Using machine learning to interpret material spectra
Posted: 7 September 2018 | European Pharmaceutical Review | 1 comment
Researchers from the University of Tokyo have developed a method to use machine learning to interpret data from spectroscopy techniques…
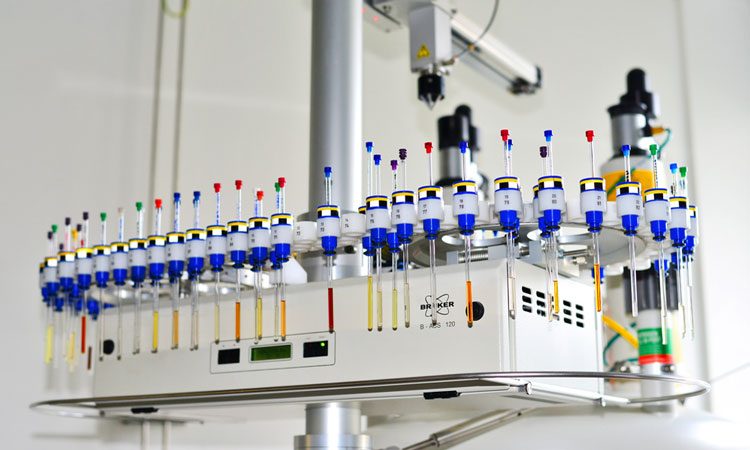
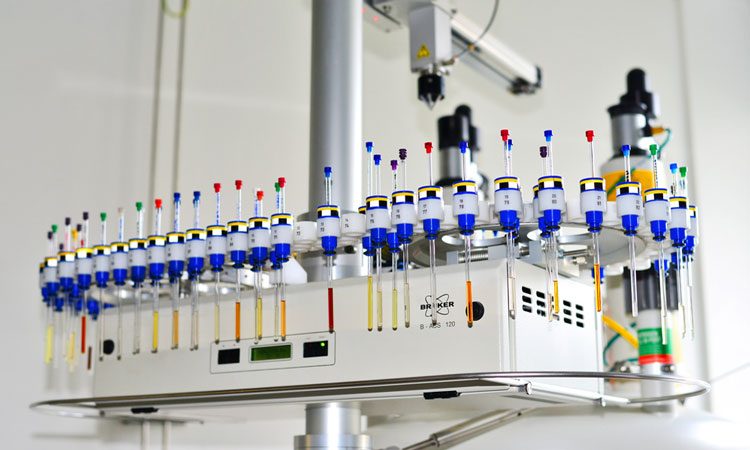
A team of researchers from a university in Tokyo have constructed a spectral database from theoretical calculations, where each spectrum had an individual correspondence with its atomic structure.
Spectroscopy techniques are commonly used in materials research as they enable the identification of materials from their varying features, which are connected to specific material properties. Modern methods have enabled rapid generation of huge numbers of material spectra, however it is necessary to interpret these spectra to gather relevant information about the material.
The interpretation of a spectrum is not always a simple task and requires considerable expertise. Each spectrum is compared with a database containing numerous reference material properties, but unknown material features that are not present in the database can be problematic, and often have to be interpreted using spectral simulations and theoretical calculations. In addition, the fact that modern spectroscopy instruments can generate tens of thousands of spectra from a single experiment is placing considerable strain on conventional human-driven interpretation methods, and a more data-driven approach is thus required.
Using big data analysis techniques has attracted attention in materials science applications, and researchers at The University of Tokyo Institute of Industrial Science realized that such techniques could be used to interpret much larger numbers of spectra than traditional approaches. “We developed a data-driven approach based on machine learning techniques using a combination of the layer clustering and decision tree methods,” states co-corresponding author, Associate Professor Teruyasu Mizoguchi.
Use of the two machine learning methods allowed the development of both a spectral interpretation method and a spectral prediction method, which is used when a material’s atomic configuration is known.
The method was successfully applied to interpretation of complex spectra from two core-electron loss spectroscopy methods, energy-loss near-edge structure (ELNES) and X-ray absorption near-edge structure (XANES), and was also used to predict the spectral features when material information was provided. “Our approach has the potential to provide information about a material that cannot be determined manually and can predict a spectrum from the material’s geometric information alone,” says lead author Dr Shin Kiyohara.
However, the proposed machine learning method is not restricted to ELNES/XANES spectra and can be used to analyze any spectral data quickly and accurately without the need for specialist expertise. As a result, the method is expected to have wide applicability in fields as diverse as semiconductor design, battery development, and catalyst analysis.
Related topics
Analytical techniques, Research & Development (R&D), Spectroscopy
Nice update.