations is quite new and will inevitably require an adjustment of the traditional approach.
In order to successfully navigate this landscape, data from PAT analysers will need to be combined with other parametric data (temperature, pressure, flow rate, heating rate, heating medium temperatures, etc) and also (more) likely, with measurements from cheaper and more simple sensors (conductivity, refractive index, etc). Luckily, with the big data movement gaining traction, R&D and quality control teams within pharmaceutical companies are gathering more and more data at every step of the process using automated Internet of Things (IoT) sensors on the factory floor.5
With the advent of cloud computing and storage, there is no reason for this data to go unused. In previous years the cost of high-performance computing, server hardware and overheads associated with computing technology were major roadblocks in the utilisation of large amounts of process data.6 Now, services such as Amazon web services7 and Google’s computing cloud8 allow data to be stored, retrieved and utilised faster and more efficiently than ever before. Additionally, for those who prefer to use local hardware, server and HPC infrastructure built on Raspberry Pi computers and the like are becoming commercially available.9 The power of this technology coupled with interconnected and automated systems means companies can now analyse massive amounts of process data (Figure 2). Tapping into this big data will allow companies to build end-to-end process controls, resulting in higher-quality products, more predictability, more efficient manufacturing, and faster time to market.
What do we need from PAT?
While this seems to suggest a move away from spectroscopic PAT analysers, this is not the case; by referring back to the PAT process domains outlined earlier, a new process can be defined. Traditional PAT (particularly molecular spectroscopy) will be vital in gaining chemical understanding, but this may be limited to a process development step. From here, machine learning and pattern recognition algorithms can be used to correlate subtle changes in parametric data to chemical events. This type of analysis is made possible by simultaneous measurement and storage of multiple parameters at increasingly small time intervals – the key enabling technologies of this type of analysis were described earlier.
This will allow control strategies to be based on simple measurements and remove the need for multiple, expensive, complex technologies to be deployed on all production lines (Figure 3). Looking back to the FDA definition of PAT, these simple measurements can still be considered as PAT measurements. Several member companies of the Pharmaceutical Manufacturing Technology Centre (an industry-focused applied research centre at the University of Limerick) have outlined this as a particular need. Evidence suggests that industries are looking to move away from installing expensive PAT in favour of using simple sensor platforms and linking the measurements with quality data via advanced chemometrics and mathematics.
Hardware is only one aspect of the PAT problem and traditionally chemometrics (a branch of statistics dealing with chemical data) was not a requirement for a PAT implementation. However, it is unlikely that the new paradigm described here will be successful without advanced chemometric and mathematical techniques being at the forefront of development. It is widely accepted that, to ‘achieve process understanding’ one must develop a mechanistic understanding of process parameters on product attributes. In an era of big data this will likely include the extension of chemometrics into the data mining domain and require the additional input of ever more complex machine learning (neural networks, support vector machines, etc).
Automated self-optimisation
An additional emerging benefit of continuous processes is the ability to use PAT measurements to carry out automated DoE type experiments. This will allow investigation of a large process parameter design space with minimal operator input using a small amount of starting material. Along with DoE type campaigns, advanced self-optimising algorithms such as a SIMPLEX10 approach and the SNOBFIT11 algorithm are effective at locating an optimum set of process parameters for continuous fl ow processes.
Conclusion
Academia and industry have a wealth of experience in deploying PAT in traditional pharmaceutical processing, and analytical instrumentation and control system integration have commercial solutions from a number of sources. The future will need to focus on redefining PAT sensors and linking big data and process understanding generated in development phases to final product quality attribute, as well as determining what that value-added quality metric will be to the customer.
Biography
References
1. US Food and Drug Administration. Guidance for industry: PAT – A framework for innovative pharmaceutical development, manufacturing, and quality assurance. DHHS, Rockville, MD (2004).
2. Laske S, et al. A Review of PAT Strategies in Secondary Solid Oral Dosage Manufacturing of Small Molecules. J Pharm Sci. 2017;106:667–712.
3. Ley SV, Fitzpatrick DE, Ingham R, Myers RM. Organic synthesis: march of the machines. Angew Chem Int Ed. 2015;54:3449-3464.
4. Stegemann, S. The future of pharmaceutical manufacturing in the context of the scientifi c, social, technological and economic evolution. Eur J Pharm Sci. 2016;90:8-13.
5. Reis M, Gins G. Industrial Process Monitoring in the Big Data/Industry 4.0 Era: from Detection, to Diagnosis, to Prognosis. Processes. 2017;5:35.
6. Goldin E, Feldman D, Georgoulas G, Castano M, Nikolakopoulos G. Cloud Computing for Big Data Analytics in the Process Control Industry. 2017;1373-1378. doi:10.1109/ MED.2017.7984310
7. Amazon Web Services. https://aws.amazon.com
8. Google Computing Cloud. https://cloud.google.com
9. Bitscope Blade. www.bitscope.com/product/blade
10. Fitzpatrick DE, Battilocchio C, Ley SV. A novel internet-based reaction monitoring, control and autonomous self-optimization platform for chemical synthesis. Org Process Res Dev. 2015;20:386–394.
11. Holmes N, et al. Self-optimisation of the fi nal stage in the synthesis of EGFR kinase inhibitor AZD9291 using an automated fl ow reactor. React Chem Eng. 2016;1:366-371.