Could process data offer a different approach for predicting quality?
Posted: 7 June 2022 | Hannah Balfour (European Pharmaceutical Review) | No comments yet
A data collection study provides a product quality dataset that could form the basis of advanced analytical models to predict final product quality.
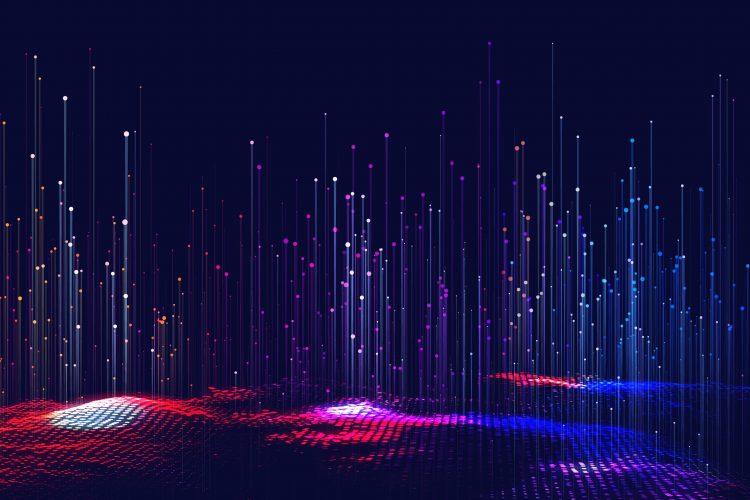
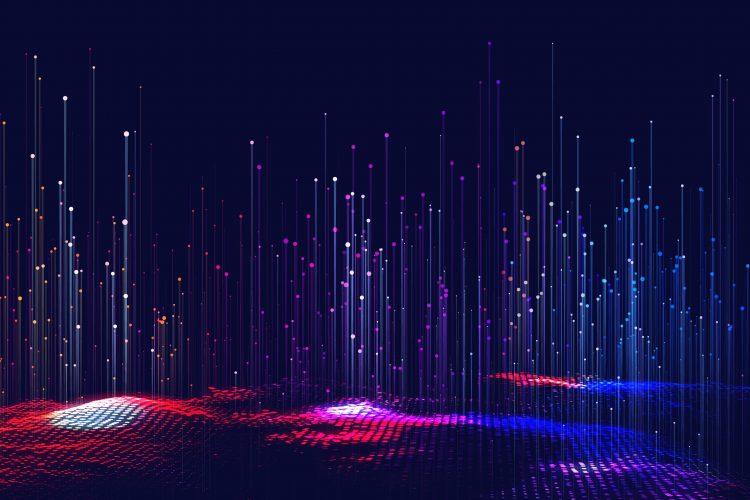
As digitalisation increases in pharmaceutical manufacturing, researchers are examining whether the data acquired from sensor-equipped manufacturing processes could be used to develop advanced analysis models and procedures to replace conventional laboratory-based quality testing.
In a paper published in Scientific Data, researchers collected data from 1,005 real-life production batches of a blood cholesterol lowering pharmaceutical product produced in high volume. Included in the dataset were parameters known to have a significant impact on final product quality, namely the quality of incoming materials and compression process data.
Analysing pharmaceutical product quality is mandatory for release to market; however, according to the authors, the current processes are “predominantly laboratory based and thus very time consuming”. As regulators promote the implementation of technology to enhance product quality and process understanding, the wealth of information available for each product batch is growing. In their paper, researchers report on the collection of Big Data for a specified pharmaceutical product and present parameters that could be used to produce models to predict final product quality.
The product, a film-coated tablet with an immediate release drug profile, has a simple formulation – consisting of excipients and an active pharmaceutical ingredient (API) – and a straightforward manufacturing process.
For each batch the dataset included raw material quality results, compression process time series data and final product quality (impurities, residual solvents and drug release) results for the selected product. The data provided an insight into “every 10 seconds of the process trajectory for each batch along with product quality collected over several years”.
Within the dataset were different sub-families, four products differing in strength and nine in manufacturing batch size. Each sub-family is defined by a product code in the data. To enable analysis despite the variation between sub-families, certain parameters were included in the dataset: weight relative standard deviation (RSD) to account for the four different target core weights; and tensile strength to account for different target thickness, diameter and hardness of tablets.
According to the researchers, the collected data “offers an opportunity to develop advanced analysis models and procedures which would lead to the omission of current conventional and time-consuming laboratory testing”. This, they wrote, offers obvious benefits to industry, namely “reducing product lead times and costs of manufacture”.
The authors noted that, if the parameters extracted from the time series datasets do not provide reliable prediction models, then applying deep learning methodologies to the whole time series dataset may help identify attributes that better predict final product quality.
Related topics
Big Data, Drug Manufacturing, Drug Safety, Formulation, Informatics, Models, Process Analytical Technologies (PAT), QA/QC, Therapeutics